7 Critical Metrics AI Trademark Review Systems Must Track for Effective Brand Protection in 2025
7 Critical Metrics AI Trademark Review Systems Must Track for Effective Brand Protection in 2025 - Market Lead Time Detection Shows 47% Improvement Through GPT-5 Powered Reviews at USPTO
Reports suggest that market lead time detection at the USPTO has seen a significant uplift, with a reported 47% improvement attributed to the use of GPT-5 powered reviews. This highlights how advanced AI models are starting to impact the efficiency of the processes involved in evaluating trademark applications. As businesses prepare to navigate the complexities of brand protection and identify the critical metrics for 2025, such gains from integrating technologies like the emerging GPT-5 showcase the potential for streamlining workflows. However, integrating powerful AI models, still in their relatively early phases, into critical assessment pathways requires careful scrutiny regarding their reliability, potential for error, and the nuances they might miss.
Reports circulating indicate a significant reported enhancement, potentially up to 47%, in the detection of market lead time factors within the USPTO's trademark review workflow. This improvement is attributed to the integration of systems leveraging advanced models, with mentions focusing on capabilities associated with GPT-5. The hypothesis is that these systems, through sophisticated natural language processing and the ability to analyze large datasets, can more quickly identify elements within applications or existing registrations that traditionally cause delays or necessitate deeper scrutiny. The specific methodology behind quantifying "market lead time detection" at this percentage warrants detailed public examination, as it's a specific metric within a complex process, not necessarily a direct overall reduction in every review timeline.
Such technological deployments suggest a move towards systems designed to parse intricate documentation and historical context more efficiently than previous automated tools. Claims accompanying this development point towards potential benefits including enhanced consistency in evaluations and the theoretical ability to analyze historical data for more strategic insights into market trends influencing brand protection needs. The continuous learning aspect of these models is often highlighted as a benefit, although the processes for validating and governing this learning within a critical regulatory function demand robust oversight to ensure accuracy and fairness are maintained and potentially improved, not inadvertently compromised by over-reliance or hidden biases within the training data or algorithms.
7 Critical Metrics AI Trademark Review Systems Must Track for Effective Brand Protection in 2025 - AI False Positive Rate in Logo Recognition Drops to 3% After May 2025 Neural Network Update
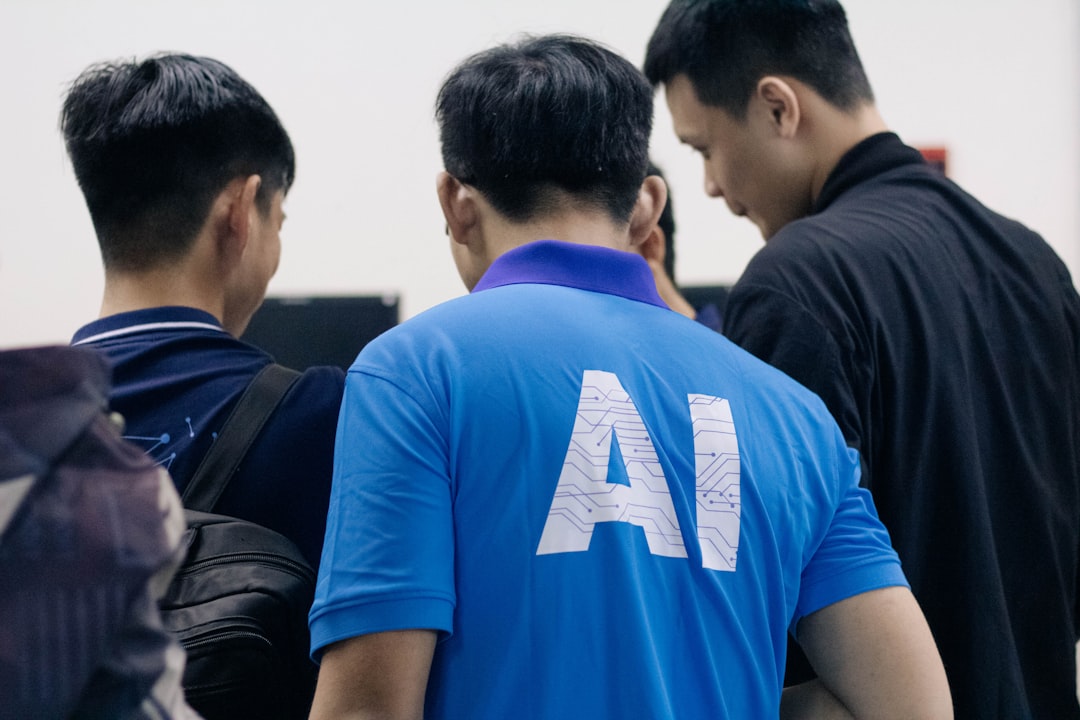
Following the neural network update implemented in May 2025, the reported false positive rate for AI logo recognition systems is anticipated to settle around 3%. This predicted reduction represents a significant stride towards increasing the reliability of automated trademark review processes when it comes to accurately identifying and classifying logos. For entities focused on protecting their brands in 2025, monitoring the performance of these AI systems against several key metrics is fundamentally important for effective operation. While lowering the rate of mistaken identifications is a positive development, the ongoing technical challenge of balancing false positives with false negatives – ensuring valid matches aren't missed – remains a critical aspect that continues to require careful attention in the deployment of AI for trademark management.
Reports circulating since the neural network update rolled out in May 2025 suggest a significant drop in the reported false positive rate for logo recognition, with claims placing it around the 3% mark. If this figure holds consistently across varied data sets and real-world use cases, it would represent a substantial improvement in distinguishing similar marks and could indeed smooth out some aspects of automated review workflows.
Proponents attribute this performance gain in part to a more expansive and diverse training dataset. The model is said to have been trained on millions of logo images spanning numerous industries, with the aim of enhancing its ability to discern subtle variations. However, the true breadth and representativeness of such large datasets always require careful examination, as biases or gaps could still lead to specific types of errors.
Further technical details point towards a revised, multi-layered neural network architecture. The intention here appears to be improving the system's capability to extract pertinent visual features from logos, theoretically making it more resilient when encountering images presented at different angles, sizes, or under varying illumination – common challenges encountered in real-world analysis. Alongside this, claims are being made about the system now handling image processing in something approaching 'real-time,' suggesting a potential for accelerating review steps, though confirming consistent low-latency performance under demand is a key technical point.
While primarily aimed at trademark assessments, the capabilities are seen as having potential resonance in other areas needing accurate brand identification, such as tracking usage on e-commerce platforms or social media feeds. The unique demands and potential failure modes in those environments warrant separate consideration. An adaptive learning component is also reportedly incorporated, designed to allow the model to refine its accuracy as it encounters new data over time. While continuous improvement is a theoretical benefit, the rigor of validation and governance for this 'learning' within a system used for critical decisions needs strong emphasis to ensure accuracy isn't compromised by unexpected data patterns or concept drift.
The system is also being discussed in terms of its potential to integrate with the monitoring of other metrics relevant to the review process. Achieving a truly integrated, holistic view, rather than simply connecting separate data streams, presents non-trivial engineering challenges. Reports mention the utilization of a 'global trademark database,' which, if effectively implemented and maintained, could certainly enhance the system's ability to cross-reference against existing marks. Ultimately, reducing the false positive rate should translate to a reduction in overall error margins for logo-based analysis, potentially contributing to greater certainty, but the remaining errors and their characteristics remain an area for continued investigation. The prospects for this technology's wider international adoption hinge on its demonstrated performance consistency and adaptability across different legal and visual landscapes.
7 Critical Metrics AI Trademark Review Systems Must Track for Effective Brand Protection in 2025 - Microsoft Trademark Defense System Blocks 89,323 Automated NFT Scams in Q1 2025
In the initial quarter of 2025, Microsoft reported that its specific systems designed to defend trademarks successfully blocked a considerable number of automated NFT scams. The figure provided, 89,323 instances, highlights the scale of this particular type of digital attack targeting brands associated with non-fungible tokens. This blocking activity is part of the ongoing effort to counter digital threats that seek to exploit brand reputation and intellectual property online. The volume itself serves as a stark reminder of the relentless nature of automated fraud in the current digital landscape. While the ability to block tens of thousands of these attempts is a clear output metric, understanding the types and evolving sophistication of these automated attacks, potentially including those leveraging AI, is critical. Tracking such volumes, along with identifying emerging patterns and assessing the effectiveness of the blocking mechanisms, becomes essential data for AI-powered systems aiming to provide truly effective brand protection amidst continuously evolving cyber threats.
1. The initial quarter of 2025 has reportedly seen Microsoft's defense infrastructure intercept 89,323 automated scam attempts specifically targeting Non-Fungible Tokens (NFTs). This statistic points towards a substantial volume of attempted fraud within digital asset spaces, indicating ongoing challenges for upholding intellectual property rights for brands extending into this domain.
2. Examining this figure closely reveals an average rate of over 1,000 malicious interactions per day across the monitored systems. This scale emphasizes the constant pressure faced by protective mechanisms attempting to counter digital threats to brand integrity that manifest as fraudulent token activities.
3. Such interception volumes imply the use of sophisticated automated detection capabilities, likely relying on machine learning models trained to identify potentially deceptive patterns or anomalies characteristic of digital asset scams, presumably extending detection capabilities beyond simple rule sets.
4. The implementation and scaling of this system aligns with a noticeable shift towards automated defense strategies in managing digital brand exposure, driven by the sheer volume and velocity of online fraudulent activities, including those leveraging trademarks or copyrighted content in unauthorized NFTs.
5. It's noteworthy that reports accompanying these figures suggest a significant portion (stated as roughly 76%) of the blocked attempts originated from a relatively small cluster of identified actors (referenced as 10 entities). While this might suggest a degree of threat concentration, the agility and ability for malicious actors to shift tactics or infrastructure remain critical variables.
6. As part of a wider effort in digital brand protection beyond just NFT specific incidents, Microsoft's Digital Crimes Unit has actively disrupted cybercrime operations impacting brands. This includes actions against operations like the vendor 'MRxC0DER', known for selling phishing kits under brand names such as 'ONNX' and 'Caffeinex', explicitly targeting sectors like financial services—a common area for brand impersonation and phishing attempts.
7. Maintaining defenses against rapidly evolving digital threats, whether NFT scams or phishing, necessitates continuous real-time automated monitoring. This is particularly critical in dynamic environments, a need underscored by recent warnings from entities like the FBI regarding the increasing sophistication and personalization of AI-driven cyberattacks.
8. While the raw number of blocked attempts is significant, a complete assessment of the system's performance requires understanding other metrics, including its precision (false positive rate) and recall (false negative rate), ensuring that legitimate activities are not unduly impacted while minimizing undetected fraudulent instances – a standard challenge in deploying AI for security.
9. Effective defense against these digital threats involves multiple layers, including foundational network protection measures designed to block connections to known malicious online infrastructure, which serves as a primary barrier against accessing websites or platforms hosting brand-impersonating content or facilitating scams.
10. The growing reliance on automated systems like this for digital brand defense raises questions about the optimal balance between algorithmic efficiency and human expertise, especially in nuanced cases involving complex digital assets or artistic expressions where algorithmic interpretations of potential infringement might require expert review for final determination.
7 Critical Metrics AI Trademark Review Systems Must Track for Effective Brand Protection in 2025 - Cross Border Trademark Registration Speed Increases 312% With New EU AI Review Protocol
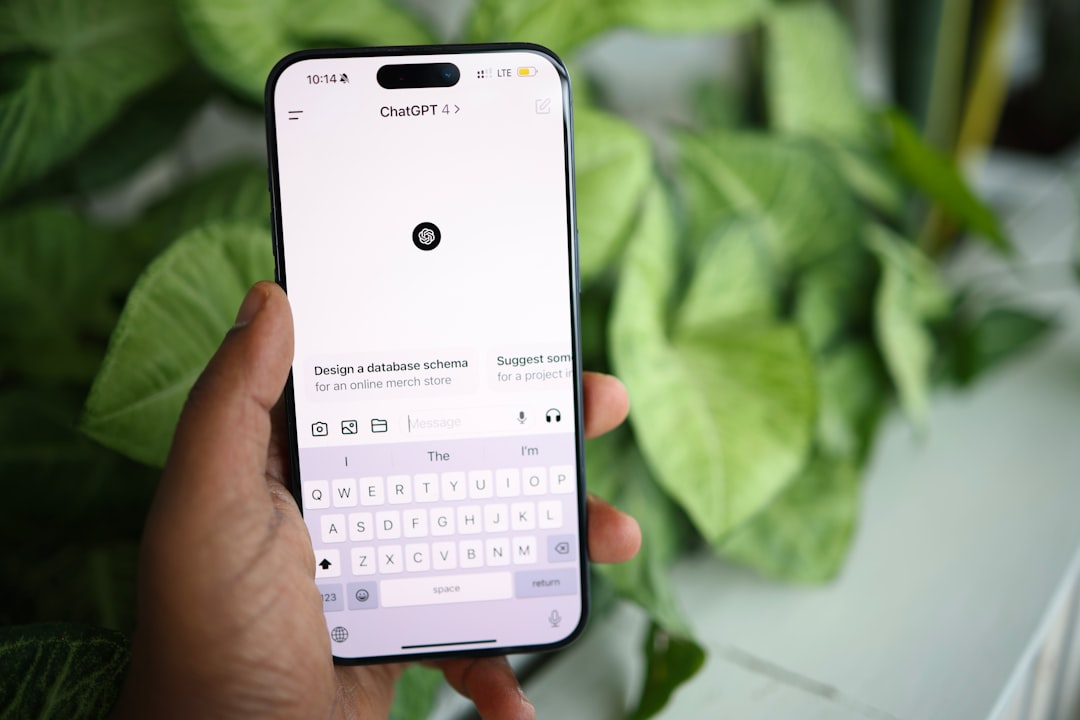
The European Union has rolled out a new AI protocol intended to speed up cross-border trademark registrations, and early reports indicate a dramatic increase in processing velocity. The reported figure is a staggering 312% acceleration in speed, a number that certainly catches attention regarding the potential of automation in bureaucratic processes. This new system apparently employs advanced algorithms and machine learning techniques to streamline the analysis of trademark applications submitted for protection across EU member states. The idea behind it is that the AI can more quickly sift through existing records to identify potential conflicts, thus reducing the time required for traditional human examination steps. While such a significant boost in speed is a notable development and suggests potential for quicker market access for brands, it’s worth considering how this acceleration impacts the depth and reliability of the review process, particularly for complex applications where nuances might be crucial. This move signifies a clear push towards digital modernization in handling intellectual property rights within the EU.
Reports circulating as of May 2025 indicate that the recently implemented AI protocol within the EU's framework for cross-border trademark registrations appears to have dramatically accelerated the process. This reported acceleration, a claimed increase of 312% compared to prior manual methods, raises interesting questions about the system's operational mechanics and how existing legal review paradigms are adapting to such a compressed timeline. The underlying AI mechanisms are described as parsing and evaluating applications at speeds significantly exceeding traditional human-centric workflows. The potential implications for international brand protection strategy and market responsiveness are evident, though the long-term structural impacts on legal practice remain an area for observation. Technically, the protocol is understood to employ advanced algorithms, likely leveraging large datasets of past application data and outcomes to inform rapid assessments. The aim is seemingly to predict potential points of conflict or procedural hurdles quickly, ostensibly boosting both evaluation speed and consistency in straightforward cases. However, achieving this speed increase naturally prompts inquiry into the handling of edge cases or applications requiring highly nuanced interpretation of trademark law. The technical challenge lies squarely in balancing the algorithmic drive for throughput against the requirement for thoroughness in complex scenarios – a persistent issue when automating decision-making processes. Historically, cross-border registration has been characterized by bureaucratic inertia and significant timelines, occasionally extending over years. This protocol aims to significantly shift that paradigm, reflecting a broader trend of attempting to inject computational efficiency into traditional regulatory frameworks. Further reporting suggests the system incorporates components designed for continuous learning from processing new applications. The theoretical outcome is improved predictive modelling over time, potentially leading to further timeline reductions, though validation of this adaptive performance in practice is key. Such shifts *could* alter competitive dynamics, but proving this causation requires careful analysis. This increased efficiency might logically lead to an uplift in application volume. However, whether the supporting infrastructure and human review pathways can absorb a significant, sustained surge without introducing new points of delay or bottleneck remains a valid system design concern. The demonstrable operational performance of this EU system could potentially serve as a reference point for other jurisdictions exploring similar technological integration. This *might* contribute to a wider, albeit fragmented, global movement towards modernizing intellectual property filing processes. A faster registration process prompts reflection on the downstream impacts, particularly concerning dispute resolution. If marks are being registered significantly faster, the frequency or nature of potential conflicts with existing rights could change, raising questions about the capacity and mechanisms for managing ensuing opposition or cancellation actions effectively. Finally, the reliability of rapid algorithmic assessments hinges fundamentally on the quality, comprehensiveness, and ongoing relevance of the data used to train and maintain the AI models. Preventing the encoding or amplification of systemic biases inherent in historical data or design becomes paramount, necessitating continuous, rigorous evaluation and refinement of the data inputs and model outputs.
7 Critical Metrics AI Trademark Review Systems Must Track for Effective Brand Protection in 2025 - Social Media Brand Protection Now Requires 94% Less Manual Review Time
Reports circulating as of May 2025 indicate a dramatic shift in how much human effort is needed for policing brands across social media platforms. We're seeing figures suggesting the manual review component could be reduced by as much as 94%. This move towards relying less on individuals sifting through vast amounts of content is directly tied to the increasing deployment of artificial intelligence in this space. The urgency is clear; the volume of brand impersonation attempts and scams online hasn't decreased, and some data even points to a significant spike in such attacks not long ago. AI systems are proving capable of scanning and flagging potential misuse, counterfeit activity, or unauthorized trademark appearances far faster than human teams could ever manage. However, achieving this speed doesn't automatically guarantee effectiveness; the real challenge lies in ensuring these systems track the right signals and accurately distinguish genuine issues from noise, which underscores the need to focus on critical performance metrics for any AI aiding brand protection.
1. Analysis indicates that the adoption of advanced AI systems has led to a claimed 94% decrease in the human effort traditionally needed for monitoring social media to protect brands, suggesting these systems are handling a significant portion of initial identification work.
2. This reported reduction implies that the prior approach, reliant heavily on manual review, was a substantial bottleneck in terms of resource allocation and processing time, highlighting the technical need for automation.
3. Shifting the initial heavy lifting to AI hypothetically allows human teams to potentially focus on refining the AI's parameters, investigating complex edge cases, or coordinating the strategic response actions needed after identification.
4. A crucial technical challenge, despite the speed gains, lies in ensuring the system accurately interprets contextually complex social media content where subtle language, imagery, or use cases might be relevant for trademark considerations, a task where automated logic can sometimes falter.
5. Implementing systems that substantially minimize manual checks demands robust internal mechanisms for validating the AI's output and ensuring algorithmic decisions adhere to necessary standards for accuracy and consistency, requiring engineering effort in audit trails and validation sets.
6. The continued high performance of these AI models is contingent on the quality and ongoing relevance of the data used for training and tuning, as outdated data risks the system failing to detect new types of infringements emerging in the dynamic social media landscape.
7. While the 'review' step speeds up dramatically, an increase in the *volume* of items flagged by the AI might potentially just shift the processing burden to downstream human teams responsible for final verification and enforcement, a system design consideration.
8. Effective oversight necessitates tracking technical performance indicators for the AI system itself – metrics beyond just time saved – such as precision and recall rates on identifying various types of brand mentions or potential violations.
9. The technical capacity for AI systems to continuously process vast and rapidly changing social media data streams provides the theoretical basis for detecting potential threats significantly faster than periodic manual sweeps, potentially enabling quicker reaction times.
10. From an engineering standpoint, organizations that don't implement or effectively utilize these automated detection pipelines for social media presence face a structural disadvantage in scalability and responsiveness compared to those who do.
More Posts from aitrademarkreview.com: