AI-powered Trademark Search and Review: Streamline Your Brand Protection Process with Confidence and Speed (Get started for free)
7 AI-Related Red Flags When Evaluating a Technical Co-Founder for Your AI Startup A Patent and IP Perspective
7 AI-Related Red Flags When Evaluating a Technical Co-Founder for Your AI Startup A Patent and IP Perspective - Unproven Track Record in MLOps and Model Deployment at Scale
When evaluating a technical co-founder for your AI startup, a lack of demonstrable experience in MLOps and deploying models at scale should be a significant red flag. Successfully deploying AI models in a real-world setting requires more than just building a good model. It demands a robust operational framework. This is where MLOps becomes essential. Without a proven ability to manage the entire lifecycle of AI models, including development, deployment, monitoring, and updates, your startup could face numerous challenges.
A co-founder's ability to streamline workflows, meticulously document processes, and create robust deployment strategies is crucial for successfully integrating AI into your core business operations. MLOps isn't a mere afterthought; it's the backbone of efficient and scalable AI implementation. If a potential co-founder hasn't navigated these complexities before, their capacity to lead your startup's AI efforts effectively might be questionable. In the competitive landscape of AI startups, a lack of solid MLOps experience can seriously impede the company's growth and limit its ability to fully harness the potential of its AI solutions.
When evaluating a potential co-founder for an AI startup, it's crucial to assess their practical experience in operationalizing AI models. Simply having theoretical knowledge of machine learning isn't enough; it's the ability to navigate the complexities of MLOps and deploy models effectively at scale that truly matters. Have they truly wrestled with the challenges of managing and deploying models in a real-world setting? Have they dealt with versioning, model drift, and the operational demands of keeping things running smoothly at scale? If the candidate doesn't have a proven track record in these areas, it can signal a potential bottleneck for your startup. Building and scaling AI systems is far more than just coding a model. You need a system for managing and monitoring models across their lifecycle, ensuring performance doesn't degrade over time. This is especially critical in dynamic business environments where data patterns can shift quickly. Without a firm grasp of MLOps principles and experience in scaling deployments, the risk of delays, performance issues, and wasted resources significantly increases. Essentially, a solid understanding of how to seamlessly integrate AI models into the core business operations and scale them to meet growing demands is absolutely vital. If a co-founder hasn't had the opportunity to grapple with these challenges firsthand, their ability to lead your company in successfully deploying and managing its core AI technology may be questionable.
7 AI-Related Red Flags When Evaluating a Technical Co-Founder for Your AI Startup A Patent and IP Perspective - Missing Patent Applications for Core AI Technology Before Key Deadlines
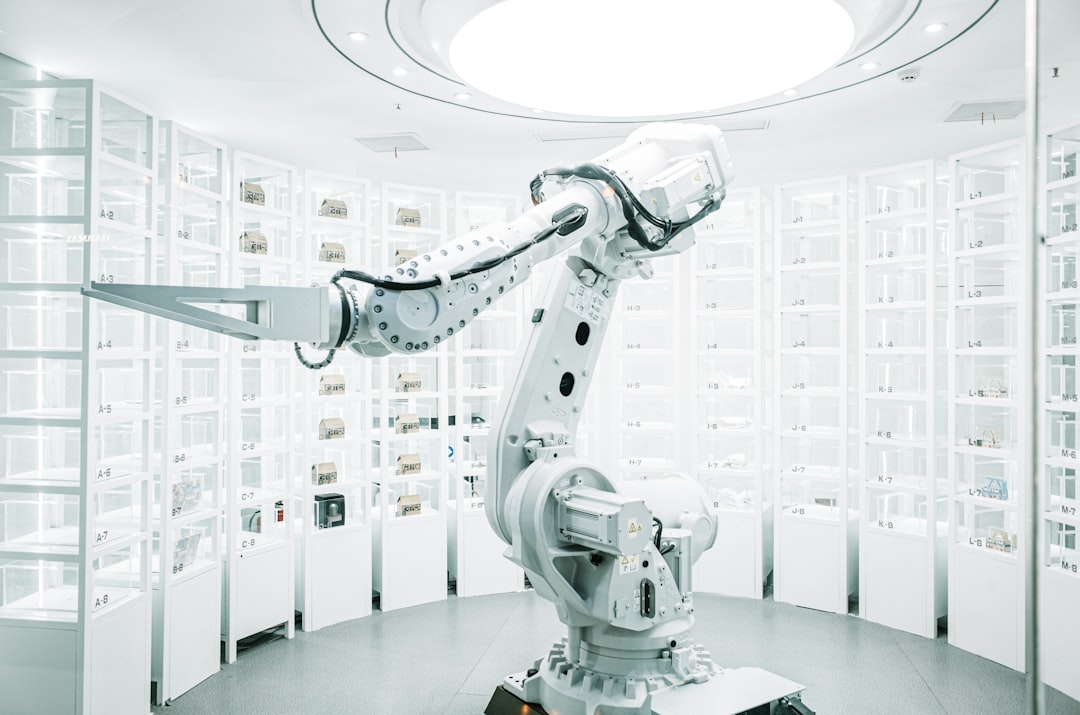
In today's rapidly evolving AI landscape, securing intellectual property through patents is more critical than ever, especially for core AI technologies. However, a common misstep for some AI startups is failing to file patent applications before key deadlines. This oversight can have serious consequences for protecting their innovative work. The recent surge in AI-related patent filings, especially since 2021, underscores the importance of a co-founder's awareness of patent timelines and processes.
When evaluating a potential technical co-founder, a lack of understanding of patent applications and the broader intellectual property landscape should be considered a warning sign. It could indicate a limited grasp of how patents protect innovation and contribute to a company's strategic advantage. In the fiercely competitive world of AI startups, a failure to secure timely patent protection for core AI technology can easily hinder the company's growth and future success. Therefore, it's vital to assess a potential co-founder's expertise in this critical area to ensure they can effectively guide the startup's IP strategy. Ignoring patent deadlines and related aspects of intellectual property can be a serious hindrance in the long run, and it's an area that deserves careful scrutiny during the co-founder selection process.
The landscape of AI patent applications has exploded in recent years, reflecting the surge in investment and development across the field. The US Patent and Trademark Office (USPTO) has seen a dramatic increase in AI-related filings, especially since 2021, with search trends for AI patenting skyrocketing. This growing interest has even prompted the USPTO to provide updated guidance for patent examiners, recognizing the unique challenges posed by evaluating AI inventions.
It's interesting how companies like IBM, Google, and Microsoft are leading the charge in AI patents, solidifying the US's role as a pioneer in this domain. However, the global trend is evident; the Japan Patent Office has also noted a similar increase in AI-related applications, suggesting a worldwide push towards innovation in this sector.
While many stakeholders are contributing feedback to the USPTO on AI patent eligibility, there's also an active debate about the patentability of inventions that rely on AI assistance. Further complicating things, AI patents often involve numerous intertwined technologies, making the classification process intricate and highlighting the complex nature of AI development.
The sheer volume of patent filings, particularly in the US, can create bottlenecks. A startup might face substantial delays in getting their core AI technology patented, which could lead to missed market windows and hinder their growth. Then there's the risk of competitors swooping in to replicate innovative ideas if proper patent protection isn't in place. This risk can be particularly detrimental in a fast-paced industry where gaining a foothold quickly is vital for success.
Moreover, having strong intellectual property can be a game-changer when it comes to attracting investors. VCs often look for patents as an indication of a company's potential for future success and ability to protect its innovations. Similarly, startups lacking adequate patent protection could struggle to form partnerships, as other companies may be hesitant to collaborate if the startup's technology isn't properly secured.
Furthermore, navigating the complexities of international patent law is crucial. Different countries have varying legal frameworks related to patents, and any oversight in this area could mean a startup's valuable AI technology isn't protected as well as it could be in key international markets.
Another potential stumbling block for startups is the public disclosure of information prior to patent filing. Depending on where you're filing, premature disclosure might jeopardize your chances of receiving a patent at all. This makes navigating the disclosure landscape particularly tricky for companies in this space who need to balance publicity with protecting their innovations.
The increasing complexity of the patent process can also be seen in the growing phenomenon of "patent thickets". It's becoming more challenging to innovate without potentially infringing upon other patents, which can be difficult to anticipate.
Beyond these challenges, ensuring the intellectual property rights are securely assigned within a team through well-drafted employee agreements is crucial. It's easy to lose out on important technology when team members move on to other opportunities if those agreements aren't airtight.
Lastly, the patent application process itself can be a significant time commitment. It can take several years for applications to be finalized, meaning any delays could put a startup at a disadvantage against competitors who have already brought similar innovations to market. Given the speed of advancements in AI, missing these deadlines can become an almost insurmountable obstacle.
In essence, when evaluating technical co-founders for an AI startup, a keen eye for patent-related matters is essential. Understanding these nuances can help a startup avoid potentially costly and time-consuming hurdles, ultimately maximizing its chances of success in the competitive world of AI.
7 AI-Related Red Flags When Evaluating a Technical Co-Founder for Your AI Startup A Patent and IP Perspective - Gaps in Data Security and Privacy Protocol Implementation
When evaluating AI startups, a critical area to assess is the technical co-founder's understanding and implementation of data security and privacy protocols. AI systems often leverage vast amounts of sensitive data, making robust security paramount. However, the rapid advancement of AI technologies has outpaced the development of comprehensive legal frameworks for data protection. This creates a gap where existing laws may not offer sufficient safeguards for individuals and organizations alike.
Failure to implement strong security practices exposes startups and users to considerable risk. A lack of proactive measures, such as implementing zero trust architectures and robust monitoring systems, could lead to data breaches, misuse of sensitive information, and severe reputational damage. Moreover, neglecting these crucial elements can create compliance issues with current and future regulations.
The potential consequences of inadequate data security and privacy are severe. Not only can breaches and misuse damage a startup's reputation and financial stability, but they can also lead to significant legal and regulatory penalties. Given the sensitivity of data used in many AI applications, a co-founder's demonstrable experience in creating secure systems and maintaining compliance with evolving data privacy regulations becomes a crucial factor to consider during evaluation. In the competitive AI landscape, startups that fail to prioritize data security and privacy risk falling behind, potentially losing user trust and hindering their ability to succeed.
When building AI systems, ensuring data security and user privacy is paramount, yet many startups stumble in implementing robust protocols. A significant issue is the prevalence of insider threats, with a concerning portion of security breaches stemming from employees, either intentionally or due to negligence. This underscores a lack of attention to security awareness training and a need for a stronger internal security culture.
Additionally, unpatched vulnerabilities continue to plague many systems. The rate of successful exploits targeting known vulnerabilities is alarming, and this seems to be even more problematic in fast-changing AI environments where keeping up-to-date can be challenging. This signifies the need for better software management and patching practices.
A common shortcoming is inconsistent data governance. Startups often lack a unified framework for managing data security across departments, leading to inconsistent application of rules and increased vulnerability. This highlights a need for centralized data management and clearly defined security roles.
Furthermore, a startling number of individuals within a company are unaware of their organization's data security protocols. Insufficient training on security protocols can expose even the most technically advanced systems to human errors. Improving security awareness across the entire workforce is critical for a strong security posture.
Another frequent oversight is the failure to encrypt sensitive data. Without proper encryption, sensitive information is easily accessible in case of a breach. This highlights a significant lack of emphasis on encryption for both data in transit and data at rest.
Misconfigurations in cloud services are also common. Many startups are rapidly embracing cloud solutions without fully appreciating the potential pitfalls, such as misconfigured permissions or improperly set access controls. It underscores a need for greater competence in cloud security configuration and ongoing monitoring.
The reliance on third-party vendors also creates vulnerabilities. Startups must pay more attention to vendor security practices. If vendors have lax security standards, this can undermine the entire security posture of the startup, exposing sensitive information and creating a clear gap in protection.
Security also suffers when startups neglect basic security best practices. For instance, a considerable number fail to implement multi-factor authentication (MFA), leaving user accounts vulnerable to compromised credentials. This signifies a failure to use readily available and strong security practices.
A lack of comprehensive data lifecycle management is another issue. Startups often hold onto data far longer than needed, creating more potential attack surfaces. Clear rules about data retention and deletion are critical.
Finally, many startups are not keeping up with changing data protection regulations. Failure to adhere to regulations like GDPR and CCPA creates potential for legal action and negative publicity. This signals a need for constant monitoring of regulations and updates to company policies to maintain compliance.
These examples underscore a broader issue: Many AI startups overlook crucial areas in implementing comprehensive data security and privacy protocols. This is a growing concern, as the volume of sensitive data used in AI systems only grows. The challenges of creating and maintaining security in such complex systems remain significant. Ongoing research and efforts toward best practices in these areas are essential for advancing data privacy and security in AI.
7 AI-Related Red Flags When Evaluating a Technical Co-Founder for Your AI Startup A Patent and IP Perspective - No Clear Understanding of AI Model Documentation Requirements
When assessing a potential technical co-founder for your AI startup, a lack of understanding regarding the documentation needs for AI models should raise serious concerns. AI model documentation plays a crucial role in establishing accountability, transparency, and the ability to evaluate the AI system's performance. However, a surprising number of individuals involved in AI development fail to fully appreciate the importance of this aspect. Without thorough documentation detailing the AI model's development and performance evaluation, the startup faces significant risks. Keeping track of iterations, adhering to regulations, and building trust among internal and external stakeholders becomes difficult without comprehensive documentation. A failure to address these documentation requirements can result in operational setbacks, ethical issues, and legal complications that may significantly hinder the startup's growth and credibility. For a startup to navigate the evolving AI landscape successfully, a co-founder needs to demonstrate a solid grasp of model documentation best practices and be able to effectively manage the complex details inherent in AI development through proper documentation.
While the importance of documentation in traditional software development is well-established, the specific needs for documenting AI models remain somewhat unclear, at least from my perspective as a researcher. It's becoming increasingly evident that the current approaches to AI model documentation might not be adequate. For example, many AI projects don't meticulously record model configurations, like hyperparameters. This oversight can lead to reproducibility problems, as even minor changes in settings can result in dramatically different model outputs. It becomes difficult to truly understand and iterate upon model performance without knowing exactly what was done in the initial build and subsequent iterations.
Further complicating matters is the lack of a standardized approach to documenting AI model updates and the lineage of training data. Without a clear understanding of how models have evolved and what data shaped them, it's challenging to maintain a complete picture of their capabilities and limitations throughout their lifecycle. This can significantly hinder the ability to optimize performance after deployment.
The ambiguity around documentation requirements extends into potential legal complexities, particularly as AI models are increasingly used in situations with ethical implications. If a model produces results that exhibit bias or discrimination, having a clear record of how the model was developed becomes crucial to defend its legitimacy.
Beyond legal ramifications, poor or nonexistent documentation creates roadblocks for collaboration within engineering teams. It can become a frustrating time sink trying to piece together the state of a project if there's not a cohesive documentation effort. When this happens, the team's focus shifts from innovation to simply understanding the current state of the AI model, which can noticeably slow down productivity.
Adding to these challenges is the lack of a systematic approach to documentation in many organizations. Without clear guidelines for documenting models, the ability to reliably track performance metrics, ensure accountability, and understand the model's history become far more difficult. It's like trying to navigate without a map in a complex and constantly changing landscape.
Interestingly, a surprising number of technically capable co-founders tend to underestimate the long-term impact of poorly governed documentation practices. Failing to prioritize good documentation from the very start can create headaches later when a startup needs to pivot or expand its business. Having a clear and well-maintained set of records for models can be instrumental when changing directions or introducing new functionalities.
Furthermore, startups need to consider that more rigorous compliance audits related to AI are becoming the norm. If they lack a robust documentation system, the ability to meet audit requirements will be severely challenged. And in the complex world of AI regulation, any inability to provide the needed evidence could lead to substantial fines and other difficulties.
Beyond operational aspects, there's a communication hurdle that lack of documentation creates when interacting with stakeholders. Often, there's a disconnect between the detailed engineering work and a compelling narrative of the model's value proposition. Without proper documentation outlining the rationale behind design decisions, it's hard for external parties to truly appreciate the strengths and limitations of the AI system. This can impact funding opportunities and overall project viability.
In my observations, it's surprising how many individuals involved in AI development struggle to find past records on model development. This recurring issue can be frustrating for developers as teams frequently rediscover previously identified challenges or make the same mistakes. This emphasizes that having a system for maintaining a detailed history of a project's development can significantly reduce the chance of recurring errors.
Finally, there's a strong correlation between good documentation and project sustainability. Organizations that prioritize comprehensive documentation practices tend to have greater success maintaining their AI projects over longer time horizons. These well-documented projects are better equipped to facilitate onboarding of new team members and adapt to shifting needs as the projects mature.
In summary, while the world of AI is rapidly evolving, the lack of a clearer and standardized understanding of model documentation requirements continues to pose challenges. This gap in understanding has the potential to negatively impact reproducibility, collaboration, project longevity, and more. This is certainly a field where further research and development of clear best practices are necessary to optimize the development and deployment of AI systems.
7 AI-Related Red Flags When Evaluating a Technical Co-Founder for Your AI Startup A Patent and IP Perspective - Limited Knowledge of Current AI Patent Litigation Landscape
The AI patent landscape is becoming increasingly complex, with a significant rise in patent filings and a corresponding increase in related legal disputes. The high rate at which AI-related patents are being granted underscores the importance of securing them to protect innovations in this competitive field. However, navigating this landscape requires a nuanced understanding of AI patent litigation, something that's crucial for anyone involved in AI startups, particularly technical co-founders. Failure to grasp the intricacies of AI patent law can lead to significant risks for a company. Furthermore, recent legal decisions have introduced complexities around patentability related to whether a human inventor is required, further highlighting the importance of being well-versed in the legal aspects of AI patents. Therefore, a lack of awareness of the current AI patent litigation landscape can be a critical red flag when evaluating a potential co-founder, as it suggests a potential blind spot in a critical area of AI startup development.
The legal landscape surrounding AI patents is becoming increasingly complex and contested. Patent disputes related to AI have surged in recent years, with a greater than 50% annual increase over the last five years. It's a bit surprising that many of these disputes center on core, foundational elements of AI, like how data is processed and algorithms are deployed, indicating a high level of competition in even the most basic areas of the technology.
It seems that a substantial number of AI patent applications, more than 30%, get initially rejected by patent offices due to questions about whether they are truly new or sufficiently useful. This suggests that the process of obtaining patent protection for AI is far from straightforward. Furthermore, there's a growing trend of "patent trolling" where some entities seem to be buying up patents just to sue companies for licensing fees, rather than actually developing technology themselves. This creates a very confusing environment for startups trying to navigate patent law.
While the US has been a leader in AI patent filings, it's interesting that countries like China and Germany are rapidly developing their own patent systems. This is changing the global dynamics of AI innovation, and it might influence where AI startups choose to focus their efforts. The concept of "patent thickets" is another growing concern. With so many overlapping patents in the AI space, it's becoming more difficult to innovate without potentially infringing on someone else's intellectual property.
Many AI startups appear to not fully appreciate the value of patent marking, a process where you indicate on your products that you hold a patent. This can be quite useful in negotiating licensing agreements and discouraging infringement, but it's often overlooked. The emergence of open-source AI software also creates a bit of a paradox. On one hand, it allows for rapid innovation, but on the other, it increases the risk of accidentally infringing on existing patents. This creates a tricky balancing act for startups.
Another area where startups often stumble is failing to disclose all relevant prior art when applying for a patent. If you don't fully disclose what's already been done in the field, your patent could be invalidated, leaving your technology vulnerable to competitors. The average time to get an AI patent granted is around 24 months, which might sound reasonable, but it's actually quite a long time in a field like AI where things are changing so quickly. Startups could lose their market position while waiting for a patent to be approved. This highlights the importance of having a good patent strategy early on.
It's clear that understanding the ever-evolving AI patent landscape is crucial for any AI startup. The complexities of patent law, the increasing competition, and the changing global landscape present significant challenges that need to be considered from the beginning.
7 AI-Related Red Flags When Evaluating a Technical Co-Founder for Your AI Startup A Patent and IP Perspective - Insufficient Background in AI Ethics and Compliance Standards
When evaluating a technical co-founder for your AI startup, a lack of understanding regarding AI ethics and compliance standards is a major red flag. In an increasingly regulated field, a weak foundation in the ethical implications of AI applications can expose your company to legal hurdles and reputational harm. It's crucial for AI startups to embrace responsible development practices, especially when dealing with sensitive data, bias concerns, and the need for transparency.
Furthermore, a co-founder who doesn't grasp the evolving ethical guidelines within the AI sector could struggle to secure funding or attract valuable partnerships. Investors and collaborators are increasingly mindful of ethical considerations, often making them a key factor in their decision-making process. Simply put, a co-founder's inability to demonstrate a thorough grasp of AI ethics and compliance not only poses immediate operational challenges but also questions the core principles upon which your startup is built. Without a commitment to ethical development and adherence to relevant standards, the long-term prospects and integrity of your AI venture can be seriously jeopardized.
When evaluating a technical co-founder for an AI startup, a lack of understanding and experience in AI ethics and compliance standards is a significant red flag. It's not simply a matter of checking a box, but rather recognizing that the field of AI ethics is constantly evolving and often lacks clear consensus. While the push for AI regulation is growing, with many countries and organizations beginning to put frameworks in place, it's easy to see how a co-founder might not be aware of the latest developments.
The ethical implications of AI are multifaceted and often debated. For example, core ethical principles like fairness, accountability, and transparency are still under scrutiny, and the consensus on their practical application within AI systems is quite variable. There seems to be a noticeable gap between the rapid pace of AI development and the establishment of solid ethical frameworks. Many AI developers are worried that current standards are not keeping up with the technological advancements, and many startups don't include AI ethics training as a core component of their development processes.
A startup that doesn't incorporate ethical considerations from the start risks producing biased or discriminatory outputs. This can be a significant issue in AI-powered systems that affect decision-making across many sectors. Additionally, a lack of focus on ethical compliance can lead to difficulties in attracting investment, as many venture capitalists are increasingly prioritizing startups that can demonstrate a commitment to responsible AI innovation. Also, with the increasing focus on AI ethics and regulation, not having the correct level of knowledge could create issues with compliance, as startups navigate conflicting guidelines from different governing bodies.
The lack of adequate attention to ethical considerations can also lead to poor documentation of ethical reviews and decisions. This gap in recording information related to AI ethics can impact the long-term success of an AI startup, and can lead to misunderstandings related to the technology itself. Overall, a potential co-founder who lacks an awareness of AI ethics and compliance standards could create future challenges for a startup, from reputational damage to legal issues, and in a space that's so competitive, it's a risk that's worth considering carefully. It's becoming increasingly clear that building ethical AI systems requires a proactive and conscious effort from the very start, and a co-founder needs to have a comprehensive understanding of this aspect to guide a company successfully.
AI-powered Trademark Search and Review: Streamline Your Brand Protection Process with Confidence and Speed (Get started for free)
More Posts from aitrademarkreview.com: