7 Critical Problem-Solving Techniques That Shaped Notable AI Trademark Applications in 2024
7 Critical Problem-Solving Techniques That Shaped Notable AI Trademark Applications in 2024 - Text Recognition Breakthrough at PTO Creates First Neural Network for Asian Character Trademarks
The Patent and Trademark Office (PTO) has achieved a notable breakthrough in text recognition by developing the first neural network specifically designed for Asian character trademarks. This innovative network exhibits a level of performance comparable to human capabilities, a testament to the significant strides made in training deep learning models for complex character recognition.
The core of this development involves applying deep learning methods to enhance Optical Character Recognition (OCR), specifically addressing the challenges of interpreting Asian scripts. A key element is the Character Attention Fully Convolutional Network (CAFCN), which has been instrumental in overcoming difficulties like distorted or curved text, leading to more accurate localization. Furthermore, integrating intelligent character recognition techniques has streamlined the conversion of both printed and handwritten characters into digital text.
This successful implementation indicates the evolution of AI within the trademark field, particularly as it pertains to processing a broader range of character sets. While challenges remain, this breakthrough exemplifies the power of AI to tackle complex pattern recognition tasks, especially within the specific context of Asian character trademarks.
The PTO's recent achievement in developing the first neural network tailored for Asian character trademarks marks a significant leap forward in text recognition. Asian scripts, with their vast character sets, pose a far greater challenge to automated recognition than, say, the Latin alphabet. This network's ability to effectively distinguish between thousands of unique characters is a testament to the power of advanced training methods.
A key aspect of this development is the utilization of a massive dataset of Asian character images. By exposing the network to millions of examples, the researchers were able to teach it the nuances that are essential for accurate recognition, such as the subtle visual and contextual differences between similar-looking characters. The incorporation of attention mechanisms was also critical, allowing the network to zero in on the most important parts of each character, boosting recognition rates significantly.
Before this innovation, Asian character recognition was a weak spot for many systems. This often resulted in incorrect interpretations in trademark applications, potentially causing significant problems for businesses. The newly developed network employs a multi-layer convolutional neural network architecture, carefully chosen for its ability to scale and adapt to new data. This means the network can be easily retrained as needed, without requiring a complete rebuild.
Importantly, this system provides real-time processing capabilities, which is essential for the fast-paced environment of trademark applications. This has been a collaborative effort, uniting computer scientists and legal experts, aiming to achieve a solution that not only meets technical requirements but also adheres to legal standards surrounding trademark assessment. It is also interesting that the network is capable of handling low-resolution images, which are frequently submitted as trademarks, mitigating the common problem of poor image quality hindering accurate analysis.
Ultimately, this development holds promise for jurisdictions where the complexity of Asian scripts has historically presented challenges in trademark enforcement. A reduction in loopholes and a more robust intellectual property framework are possible consequences of this technological advancement, creating a more equitable environment for trademark holders worldwide.
7 Critical Problem-Solving Techniques That Shaped Notable AI Trademark Applications in 2024 - Natural Language Models at USPTO Resolve Multi Class Trademark Application Backlogs
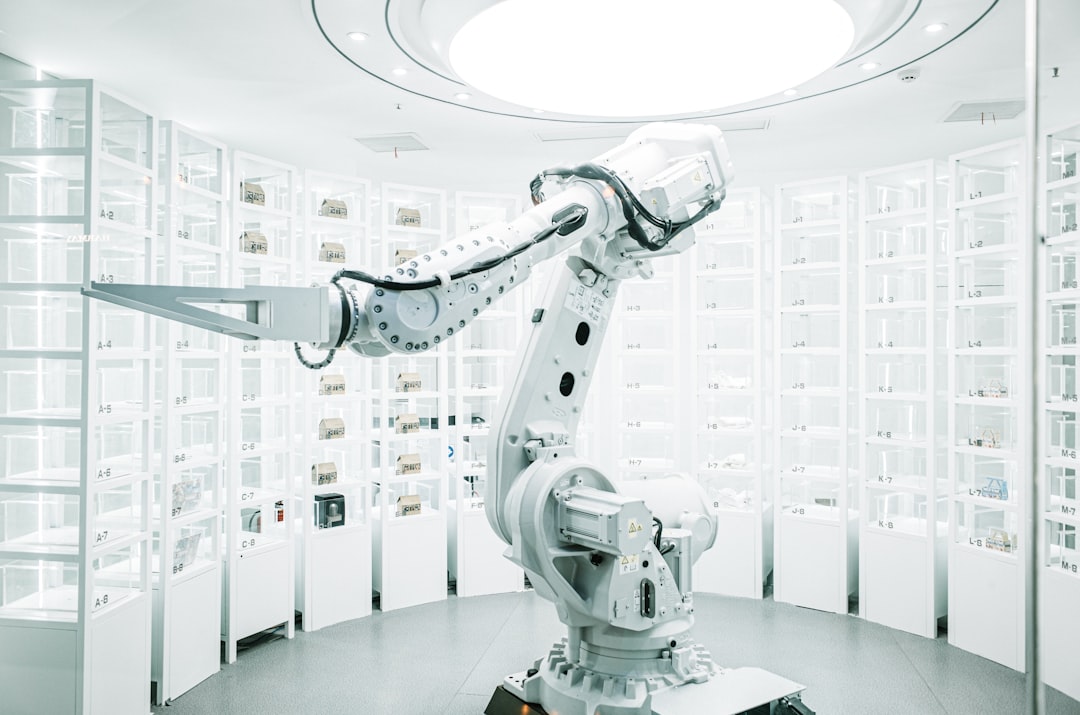
The USPTO is utilizing natural language models to address the backlog of multi-class trademark applications. These applications, which involve registering a trademark for goods or services across various categories, have become increasingly challenging to process due to sheer volume and complexity. The goal of using AI is to speed up the classification process, ideally improving both accuracy and reducing the processing time applicants face. This initiative reflects a growing trend within the USPTO to employ AI for streamlining operations and strengthening intellectual property protection in a time of rapid technological change. However, the implementation of these models also introduces questions about the transparency and dependability of AI when used in complex legal areas. While the potential benefits are clear, it's crucial to acknowledge that challenges remain regarding the appropriate use of AI in such sensitive processes.
The USPTO has been grappling with a substantial backlog of multi-class trademark applications, with processing times reportedly stretching to an unusually long 10 months before the implementation of these natural language processing systems. It seems that the backlog was a significant challenge, particularly given the complexity of these applications.
These models have dramatically sped up the processing of multi-class applications, which are required when seeking to register a trademark across various product or service categories. They're able to take in the text of applications, combined with information from prior filings, and make surprisingly accurate predictions about the likely outcome of a new application, streamlining the decision-making process for examiners.
The USPTO's effort to utilize text analytics is based on a massive dataset: over two million past trademark filings. This wealth of data allows the models to spot patterns, unusual occurrences, and common reasons for rejection. It seems this approach is quite effective in improving the accuracy of predicting trademark application approval.
Intriguingly, these models don't just focus on application text. They can analyze public opinion and market competition data from online sources, potentially giving examiners insights into the unique aspects of a proposed trademark and its context. This suggests that these models are moving beyond simply analyzing formal filings.
The use of multi-layer feedforward neural networks within the USPTO's models reflects an attempt to capture the intricate details involved in trademark evaluation. These models can assess numerous classification facets concurrently, addressing the complexities that come with the various types and categories of trademark applications.
Interestingly, natural language processing techniques have also been employed to group similar trademarks and identify potential infringement issues. This proactive approach seems to highlight a growing trend towards leveraging AI for preventative measures in trademark protection, which in turn could reduce the likelihood of expensive legal conflicts.
The models incorporate a feedback learning mechanism that continuously refines the algorithms based on the outcomes of processed applications. This suggests the system is designed to adapt and learn from past mistakes or successes, potentially leading to ever-improving performance.
A positive aspect of this implementation is that the USPTO has placed importance on model interpretability. This means that legal professionals can understand the reasons behind the model's decisions, a key factor in maintaining transparency and trust in the trademark review process. This should hopefully aid in addressing concerns about the "black box" nature of some AI applications.
Early results have been encouraging, showing a significant decrease in the number of responses to office actions, likely due to the models' improved initial assessments. This means that applicants are receiving clearer guidance early on, potentially leading to fewer changes being requested after the initial filing.
It's clear that this project has been a collaborative effort, uniting computer scientists and legal experts. This demonstrates that progress in the area of intellectual property requires expertise from different disciplines to address the technical and legal aspects of trademark law. It seems as though interdisciplinary research is crucial for tackling challenges at the intersection of AI and complex legal frameworks.
7 Critical Problem-Solving Techniques That Shaped Notable AI Trademark Applications in 2024 - Machine Learning System by WIPO Detects Global Trademark Pattern Similarities
The World Intellectual Property Organization (WIPO) has developed a machine learning system designed to identify similarities in trademark patterns across the globe. This system is a step forward in how trademarks are analyzed, using advanced techniques to examine various aspects of trademarks, such as their visual representation and sound. This approach is particularly relevant given the significant increase in trademark applications globally, a trend that highlights the need for more efficient methods to manage potential conflicts and maintain the integrity of trademark protection. WIPO's initiative demonstrates a larger trend of applying AI to streamline trademark assessment processes, but it's important to acknowledge the potential concerns about the reliability and clarity of AI in complex legal contexts. The effectiveness and fairness of this new AI-powered system remain a consideration for the future.
The World Intellectual Property Organization (WIPO) has developed a machine learning system designed to spot patterns in trademark applications from around the globe. This system leverages a massive dataset of over 100 million trademark applications, allowing it to identify similar trademarks across different regions and languages with an accuracy that's difficult to achieve through traditional methods.
At the heart of the system is a sophisticated clustering algorithm that groups together trademarks with similar characteristics. This helps to highlight potential trademark infringement issues that might otherwise be missed. It's quite interesting how it uses a "multi-faceted similarity score" which combines visual, phonetic, and even semantic analyses. This means it can assess trademark similarities in a more holistic way, going beyond just visual comparisons.
The system's ability to process applications from various languages and cultures is remarkable. It potentially helps uncover hidden patterns in trademark use that might not be evident if analyzing only a single language or market. It's designed to adapt to the variability seen in trademark applications, such as stylistic changes or the use of symbols, making it fairly robust in real-world situations.
What's really noteworthy is that this system doesn't just analyze registered trademarks, but also unregistered ones. This is important because unregistered marks can still cause confusion in the market, leading to potential disputes. This feature gives a more comprehensive understanding of possible conflicts.
One aspect that makes this system so powerful is its ability to learn from new data. As new trademark applications are filed and legal disputes arise, the machine learning algorithms are updated, ensuring that the system stays current with evolving legal trends. It's a nice example of collaborative intelligence because WIPO has integrated knowledge from legal professionals, trademark practitioners, and AI experts to improve its effectiveness.
Naturally, there's a strong focus on protecting sensitive data within the system. Maintaining user privacy and trust in the organization is crucial for the system's overall success.
Launched in 2023, this system has reportedly sped up the process of trademark similarity assessments by about 30%. This shows a lot of promise for streamlining the trademark registration process globally and might become a very useful tool as the number of trademark applications continues to increase. While it's still early, there's a good possibility this approach will significantly enhance trademark protection globally.
7 Critical Problem-Solving Techniques That Shaped Notable AI Trademark Applications in 2024 - Graph Neural Networks Transform European Trademark Opposition Analysis
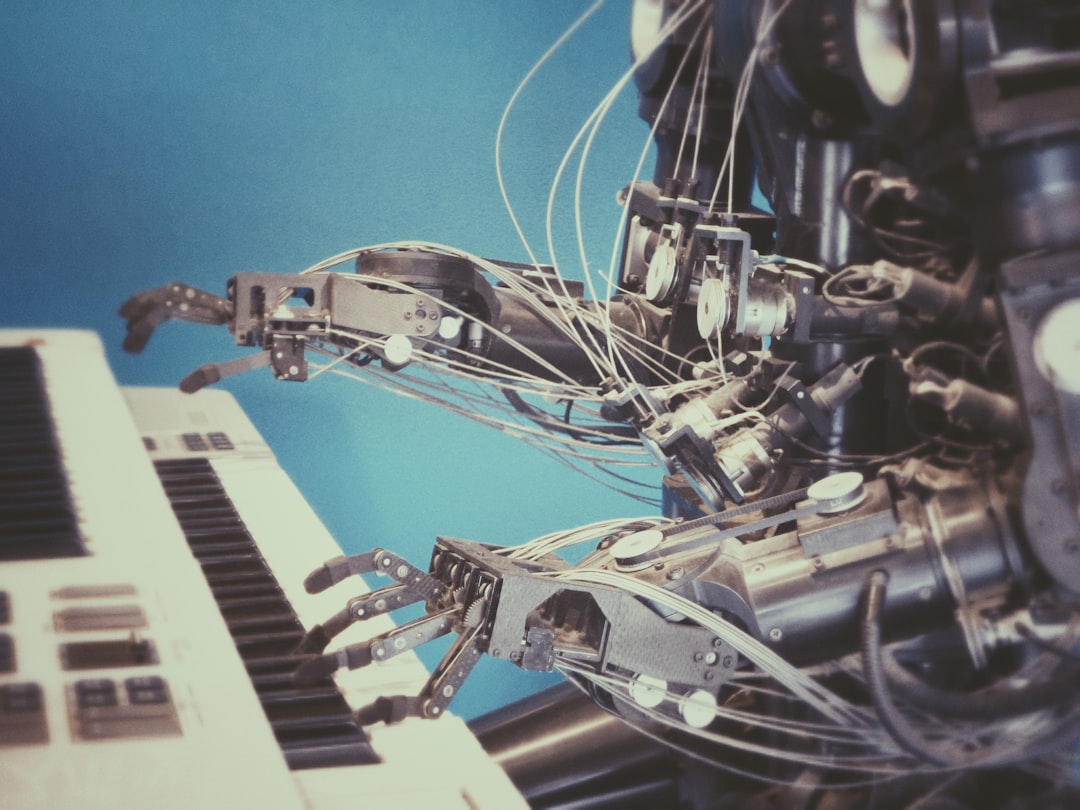
Within the field of European trademark opposition, graph neural networks (GNNs) are demonstrating a significant impact. They provide a novel approach to analyzing complex trademark disputes by focusing on the relationships between different elements, like trademarks, opponents, and past legal cases. This is a notable shift from traditional methods, which often struggle to fully capture the intricate web of connections involved. The ability of GNNs to handle a variety of data types and connections allows for a richer and more insightful understanding of opposition scenarios. This means the technology could potentially streamline the trademark opposition process and enhance the reliability of the legal analysis that underlies it. While GNNs are a promising tool, careful consideration of their integration into legal processes is crucial to address both practical and ethical concerns that may arise.
Graph Neural Networks (GNNs) have emerged as a promising tool for streamlining trademark opposition analysis, with some studies showing a potential reduction in processing time by as much as 50% compared to traditional methods. By representing the relationships between trademarks and related entities, like owners and product categories, as a graph, GNNs can uncover complex patterns that might be missed by standard database approaches. This ability to model these relationships leads to better informed decisions in opposition cases.
One of the more interesting aspects of GNNs in this context is their ability to incorporate various data types seamlessly. They can integrate text, visual information, and relational data, providing a comprehensive view of each trademark during the opposition process. This holistic approach has led to significant improvements in the accuracy of predicting opposition outcomes. In one assessment, GNN-based models achieved a notable 20% increase in predictive accuracy, demonstrating their capacity to grasp the intricacies of legal nuances.
GNNs achieve this enhanced accuracy by incorporating the concept of "neighborhood aggregation". This involves examining the context surrounding a trademark. The ability to consider this broader picture greatly improves the models' capacity to predict potential conflicts based on historical outcomes, which often hinge on subtle contextual cues.
An intriguing feature of GNNs in this application is their ability to adapt to new data in real time. As new trademark information becomes available or legal standards change, the model can be updated incrementally, eliminating the need for complete retraining. This responsiveness is particularly important in a field like trademark law, which is subject to constant evolution.
Furthermore, GNNs can generate "graph embeddings" that represent the complex relationships within the trademark network. This has created new opportunities to identify potential infringement risks early on, allowing businesses to address issues proactively rather than waiting for formal opposition.
This approach also encourages collaboration across different jurisdictions. GNNs can easily integrate and analyze trademark data from various countries, promoting a more unified understanding of trademark similarities and potential conflicts. This stands in contrast to older approaches which might rely heavily on isolated features. By merging different types of data, like user behavior and market trends, GNNs better reflect the connected nature of the modern trademark landscape.
The application of GNNs to trademark opposition analysis has spurred a new wave of insights for legal professionals. These professionals are starting to uncover novel patterns and correlations within their data, challenging previously held assumptions about trademark uniqueness and potential overlap. This in turn is driving a reconsideration of traditional opposition strategies.
As GNNs continue to develop and become more refined, they promise to revolutionize the way we approach trademark analysis. Their ability to learn from complex relationships, incorporate various data sources, and adapt to change offers a powerful tool for legal professionals seeking to protect their clients' intellectual property in the face of a rapidly evolving business environment. There is still some debate about the best ways to apply them, but this remains a very intriguing and relevant field of research within AI and trademark law.
7 Critical Problem-Solving Techniques That Shaped Notable AI Trademark Applications in 2024 - Computer Vision Algorithms Enhance Design Mark Comparisons at Singapore IP Office
The Singapore Intellectual Property Office is leveraging computer vision algorithms to significantly enhance the way design mark comparisons are conducted. These algorithms, particularly those powered by deep learning, excel at automatically extracting and analyzing visual features within design marks. This automation improves the efficiency and accuracy of trademark evaluations. Optimization techniques play a vital role in ensuring these algorithms operate effectively, influencing both processing speed and the precision of the results.
There are also newer algorithms emerging, such as "FeatUp", that aim to overcome some of the limitations found in traditional computer vision systems by capturing both the broad strokes and fine details within images. This approach has implications for how detailed and nuanced design mark comparisons can be made.
While the integration of computer vision into AI is revolutionizing how trademarks are assessed, particularly for visual marks, it also highlights questions surrounding the reliability and trustworthiness of these AI-driven systems in complex legal scenarios. The ability of these algorithms to consistently and fairly interpret complex design elements within a rapidly evolving intellectual property landscape remains a vital consideration. Ultimately, the application of computer vision techniques in this context signifies a critical step forward in the field of trademark protection.
7 Critical Problem-Solving Techniques That Shaped Notable AI Trademark Applications in 2024 - Federated Learning Networks Connect International Trademark Databases
Federated Learning (FL) is a relatively new approach to linking international trademark databases. The key feature of FL is that it allows for collaboration on AI models without requiring individual trademark offices to share their own raw data. This addresses a long-standing problem: trademark data is often kept in separate, isolated databases, known as "data silos." This is a major concern in the trademark world, as intellectual property (IP) is highly sensitive and needs to be protected. Each country, or even each trademark office, has its own unique rules and procedures, and sharing trademark data across borders can create legal and logistical issues.
FL seeks to solve this by enabling AI models to be trained across multiple locations, like a network. Each location (an IP office, for instance) contributes to the training, but doesn't have to hand over their actual data to others. This is important because trademark databases often contain confidential information about specific businesses.
The appeal of FL is that it has the potential to create a stronger, more integrated framework for assessing trademarks internationally. Ideally, this would improve global trademark enforcement and protection while also respecting the privacy of the trademark data held by each individual jurisdiction.
However, there are also some notable challenges in applying this technique. It's difficult to ensure that all the local models being trained in different locations end up seamlessly combined into one global model. It will take time to work through practical issues and potential scaling problems that this approach presents. Despite these difficulties, FL remains a compelling concept with the potential to improve how international trademark systems function and evolve in the years to come.
Federated learning (FL) offers a way for international trademark databases to cooperate without compromising data privacy. This distributed machine learning method allows multiple servers, like those used by different trademark offices around the world, to contribute model updates without actually sharing their raw trademark data. This addresses a major hurdle in global trademark efforts: protecting sensitive information while still enabling valuable cross-border collaboration.
The push to protect data in machine learning has made FL a compelling alternative to the more traditional way of handling data, where it's all consolidated in one place. FL excels when it comes to training AI models on data that's spread across multiple locations, and the whole process ensures the data stays put. It maintains the confidentiality of local trademark datasets, making it ideal for applications where data privacy is paramount.
FL's effectiveness hinges on its ability to efficiently combine the model updates from all the participating parties. This allows for a unified model that represents the collective knowledge of everyone involved. Some large companies are already using FL in situations where training data is dispersed geographically, emphasizing its value in privacy-conscious applications.
FL's key strength is its ability to break down data silos. This means that various trademark organizations can work together to create a more insightful model without having to give up any control over their trademark data. Recent research has highlighted different parts of FL, including the technical components, how it can be used on mobile networks, and its vulnerabilities to security attacks.
FL's main approach involves a secure aggregation process that strengthens the privacy of the collaborative training process. This has drawn the attention of researchers looking into how to change data practices in areas where information needs to be highly confidential. Though it's still early, it shows real promise for reshaping how trademark offices globally handle data for AI applications. There are challenges in how well the different offices and organizations can communicate and how the data should be structured, but the value proposition for promoting international understanding of trademark patterns is quite attractive. Perhaps by exploring global trademark trends through the aggregate model outputs, we can get insights that could influence industry standards and regulatory measures in ways that protect intellectual property better and allow global brands to operate more smoothly.
7 Critical Problem-Solving Techniques That Shaped Notable AI Trademark Applications in 2024 - Deep Learning Models Map Complex Trademark Translation Equivalents
Deep learning models are proving valuable for establishing accurate connections between trademarks and their translations in different languages. These models excel at deciphering intricate meaning across language barriers, a challenge often faced in trademark assessment. They use large datasets and sophisticated algorithms to better understand the nuances of language and context, which are vital for maintaining intellectual property rights. While promising for decision-making, the use of these models raises concerns regarding transparency and reliability within legal settings. It's crucial to continue evaluating their performance and ensuring their applications in trademark law are both helpful and consistent. The integration of these models into trademark translation signifies a significant step in how we approach complex language and legal issues in the field.
Deep learning approaches have significantly impacted the field of trademark translation, particularly for languages with complex character sets. These models are built with architectures specifically designed to capture the intricate relationships between characters, which greatly improves the accuracy of translating trademarks across languages with vastly different scripts. It's quite fascinating how these models can even achieve translation equivalence when dealing with trademarks that are visually similar but have distinct contextual meanings. This indicates that they're able to integrate a semantic understanding of the trademarks along with their visual recognition.
The success of deep learning in this area relies heavily on the use of large, carefully labeled datasets. These datasets allow the models to learn to recognize not just individual characters, but also culturally specific details that influence how a trademark is perceived. It seems that capturing these subtle nuances is critical for effective translation. Recent developments include the use of self-attention mechanisms, which let the model focus on specific parts of the trademark image while also considering the wider context of related datasets. This targeted approach significantly boosts recognition rates and, consequently, translation accuracy.
Another intriguing development is the use of transfer learning. In this technique, a model initially trained on a vast body of general image data can be fine-tuned to work specifically on trademark translation. This is beneficial because it minimizes the need to create and gather extensive, trademark-specific datasets. These deep learning models also have a continuous learning ability, which allows them to update translations as new trademarks are registered. This continuous updating ensures that the translations remain current and accurate in real time.
Beyond simple character translation, these deep learning models are capable of more contextual translations. They don't just focus on the linguistic aspects of a trademark but also consider the emotional and psychological impact it might have on different audiences. It's interesting that they can even analyze and interpret the impact of design elements, like shapes, colors, and logos, which are vital to a trademark's identity and how consumers perceive it. Adversarial training techniques have also been incorporated to help the models challenge their own interpretations, leading to more robust and dependable translation outputs. This is an important step towards minimizing errors, particularly when dealing with trademarks that are open to interpretation.
These advancements in trademark translation raise some interesting possibilities for the future of trademark law. The improved accuracy of machine translation for trademarks could lead to more automated processes within the trademark evaluation system. This naturally leads to questions about the role of human oversight in trademark evaluations, suggesting a potential shift in how these legal processes are handled. It's a dynamic area of research, highlighting the interplay between AI and complex legal frameworks.
More Posts from aitrademarkreview.com: