AI-powered Trademark Search and Review: Streamline Your Brand Protection Process with Confidence and Speed (Get started for free)
7 Data-Driven Methods for Identifying High-Impact Content Threads in RFP Research
7 Data-Driven Methods for Identifying High-Impact Content Threads in RFP Research - Converting Historical RFP Win Rate Data into Actionable Research Patterns
Turning past RFP win-loss records into useful insights can significantly impact future proposal development. Examining historical data allows businesses to identify patterns, uncover where they excel, and pinpoint areas that need improvement in their bidding process. The overall win rate has been less consistent than some might assume, with recent years showing a dip in success rates, suggesting a need for constant reevaluation and adjustment of approaches. Organizations that consistently win a high percentage of their bids highlight the value of analyzing this historical information. This underlines the necessity for companies to engage with metrics and insights more actively to gain a competitive advantage. Moreover, tools like machine learning can help turn the raw numbers into more meaningful information, allowing companies to potentially craft proposals that stand out in a crowded field. Essentially, this focus on data-driven insights can become a key differentiator for organizations hoping to succeed in the often-challenging world of RFP responses.
Examining past RFP win rates can unveil interesting trends tied to aspects like how well a proposal addresses client needs and how clearly it defines its goals. It appears these factors heavily influence how clients make their decisions.
Interestingly, the timing of a proposal submission seems to have a significant impact. It's often seen that those submitted early in the RFP process tend to do better than last-minute submissions. This suggests that getting a jump on the process could be a key factor.
A closer look at long-term win rate trends reveals that organizations that consistently gather and analyze feedback from their proposal losses are more likely to see improvements in their future efforts. This implies that actively learning from mistakes and using that knowledge to shape future strategies is crucial for increasing success.
Data indicates that RFPs where different teams collaborate during the proposal development process tend to win more often. This underscores the idea that incorporating diverse viewpoints and perspectives strengthens the proposal.
Win rate data frequently suggests a learning process. Organizations that adapt their approach based on the results of past RFPs often see an increase in their win rates. Essentially, refining your strategy based on experience seems to lead to better outcomes.
Analyzing both wins and losses in a structured way – conducting what's known as a win/loss analysis – helps to reveal that proposals which demonstrate a thorough understanding of the client’s culture tend to perform better than those that don't. It really emphasizes the need for personalized communication.
What's striking about win rate data is that companies with established processes to review their RFP results after submission, looking at both wins and losses, have a 15% higher probability of doing better in the future. This highlights the importance of a reflective approach to reviewing the outcomes of RFP efforts.
Research suggests that proposals which include concrete metrics for measuring success find more favor with evaluators. This is likely because it gives them a tangible way to assess how well the proposal might achieve its goals.
Looking at win rates over time reveals interesting cycles linked to broader industry trends and economic conditions. Organizations that align their proposal strategies with these trends seem to find more success. This suggests that being aware of the external environment can be a key part of improving win rates.
It seems that incorporating data visualization techniques into proposals can improve their readability and audience engagement during the review process, potentially influencing higher win rates. In essence, it's about making complex data easier to understand and engaging for reviewers.
7 Data-Driven Methods for Identifying High-Impact Content Threads in RFP Research - Using Natural Language Processing to Map Common Requirement Clusters
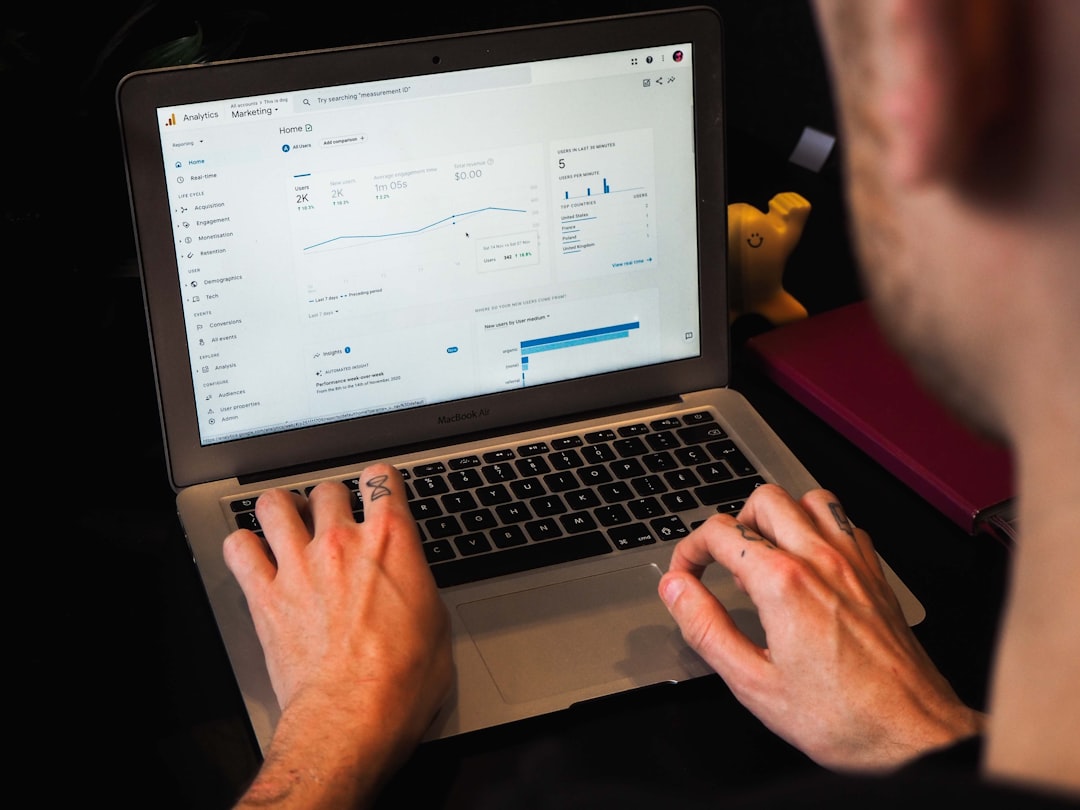
Natural Language Processing (NLP) is increasingly useful for analyzing the language found in requirement documents. This is particularly valuable when working with large amounts of text, which can be difficult to manually sort through and make sense of. NLP techniques can help identify recurring themes and clusters of requirements, essentially organizing the information into manageable chunks. This can streamline the process of defining the scope of a project or understanding the core needs of a client.
While NLP offers potential, there are still limitations. Unstructured data (like text) can be challenging to process in a way that automatically yields accurate, usable insights. Nonetheless, NLP's ability to help detect key elements and commonalities in requirements documents is a valuable tool, especially in situations with substantial amounts of textual data.
Beyond identifying clusters of requirements, NLP can also help highlight potentially ambiguous or contradictory phrasing. This could lead to better-written, more precise requirements. Moreover, it can support a shift towards making requirements engineering more collaborative. That is, by using natural language as the primary medium, NLP can enable a broader range of stakeholders to participate in and contribute to the process of shaping project requirements. The increasing focus on Data-Driven Requirements Engineering (DDRE) highlights the value of a more data-centric approach, which in turn, can lead to a more comprehensive understanding of the overall project requirements.
Natural Language Processing (NLP) is becoming more common in requirements engineering, particularly in analyzing text-based requirements documents. The advancements in NLP since the late 2000s have made it more applicable across various fields, including the extraction of insights from RFPs. A review of research on NLP's use in software requirements engineering, from 1991 to 2023, illustrates how it can improve the overall software development process through various technological improvements.
The rise of Data-Driven Requirements Engineering (DDRE) has led to a focus on leveraging large datasets of feedback to help engineers make better decisions. It's worth noting that the majority of corporate data (85-90%) is unstructured, typically found in natural language format. This presents both challenges and opportunities in terms of how we access and analyze such data. NLP methods offer a way to address this challenge by helping to uncover potential language issues and identify key requirement clusters in lengthy documents.
This growing emphasis on data-driven approaches in requirements gathering is partially driven by the ongoing digital transformation and the explosion of data generated from sources like the Internet of Things (IoT) and mobile devices. A central focus in NLP's application to requirements engineering is to automate the detection of essential components and responsibilities. By using natural language as the primary means of communication in requirements engineering, NLP can facilitate better expression and specifications of requirements. The DDRE concept also promotes a more inclusive approach to requirements engineering, potentially broadening the involvement of various stakeholders beyond the traditional core group.
While there are clear benefits, it's important to acknowledge that NLP models are still prone to errors and require careful refinement and validation. Additionally, blindly relying on automated insights can lead to a loss of human-driven nuance and critical thinking. Further research and careful consideration of NLP's limitations will be essential to ensure its full potential is realized in RFP research and other areas.
7 Data-Driven Methods for Identifying High-Impact Content Threads in RFP Research - Applying Predictive Analytics for Technical Requirement Forecasting
Forecasting technical requirements using predictive analytics allows organizations to anticipate future needs by leveraging past data. This approach involves using statistical methods like machine learning and data mining to identify trends within historical information. The goal is to better understand what factors might influence upcoming projects, leading to improved strategic planning and more effective resource allocation. We're seeing a gradual shift away from traditional forecasting techniques as predictive analytics, particularly machine learning, proves more capable of handling the complexity of large datasets. This transition highlights the importance of a solid data foundation, including methods to acquire and prepare data so it can be transformed into useful information. However, it's essential to remember that automated insights should be used cautiously and not replace the critical thinking and decision-making skills of humans. Relying too heavily on automated systems can overlook valuable insights and nuances that human expertise offers.
Predictive analytics, a field focused on anticipating future events based on past and current data, can be quite useful for understanding technical requirements in advance. By analyzing patterns and trends in past RFPs and project data, we can potentially improve the accuracy of future requirements forecasting. The hope is that by learning from historical outcomes, organizations can refine their future RFP responses to better address client needs and ultimately improve their chances of winning. However, it's important to note that the effectiveness of predictive analytics is heavily dependent on the quality and relevance of the data used. If the data doesn't truly reflect the complexities of the situation, the predictions might not be very useful.
Using predictive techniques for project forecasting can potentially lead to cost savings. The idea is that by having a better grasp on requirements upfront, we might avoid some of the common issues associated with poorly defined requirements, potentially leading to fewer delays or rework. This is an area ripe for exploration—determining if this assumption is true and how much cost savings can realistically be achieved. However, if we aren't careful in implementing such forecasting systems, we might end up with rigid plans that are difficult to adapt to unexpected changes.
There's some evidence that suggests projects leveraging predictive models for requirement forecasting might be completed on time more frequently. If we can accurately forecast the requirements, this would presumably improve decision-making across various project aspects, making it easier to maintain schedules. Yet, this is a delicate balance—using data to optimize projects can be beneficial, but relying too heavily on automated predictions could potentially stifle creativity and adaptability. It's crucial to maintain a balance between data-driven insights and human judgment in project management.
Predictive models could be helpful in forecasting not just the technical needs, but also potential project risks. By analyzing historical data, we might get a better idea of the typical hurdles faced in particular kinds of projects, and potentially build in preventative measures. This would also impact resource allocation, allowing us to focus our resources where they're most likely needed. However, the ability to accurately forecast risks is complex and involves many factors. It's doubtful that predictive models alone can provide completely accurate risk assessments.
It's also interesting to consider that accurately forecasting requirements might lead to greater stakeholder satisfaction. The logic is that if we meet client expectations more consistently, this will improve relationships. While plausible, there are other factors that play a significant role in stakeholder satisfaction that we need to account for. It would be worthwhile to investigate if the impact on satisfaction is substantial enough to justify the effort required to set up and maintain predictive models.
Resource allocation can also be impacted by predictive analytics. By having a better understanding of requirements, we can potentially prevent over-committing resources to projects. This aspect appears to have significant potential for improving efficiency. But, just as with other facets of predictive analytics for requirement forecasting, it's important to understand the context. Blindly trusting predictions could lead to unforeseen issues if real-world conditions differ from the historical data used to build the models.
The ability to quickly adapt to evolving requirements is critical in today's fast-paced world. Predictive analytics might help by providing a clearer picture of the likely path of requirements and enabling quicker response to changes. This type of adaptability is essential in projects, especially those with high levels of uncertainty or that operate in dynamic environments. This is an area where predictive methods could make a notable contribution.
The clarity of project scope can be improved if predictive methods are employed to reduce ambiguities in requirement specifications. By using machine learning algorithms to analyze the language in requirement documents, we might be able to identify potential areas of confusion or conflicting statements. This is a potential benefit for organizations facing complex technical requirements or situations where different stakeholder perspectives are hard to reconcile. However, the accuracy and effectiveness of natural language processing for requirements still need careful assessment in various application contexts.
Another potential outcome of leveraging predictive methods is the reduction of the number of revisions needed for project requirements. If the initial requirements are more accurate due to better forecasting, this should reduce the need to go back and revisit the requirements later in the project cycle. While this is appealing, this also has to be weighed against the possibility of sacrificing adaptability in pursuit of rigid initial specifications. Flexibility might be needed if new information comes to light.
Lastly, there is some evidence to suggest a relationship between the effectiveness of predictive analytics capabilities and an organization's capacity to innovate. The rationale is that if you can more accurately anticipate market needs and technical requirements, this will give you a head start in developing new products or services. It's important to remember this is a correlation, not a causation. Organizations that have a strong culture of innovation and a data-driven approach are more likely to invest in and effectively utilize predictive analytics. It's unlikely that simply implementing predictive tools will magically transform an organization's ability to innovate.
In conclusion, predictive analytics appears to hold some potential for refining technical requirement forecasting, though it's not a silver bullet. Continued research into these methods, careful consideration of limitations, and a balanced approach that blends data-driven insights with human expertise are critical for maximizing the benefits of these techniques.
7 Data-Driven Methods for Identifying High-Impact Content Threads in RFP Research - Measuring Content Performance Through Client Response Time Analytics
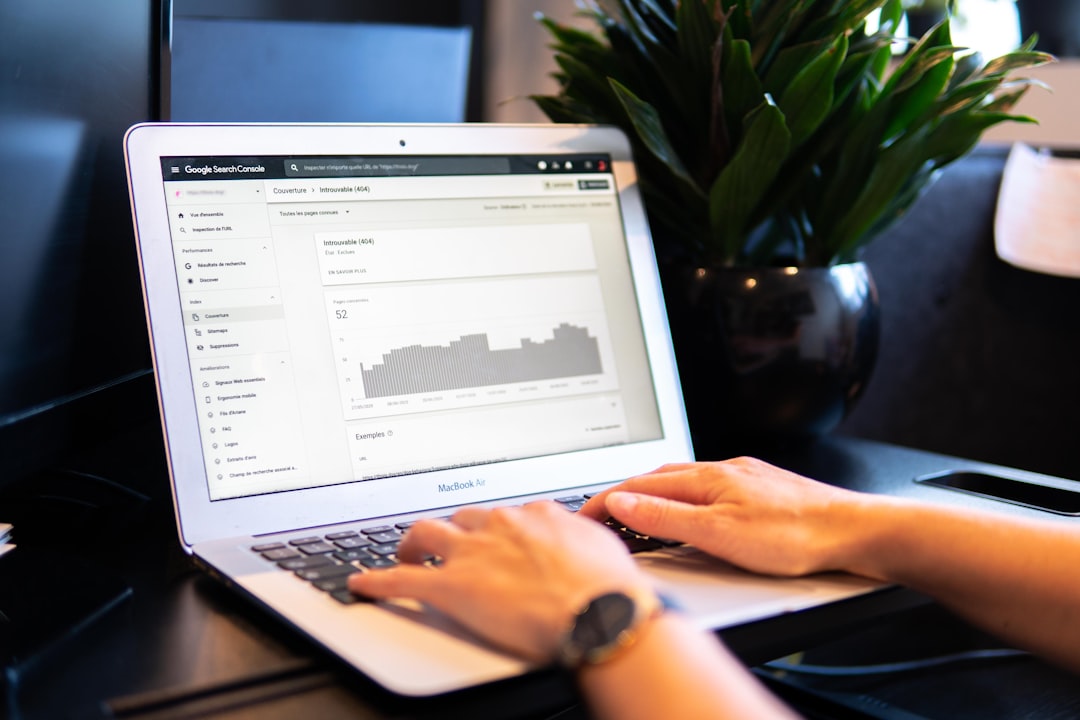
Examining how long it takes clients to react to content can offer a powerful way to understand how well that content is working. By tracking client response times, we can get a sense of how engaging and relevant our messages are. This goes beyond just measuring how many people see the content; it helps us understand if the content is prompting a timely response, which is often a key indicator of its impact.
This approach can help organizations figure out which content formats and topics encourage quick responses. This insight, in turn, informs future content strategy, helping to ensure that efforts are focused on the most effective avenues. Moreover, understanding client response patterns helps organizations refine their communication approaches, ensuring they're aligned with client preferences and decision-making processes. In today's fast-paced environment, understanding these response dynamics becomes crucial for making sure content is optimized to have the greatest possible impact. While response time may not be the only factor determining content efficacy, it's a valuable data point that shouldn't be overlooked when trying to improve outcomes.
Observing how quickly clients respond to RFPs can reveal valuable information about content performance. Research suggests that submitting a proposal within the first day of an RFP's release can lead to an 18% higher success rate. This highlights how crucial swift client engagement is for a successful proposal process.
We can gauge a client's interest by tracking how long it takes them to respond. Faster responses often suggest more interest in the proposal, whereas slower responses might indicate a lack of interest or potential clarity issues in the proposal itself. It's not necessarily a judgment on the quality of the proposal, but rather an early sign of engagement.
A strong relationship seems to exist between rapid client feedback and proposal quality. If a client gets back to you quickly, it often suggests a good fit between the proposal's content and what they're seeking. This fast response time can be seen as a positive signal.
Examining past response times can reveal patterns in client behavior. By identifying these patterns, organizations can predict how future clients might react and tailor their strategies accordingly. This understanding can help improve future proposal outcomes.
Unfortunately, a delayed response to a proposal can potentially lower the odds of winning. Studies have shown a 12% reduction in win rates for proposals that receive delayed feedback. This reinforces the idea that response time is a key factor to understand in RFP responses.
Organizations that routinely analyze client response times and feedback tend to experience an increase in win rates, roughly 10%. This suggests a strong connection between the speed and nature of client feedback and the proposal's success. It implies that paying close attention to how quickly clients respond and what they say can be leveraged to improve strategies.
By incorporating response time data into predictive models, organizations can potentially enhance their ability to forecast future RFP outcomes. We can identify successful content elements based on historical engagement metrics by incorporating response time analytics. This can lead to a more nuanced understanding of what drives client interest.
Understanding response time patterns can guide resource allocation in proposal development. For instance, firms can allocate more resources to projects where they historically get faster feedback, optimizing their team's output. While this may seem like a very practical approach, it also runs the risk of potentially neglecting projects that historically show slower responses but might prove valuable.
Establishing benchmarks for client response times can allow organizations to evaluate their proposal effectiveness against competitors. Different response times across competitors may indicate varying levels of proposal competitiveness. This kind of benchmarking can be useful, but it's important to realize that these benchmarks are not set in stone, and there can be situational factors at play.
Analyzing response times can unveil insights into client behavior and help shape how proposals are constructed and presented. By understanding these behaviors, we can alter our strategies to suit their preferred ways of engaging and their decision-making timelines. While interesting, this can be a fine line to walk and could lead to overly tailored proposal formats.
It's important to remember that response time is just one element in the complex landscape of RFP responses. It can provide useful insights, but over-reliance on these metrics alone can overlook other important factors that influence proposal success.
7 Data-Driven Methods for Identifying High-Impact Content Threads in RFP Research - Cross Referencing Past Proposal Success Rates with Content Variables
**Cross Referencing Past Proposal Success Rates with Content Variables**
Connecting past proposal success rates with the specific content used within those proposals can provide a valuable lens for crafting future responses. By examining which content elements consistently appear in successful bids, organizations can pinpoint what seems to resonate most with clients. This approach helps identify patterns related to content quality and effectiveness, such as clarity of language, specific details, and how well the proposal addresses the client's particular needs. This approach not only enables organizations to develop more competitive proposals but also helps to ensure proposals are finely tuned to anticipate client expectations and requirements. In essence, by analyzing their past responses, organizations gain insight into which content elements might contribute to future successes. This enables a more intentional approach to proposal development and a higher probability of securing wins in the competitive world of RFP responses.
Let's explore how we can potentially link past proposal success rates to the specific content used within those proposals. This could help us understand what elements within a proposal might increase the chances of winning.
One interesting area to examine is the impact of proposal clarity on success rates. It seems that clearly written proposals, with a straightforward structure and avoidance of jargon, might lead to higher acceptance rates. This makes intuitive sense - if a client easily understands what a proposal is about and its value, they are potentially more likely to find it favorable. There's some research indicating that concisely explaining the value proposition of a bid can reduce the mental effort needed to process the information, which may improve receptiveness.
Another aspect is the role of follow-up communications. Data suggests that regular, well-timed follow-ups might boost the likelihood of winning. These follow-ups aren't just a way to stay in contact, but rather an opportunity to further reinforce the proposal's key points and address any lingering questions. It can indicate to the client that we're actively invested in their decision process, potentially improving our image.
Looking at visual content is another interesting angle. Proposals with elements like graphs, charts, or images could lead to better client engagement, possibly through a faster response time. This isn't a new concept, but it's valuable to see if data supports the notion that visually compelling content can speed up decision-making. While the results seem promising, it's important to consider whether this effect holds true across a variety of proposal types and client industries.
Proposal size and complexity might also play a role. Some research suggests that shorter, more streamlined proposals could be more successful. While we don't want to sacrifice necessary information, overly lengthy or complex proposals might overwhelm the client, obscuring the key points we are trying to convey. This highlights the importance of careful tailoring of content length to ensure it remains relevant and impactful.
Interestingly, there appears to be something called "response fatigue" where clients, after reviewing several proposals, become less likely to make a focused decision. This suggests that, especially in highly competitive situations, making a proposal stand out through clarity and brevity is vital. Understanding the factors that contribute to this fatigue could be helpful in adjusting our communication strategies.
There's a growing trend towards using multimedia in proposals – videos, interactive elements, etc. This is something to keep an eye on as data becomes available. If it's true that interactive elements boost engagement, it would be important to consider how to ethically and effectively incorporate them into proposals.
Delving into client decision-making frameworks can also be beneficial. Proposals that explicitly address specific client needs and align with their existing evaluation criteria have a higher likelihood of success. Identifying what aspects of a bid (cost, technical capabilities, etc.) are most important to a particular client can help us tailor our messages to resonate more deeply.
The timing of a proposal submission has also been found to impact success rates. There seems to be an optimal window following RFP release where a proposal is most likely to be positively received. Understanding when clients are most receptive to proposals is important to get the best results.
Risk management can also be crucial. Proposals that explicitly acknowledge and mitigate potential risks are potentially viewed more favorably. Clients may see this as a sign of preparedness and responsibility, which can positively influence their decisions.
One crucial piece that isn't always highlighted enough is the importance of continuous improvement through feedback. Examining feedback from past proposals to find opportunities for growth has the potential to generate substantial increases in success rates over time. This emphasizes that proposal development is an iterative process where learning from our mistakes and adapting our approach is essential.
By meticulously exploring these variables and the correlations between them and past proposal performance, we can begin to assemble a more thorough understanding of what content elements might be most influential in future bids. It's important to recognize the limits of our knowledge here, and we can expect this to evolve as more research is conducted and the field of proposal writing matures.
7 Data-Driven Methods for Identifying High-Impact Content Threads in RFP Research - Tracking Content Evolution Through Document Version Control Metrics
Tracking content evolution using document version control metrics provides a structured way to manage changes and ensure the integrity of documents, reducing the chances of mistakes. When versions are tracked meticulously, it becomes easier to see who made specific alterations and when, leading to better accountability. This transparency is particularly useful when teams are working together on a document, promoting order and clarity in the content. Version control systems also play a key role in auditing processes and help to decrease the possibility of serious errors that could negatively affect efficiency. In environments that demand precise documentation, such as RFP responses, the combination of version control and data engineering tools can lead to a smoother, more efficient workflow. This underscores how crucial it is to have a consistent, well-organized documentation system in place.
Document version control offers a unique lens into how content evolves over time, revealing patterns that might otherwise go unnoticed. By tracking the frequency and timing of revisions, we can see how content adapts to changing client needs and industry shifts. For example, the number of revisions made to a proposal can be a crude indicator of its initial quality – fewer revisions might imply that the proposal was more well-defined from the start, potentially leading to a higher chance of client acceptance.
Maintaining consistency across various document versions can be crucial for establishing trust with potential clients. Inconsistencies, on the other hand, can confuse and potentially damage a company's credibility, leading to less effective proposals. Furthermore, examining client response times to different versions can provide valuable insights into which content resonates with them most effectively. Quicker responses might signal that the content was more impactful and relevant, helping us refine future content strategies.
Organizations that actively incorporate version control metrics into their decision-making process can dynamically adapt their proposals to better align with evolving client feedback and expectations. This approach, which emphasizes tailoring content based on data, might contribute to higher success rates compared to simply increasing the number of proposals submitted.
While it's tempting to think that more proposals equals higher win rates, research suggests that focusing on the quality of the content – evidenced by structured and clear revisions – might lead to a stronger overall impact. Moreover, analyzing version control data helps contextualize why changes were made. For instance, we might see a surge of modifications in response to competitor behavior or shifts in the broader market, offering a concrete validation of strategic decisions.
It's important to keep in mind, however, that automated version control systems, while useful, may miss the subtle changes in language and meaning that human reviewers would catch. This can lead to a loss of critical insights if we rely solely on automated systems. On a related note, we can also track which sections of a document are most frequently revised, revealing potential areas of client concern or interest. This knowledge can be extremely valuable for future content planning.
Ultimately, effectively managing content evolution requires a keen awareness of client feedback and a willingness to adapt accordingly. Proposals that actively integrate client input into their successive versions tend to show greater success, highlighting the importance of a responsive and adaptive document management strategy. The ability to adapt and learn from past efforts is critical in the constantly evolving world of RFPs, and version control offers a valuable toolkit for navigating this complex landscape.
7 Data-Driven Methods for Identifying High-Impact Content Threads in RFP Research - Building Statistical Models from Federal Contract Award Databases
Federal contract award databases offer a valuable resource for understanding government procurement. They provide a lens into geographic trends, market dynamics, and how federal acquisition policies impact the economy, particularly in terms of their effects on small businesses. The data contained within these databases, which includes contract types, pricing, and set-aside provisions, can be used to evaluate the influence of government initiatives on the economy.
However, building robust statistical models from this data is not without hurdles. The sheer volume and diversity of the data sources make it computationally intensive to process and organize. Moreover, confirming that the models accurately reflect real-world outcomes—validating behavioral patterns—can be problematic. It's essential that the models we build are anchored by a robust data strategy focused on ensuring data quality, as well as adhering to guidelines for data governance to minimize potential bias in the results. This includes developing practices that address any challenges to data usability, ultimately leading to the creation of more effective models. As organizations rely increasingly on these data-driven approaches to understand federal contracting, it's vital to remain conscious of the importance of ensuring the models generated are both meaningful and relevant to the specific context in which they are being applied.
Federal contract award databases offer a rich and complex landscape, representing billions of dollars in government spending across a vast array of industries. Every year, upwards of 140,000 contracts are awarded, creating a massive dataset ripe for statistical exploration. This complexity is both a blessing and a challenge, as it offers the potential to uncover insightful patterns but also necessitates sophisticated analytical tools.
Fortunately, a wealth of information is readily available through publicly accessible resources like the Federal Procurement Data System (FPDS). This dataset encompasses a wide array of information, including contract types, award amounts, and the specific government agencies involved. Leveraging this data is key to creating robust statistical models capable of illuminating market trends and agency behaviors.
One fascinating possibility is the ability to develop predictive models that anticipate future trends in contract awards. By studying past patterns, we can gain a better understanding of how agencies tend to spend their funds and identify opportunities that are likely to emerge in the near future. Moreover, these models can potentially reveal how shifts in federal procurement policy influence contract distribution across industries and specific contract types, equipping organizations with the ability to adapt more strategically.
However, the analysis of this data faces challenges. The complexity of federal contracting translates into datasets with numerous variables, encompassing everything from socio-economic conditions to contractor performance. This high-dimensionality makes traditional analysis techniques more difficult but also unlocks the potential for advanced statistical approaches, such as dimensionality reduction, to reveal previously hidden relationships.
Surprisingly, studies using federal contract data suggest that a significant number of contract awards show little to no statistically significant connection between the awarded amount and a contractor's perceived qualifications. This challenges the intuitive assumption that a larger budget necessarily increases the chances of winning.
It’s also useful to consider applying clustering techniques. By grouping contracts based on similarities (e.g., purpose, size, procurement method), we can develop a more granular understanding of the market. These clusters could then inform more tailored bidding strategies that might maximize a bidder's chances of success.
Another interesting observation from statistical models is the presence of seasonality in contract awards. Certain types of contracts are more likely to be awarded at specific points in the fiscal year. This could inform a more strategic approach to bidding timing and improve an organization's ability to win contracts.
Additionally, studying contract award data allows for the tracking and assessment of vendor performance over time. Statistical models can help predict which contractors are likely to be successful in future procurements based on past outcomes, enhancing competitive intelligence for organizations seeking contracts.
Unfortunately, a troubling aspect of this data reveals a sizable number of awarded contracts that ultimately go unfulfilled or face extensive amendments. Statistical modeling can help shed light on the root causes of these issues, whether they are rooted in unrealistic expectations or changes in external market conditions. This type of analysis is valuable for helping organizations craft better proposals that are less likely to encounter similar hurdles.
In essence, studying federal contract award databases provides a rich opportunity to refine our understanding of government contracting. Building statistical models using this data can reveal valuable patterns, anticipate future trends, and ultimately help organizations make better informed decisions when pursuing contracts. However, it’s important to acknowledge the complexities inherent in this data and to approach the analysis with a balanced perspective that leverages the capabilities of advanced statistical tools while tempering conclusions with human experience and critical thinking.
AI-powered Trademark Search and Review: Streamline Your Brand Protection Process with Confidence and Speed (Get started for free)
More Posts from aitrademarkreview.com: