AI-powered Trademark Search and Review: Streamline Your Brand Protection Process with Confidence and Speed (Get started for free)
7 Metrics for Assessing AI-Generated Blog Content Quality in 2024
7 Metrics for Assessing AI-Generated Blog Content Quality in 2024 - Semantic Coherence Measurement Using Natural Language Processing
Within the realm of AI-generated content, evaluating the logical flow and overall meaning—what we call semantic coherence—is paramount, especially for blog posts. Natural Language Processing (NLP) offers various tools to dissect this aspect.
Metrics like topic coherence, a key aspect of NLP, help gauge how well the content stays on track, a vital consideration in both human- and AI-driven writing. Understanding perplexity, a measure of how easily a language model can predict the next word, provides another window into the coherence of the generated text. Basically, if the text is easy to understand and follow, perplexity scores would be lower.
Techniques like semantic compression help to improve the clarity and structure of content by simplifying complex language, effectively improving the content’s overall coherence. Additionally, a tool like semantic entropy can act as a guard against "hallucinations"—situations where the AI fabricates information or loses track of its narrative.
Ultimately, a multi-faceted approach involving a combination of metrics is likely the best way to evaluate coherence. This helps create a more complete picture of the AI's performance. As AI models are further refined and adopted, rigorously assessing semantic coherence becomes increasingly critical to understanding their capabilities and limitations—especially as these models move beyond simple content generation and tackle more complex topics like clinical or scientific writing.
1. Evaluating the semantic coherence of AI-generated blog content involves utilizing natural language processing (NLP) techniques to assess how well the text holds together conceptually. This often relies on vector space models, which map words and phrases into a multi-dimensional space, allowing us to see how similar their meanings are in context. This gives us a more detailed way to judge if the blog stays focused on its main topic throughout.
2. Recent advances in NLP, particularly with models like BERT and GPT, have enabled us to quantify semantic coherence by examining how word embeddings are distributed. Essentially, we can see how well different parts of the text align with each other in terms of their underlying meanings. This offers a more insightful approach to understanding the text's overall structure.
3. Human readers, it turns out, rely on both the grammatical structure and the flow of ideas when assessing coherence. This presents a significant obstacle for AI systems, which tend to depend mainly on statistical connections without truly understanding the concepts. It's a reminder that the quest for genuinely coherent AI-generated content is complex.
4. Graph-based methods for analyzing semantic coherence offer a way to map out the connections between different ideas in the text. By visualizing these relationships, we can potentially uncover inconsistencies in how well each part of the blog relates to the central theme. This helps expose areas where the content may be straying off topic.
5. Training AI models with datasets that contain feedback from human readers has been shown to improve their semantic coherence scores. This observation reinforces the importance of human judgment in defining "quality" content. It seems that what people perceive as good writing is crucial in guiding AI development towards higher quality outcomes.
6. Interestingly, measuring semantic coherence can be linked to how readers interact with a blog, such as the amount of time they spend on it or their tendency to leave quickly. This opens the possibility of using coherence metrics to predict how well content will perform in real-world scenarios. The connection between coherence and engagement is worth further exploration.
7. Researchers are now developing techniques that simultaneously optimize for semantic coherence and readability. This is a promising direction, as it could lead to AI systems capable of producing content that is both conceptually sound and easy to understand. The goal is to create AI content that is both meaningful and accessible to readers.
8. While traditional grammar-focused metrics can sometimes miss the bigger picture of how ideas connect, incorporating semantic coherence measures into AI training can help them understand content effectiveness more comprehensively. It's about moving beyond the surface-level characteristics and understanding the underlying meaning of the text.
9. One notable hurdle is the subjectivity of human judgment when it comes to coherence. What one person finds clear and easy to follow might seem jumbled to another. This makes it hard to create universal standards for coherence evaluation. Developing metrics that are robust and reliable despite these individual differences is a challenge.
10. The dynamic nature of language, including the constant emergence of new slang and idioms, presents a unique challenge for semantic coherence models. They need to adapt and evolve along with the language they analyze to remain effective. Keeping pace with linguistic change is a continuous task for developers in this area.
7 Metrics for Assessing AI-Generated Blog Content Quality in 2024 - Engagement Metrics Analysis Through User Interaction Data
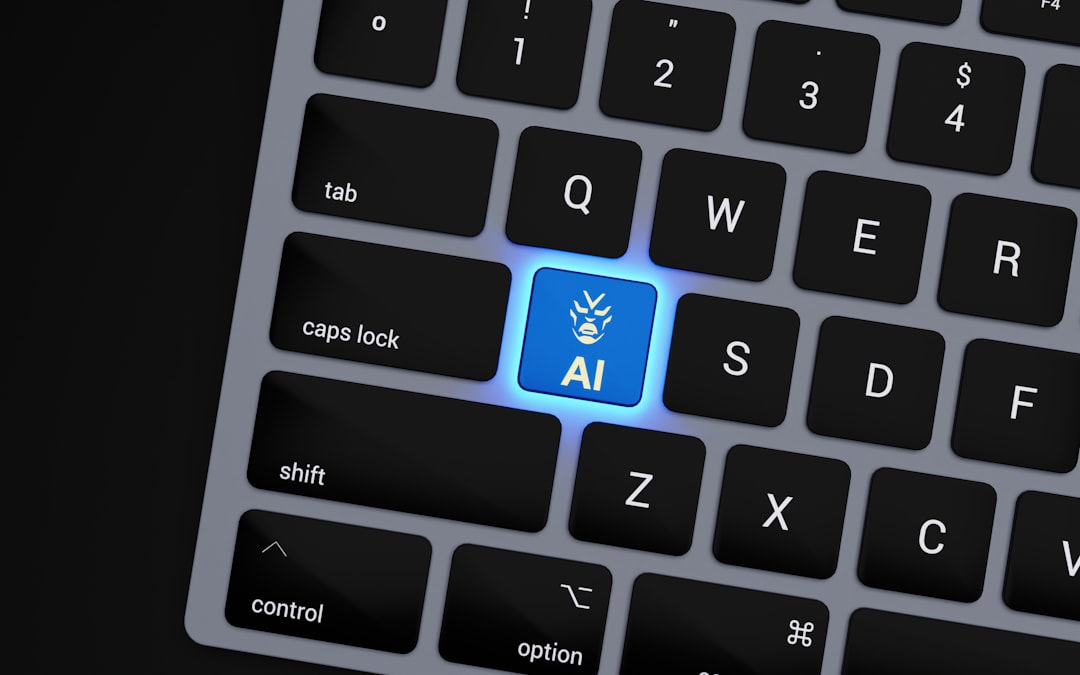
Within the realm of AI-generated blog content, understanding how readers interact with the material has taken on new importance. User engagement metrics, derived from how people use and respond to content, offer a powerful lens through which to evaluate the quality of AI-produced blog posts. Key indicators such as how quickly people start using the information (activation rate), how frequently they return to the content or related products (usage frequency), and how long they remain engaged (retention) are all valuable. These measures shed light on the effectiveness of AI in creating relevant content and how it influences user satisfaction. The relationship between engagement and the quality of AI-generated content is particularly insightful, revealing how well content resonates with audiences and, consequently, how it might be used to achieve specific marketing goals.
As the role of AI in content creation continues to expand, carefully considering these user engagement metrics will become increasingly important. It's crucial for understanding the impact of AI-driven content and making adjustments that lead to improvements in content relevance and overall audience satisfaction. Through a better grasp of how users interact with AI-generated content, it's possible to refine the process to better align with the ever-changing landscape of online consumption.
Here's a rewritten version of the text focusing on user engagement metrics in the context of AI-generated blog content, keeping the original style and length:
How users interact with AI-generated blog content is a fascinating puzzle. We can learn a lot about how effective this new kind of content is by carefully studying user behavior. Here's a glimpse into some of the nuances we've uncovered when looking at user engagement data:
1. It's not just about how many people see a piece of AI-generated content—it's also about how they interact with it. Things like how far they scroll, what links they click, and even how long they pause on certain sections can give us a more precise picture of user engagement. These are like tiny clues that reveal whether the content is truly holding their attention.
2. The old idea that more time spent on a page means better content might need some revision. We've found that users might linger on a page because they're struggling to understand it or it's poorly organized, not necessarily because they're enjoying it. This highlights the complexity of interpreting user behavior.
3. A high bounce rate—where a user quickly leaves a site—isn't always a bad sign. Sometimes, users get what they're looking for fast and move on, feeling satisfied. This suggests that we need to look at the wider context and not just assume a high bounce rate equals poor content.
4. Instead of relying on likes or shares, which can be influenced by various factors, we're finding that repeat visits to a blog post are a more reliable sign of good content. If people come back again and again, that suggests the content truly resonated with them and provided some value.
5. Going deeper than just looking at click counts, we can also analyze click patterns. It can reveal what users are curious about and what parts of the content really capture their interest. This type of analysis can guide future content decisions to maximize engagement.
6. We've noticed that different groups of people engage with content in very different ways. Tailoring content and writing styles to specific audience segments can dramatically improve interaction metrics. Understanding audience differences is becoming increasingly important for crafting effective AI-generated content.
7. Social media shares can be a sign that people find a piece of content valuable, but they can also tell us something about how well the content aligns with a user's identity and how willing they are to share their views with their social networks. The act of sharing is multifaceted.
8. Heat maps, which visually represent where users interact most on a page, offer a powerful way to see what captures people's attention. We can then use this information to tweak content and improve its overall effectiveness in engaging users.
9. The use of images and videos within AI-generated content has a major impact on user engagement. We've seen a significant increase in shares and comments when multimedia is incorporated. It seems that the combination of words and visuals is powerful.
10. The way users interact with content differs depending on whether they're using a mobile device or a desktop computer. We need to adapt content accordingly to ensure it's engaging and easy to consume across all platforms. Optimizing for different viewing experiences is key.
This is a very active area of research as we try to better understand the interplay between AI-generated content and the users who interact with it. It's an exciting challenge to create content that is not just informative but also enjoyable and relevant to a wide audience.
7 Metrics for Assessing AI-Generated Blog Content Quality in 2024 - Readability Scores Calculation With Automated Tools
When evaluating the quality of AI-generated blog content, automatically calculating readability scores becomes crucial. These automated tools offer quick assessments of how easy a piece of text is to understand, which helps writers see if their content is clear and accessible. By incorporating features that check grammar, look for plagiarism, and assess readability, creators can tweak their work to better meet audience expectations. It's important to recognize, however, that automated analysis only provides part of the picture. Human judgment still plays a vital role in understanding the complexities of language and how well ideas connect in a text. Continuously refining readability through methods like using shorter sentences and choosing simpler words can help keep readers engaged and ensure that the content appeals to a broader audience.
Automated tools have become quite popular for quickly assessing how easy it is to understand a piece of text. These tools use formulas, like the Flesch-Kincaid Grade Level, which consider things like sentence length and the complexity of words to generate a score. This score then gives us an idea of the education level needed to understand the text. However, these methods can be a bit simplistic and may not fully capture the intricacies of how people actually understand language.
While these automated readability tools are widely used, they can stumble when dealing with things like idioms or words specific to a certain field. These can confuse the tools and lead to incorrect assessments.
Intriguingly, research has shown that simply having shorter sentences and easier words doesn't always lead to better understanding. For complex topics, sometimes more sophisticated language is needed, which readability scores might not always account for, especially in fields like science or engineering.
The relationship between how easy a text is to read and how engaging it is for the reader isn't always straightforward. Just because something is easy to read doesn't guarantee that people will find it more engaging. Things like whether the topic is familiar to the reader and what they expect from the content also play a big role in how well it's received.
Some readability formulas aren't very helpful when it comes to evaluating creative writing or persuasive writing because they focus on the mechanics of writing rather than aspects like emotional impact and storytelling, which are important for effective communication.
Readability scores can differ greatly across languages, making them tricky to use when dealing with content in multiple languages. What works well for English may not translate to languages with different sentence structures or vocabulary.
With AI-generated content on the rise, there's been some pushback against the idea that readability scores are the be-all and end-all. Some believe these tools might miss important signals that show true understanding, like feedback from readers or qualitative assessments of the content.
In some cases, trying to make content easier to read based only on automated scores can backfire. Oversimplifying the text just to meet certain readability targets can remove important depth and make the content feel shallow or lacking substance.
Research in language suggests that how we write and the tone we use can influence how easy people perceive the text to be. For instance, a conversational tone might be better understood than a formal one, even if they have similar readability scores.
The growing use of automated readability tools raises interesting questions about their impact on education and writing skills. As these tools become more common, writers might find themselves focused on creating content that's easy for these tools to process rather than expressing themselves genuinely, possibly leading to less creativity.
7 Metrics for Assessing AI-Generated Blog Content Quality in 2024 - Factual Accuracy Verification Via External Knowledge Bases
AI-generated content is becoming increasingly prevalent, but ensuring its accuracy remains a challenge. One approach to addressing this is through "Factual Accuracy Verification Via External Knowledge Bases". This involves AI systems accessing external sources of information, like encyclopedias or databases, to verify and augment the content they create. Techniques like retrieval-augmented generation (RAG) are used to incorporate this external information. While promising, integrating external knowledge smoothly into AI-generated content, especially longer pieces, is tricky. Moreover, evaluating the quality and reliability of the information found in external databases is crucial. This includes being able to identify gaps in knowledge and assess how errors or biases in the source material impact the accuracy of the AI's output. As AI content generation evolves, it's becoming clear that balancing accurate information with overall content quality, including coherence, is going to be key.
Thinking about how we can ensure AI-generated content is accurate has led to some intriguing findings regarding the use of external knowledge bases. Here's what we've observed:
1. The size and variety of information held in these external knowledge bases really matters. AI systems that have access to larger and more diverse databases seem to produce more accurate results. This makes sense, since the more information they have to work with, the better their chances are of finding the right information.
2. It's not enough for the information to be accurate; it has to be relevant to the current situation. These knowledge bases have to be updated regularly to keep pace with news and changing societal views, otherwise, the AI might produce accurate but outdated information.
3. To further increase accuracy, some AI systems now use multiple external knowledge sources to check the same facts. This 'cross-verification' greatly reduces the number of mistakes, showing how having extra checks and balances can strengthen the credibility of the information.
4. Some of the newer verification techniques look beyond just simple keyword matches; they examine the context and meaning of sentences. This finer-grained analysis of how things relate to each other seems to improve the reliability of content, especially for complex topics like those found in science and technology.
5. There are systems that can grab new information from knowledge bases in real-time, so they can quickly fix any mistakes as new information becomes available. This differs significantly from systems that rely on pre-loaded information and shows a move towards even more dynamic content generation.
6. By incorporating Natural Language Understanding (NLU), AI systems are able to better comprehend complex user questions. This ability to understand the nuances of what people are asking helps them generate more precise content, ultimately enhancing the accuracy of responses.
7. However, relying on a single knowledge base can create biases or missing information. A better approach seems to be pulling data from a variety of sources. This can help us avoid biases and get a fuller picture of any topic, pushing AI towards more impartial representations of knowledge.
8. We've found that looking at how users interact with content and the feedback they provide can improve accuracy over time. By incorporating feedback loops, AI systems can learn from mistakes and make better decisions for future outputs.
9. Using external knowledge bases brings up some interesting questions related to copyright and attributing information correctly. It can be challenging to determine what's acceptable and what's not, emphasizing the need for clear rules on how to source and use information in AI-generated content.
10. The amount of information generated by AI systems can be overwhelming, making it difficult for humans to do thorough fact-checking. Automated verification tools help lighten the load, but this relies on our trust in the accuracy of those tools. Sometimes there can be a mismatch between our trust in these systems and their true accuracy, especially with very complex topics.
These insights into the use of external knowledge bases are important as we continue to improve the reliability of AI-generated content. There are still questions to answer, but it's encouraging to see how far this field has advanced in enhancing the factual accuracy of the text that AI generates.
7 Metrics for Assessing AI-Generated Blog Content Quality in 2024 - SEO Performance Tracking With Search Engine Analytics
In today's online world, understanding how well a website performs in search engine results is crucial. This is especially true now with AI-generated content becoming more common. We can gain a deeper understanding of SEO (search engine optimization) effectiveness by using analytics tools to track how users interact with online content.
One key aspect of SEO performance tracking is monitoring organic traffic, which essentially measures how many people find your website through search engines like Google. This is a strong indicator of how well your SEO efforts are working and how visible your website is to potential users.
Tools like Google Search Console are incredibly useful for this. They provide insights into keyword rankings and the overall search traffic a website receives. Google Analytics goes further, showing us user behavior—how people interact with a website once they get there. We can track metrics like how long people stay, where they click, and if they convert (e.g., making a purchase).
While metrics like bounce rates (how quickly people leave a site) and exit rates are often tracked, they don't always paint a complete picture of SEO performance. They might be influenced by factors beyond SEO, leading to inaccurate conclusions. Therefore, it's essential to use a combination of tracking tools and metrics to gain a comprehensive understanding of how users are interacting with content and ultimately, the site itself.
As the online world continues to change, it's more important than ever to have systems in place that accurately track website performance. This allows us to adapt and optimize our content to better resonate with the target audience. Using a combination of tools and metrics is the best approach to achieving a true grasp of what is working (or not) in a website's search presence.
Observing how our SEO efforts impact a blog's visibility and user engagement is becoming increasingly vital, especially with AI-generated content. While traditional SEO analysis focuses on ranking and visibility, a deeper dive into user behavior can provide richer insights into the effectiveness of our content strategy, especially as AI continues to shape how we interact with the web. Here's what we've found to be useful in this area:
1. Tracking SEO performance isn't just about seeing how content ranks; it's also about recognizing which emotional aspects associated with particular keywords attract the most readers. This is often overlooked in standard SEO approaches but can be very informative.
2. Examining search engine data can help reveal the motivations behind people's searches. This goes beyond simply matching keywords; it's about catering content to the specific needs and sentiments of our audience. This refined approach can dramatically boost content relevance.
3. It's fascinating that how quickly a site loads can heavily influence SEO ranking. Slow-loading sites tend to have higher bounce rates as users lose patience waiting. Research suggests that even a 1-second delay in load time can reduce conversions significantly.
4. Looking at how consistent users are in their engagement is revealing. A high churn rate—where visitors quickly leave after looking at just one page—may signify either poorly written content or a mismatch between what users hoped to find and what we provided.
5. Metrics like the rate of returning visitors are often underestimated but can be a great sign of genuine engagement and content quality. When users come back to a blog, it usually means they found the content valuable and enjoyable.
6. By using predictive analytics, we can better refine SEO strategies. These techniques analyze patterns in user activity, enabling us to anticipate which kinds of content will likely be popular in the future, rather than just assessing what worked in the past.
7. Things like how far down a page users scroll or whether they click call-to-action buttons reveal a lot about genuine engagement. These behavioral signals are often much more insightful than traditional metrics such as total page views.
8. Local SEO has become much more important—even businesses with a global reach can benefit from seeing how their content is performing in different regions. This localized approach helps us better grasp regional interests and optimize content accordingly.
9. AI-powered SEO tools allow us to adapt strategies in real-time as trends evolve. Marketers can shift gears rapidly instead of relying on periodic assessments that might miss emerging opportunities.
10. Search engines prioritize up-to-date content, which means 'freshness' is a key SEO factor now. Regularly revisiting and updating existing content can give it a significant boost in visibility and engagement.
This is a continually developing field of study as we strive to understand the complex interplay between SEO, user behavior, and AI-generated content. The goal is to create content that is not only relevant and informative but also engaging and fulfilling for a wide audience.
7 Metrics for Assessing AI-Generated Blog Content Quality in 2024 - Content Originality Assessment Through Plagiarism Detection Software
In the world of AI-generated blog content, making sure the content is original is crucial. Plagiarism detection software helps with this by checking the AI-written text against a huge collection of online materials to find any copied content. But, these tools have limitations. Some might miss subtle cases of plagiarism, which makes them less useful. Also, while some software like Copyleaks and Originality.ai are helpful, there are still worries about their limits—things like the number of times you can scan content or the percentage of similarity that triggers a plagiarism alert. These limits can lead to incorrect results, either flagging original content as copied or missing copied sections. As AI-produced content gets more popular, it's important to not just depend on software to ensure originality, but also to establish clear standards for what we consider high-quality content. This means having a more nuanced understanding of originality that goes beyond simple plagiarism detection.
The rise of AI-generated content has brought about a renewed focus on content originality. Plagiarism detection software, once primarily used for academic integrity, now plays a significant role in assessing AI-generated text. Here's a look at some interesting aspects of these tools that are continually evolving:
1. The algorithms driving these tools are becoming more sophisticated. Instead of just looking for exact matches, they're analyzing the underlying meaning and structure of text, which helps them catch paraphrased or reworded content that older tools might miss.
2. These systems are incredibly powerful, able to scan through massive amounts of content in a very short time. This is extremely valuable for universities, publishers, and anyone dealing with a large volume of written work.
3. The databases used by these tools are constantly being updated with new content from websites, publications, and other sources. This helps keep them current, so they can identify even the most recently published materials.
4. We're moving beyond just matching words. New techniques are able to assess how similar the ideas in a piece of writing are, taking into account sentence structure, context, and how ideas flow. This gives us a more nuanced understanding of originality.
5. Some advanced tools can now detect plagiarism across different languages. This is a huge benefit in today's globally connected world, allowing for more comprehensive checks.
6. AI is also being used to put the copied content into context. These tools are becoming better at understanding how words and phrases are used in different situations, which helps them reduce the number of false alarms.
7. Researchers are also looking at user interaction data, like how often a piece of content is shared or interacted with, to help assess originality. This provides an added layer of analysis, offering insights into how users perceive a document's quality.
8. Plagiarism detection tools are becoming more nuanced in their assessments. Instead of just a simple "plagiarized" or "not plagiarized" output, some now provide a gradation of plagiarism, helping us better understand the severity of any issues.
9. It's becoming more common to see plagiarism checkers built directly into writing platforms. This real-time feedback can help people write more original content from the very start.
10. There's a growing emphasis on using plagiarism detection software in a way that's educational. The goal isn't just to catch cheaters but also to teach people about proper citation practices and the importance of intellectual honesty.
Overall, plagiarism detection software is becoming more powerful and sophisticated. It's changing the way we think about maintaining the integrity of content in the digital age. We're moving towards a future where these tools play a more educational role, helping foster a stronger sense of originality and academic responsibility.
7 Metrics for Assessing AI-Generated Blog Content Quality in 2024 - Brand Voice Consistency Evaluation Using AI-Powered Sentiment Analysis
Maintaining a consistent brand voice is crucial when evaluating AI-generated blog content, especially as AI becomes more integrated into marketing. AI-powered tools that analyze sentiment can efficiently sift through a company's existing content to identify recurring patterns in their communication style. This helps them fine-tune their brand messaging. These AI advancements streamline content production and help businesses adapt their voice across various content types and volumes. However, there's a risk that over-reliance on automated systems can lead to a loss of the subtle nuances that come from genuine human expression. This creates a tension between automated content creation and the emotional connection that a true brand voice should have. Recognizing this dynamic is key for businesses looking to establish trust and engagement in today's digital world.
Examining how AI can help maintain a consistent brand voice is quite interesting. We've found that AI-powered sentiment analysis offers a new way to look at this aspect of brand management. Here are some of the nuances we've uncovered so far:
1. AI tools can go beyond simple positive, negative, or neutral sentiment. They're able to detect subtle emotions like sarcasm or irony, which is crucial for accurately reflecting a brand's personality. This added level of detail makes assessments much more insightful than they used to be.
2. AI excels at analyzing huge amounts of data. This means it can get a much wider perspective of how a brand is perceived across various platforms like social media, blogs, and customer feedback, all in real-time. This is a major difference compared to the traditional methods that typically rely on smaller datasets.
3. With AI, we can pinpoint inconsistencies in how a brand is presented. This can be really useful when different teams or even external partners are responsible for content creation. By identifying patterns in sentiments, we can find areas where the brand messaging strays from the intended personality.
4. Sentiment analysis tools are evolving to better understand how language differs across cultures. This can help ensure that a consistent brand voice can be maintained when targeting diverse audiences, which can be challenging to do without the help of AI.
5. There's evidence that brands with a consistent voice are perceived as more trustworthy. AI can help make sure a brand's message aligns with these expectations and perceptions, potentially influencing customer loyalty and purchasing decisions.
6. Integrating sentiment analysis into content processes creates a real-time feedback loop. This enables brands to respond quickly to audience reactions, preventing potential issues or crises that can harm a brand's reputation. It also helps make sure the brand remains aligned with its customers.
7. AI models can now use sophisticated metrics to capture aspects of language that used to be hard to quantify, such as how warm or confident the tone of a message is. These types of metrics provide very practical information to guide brand communication strategies.
8. One of the challenges we've seen with these tools is that they can sometimes reflect biases present in the data they were trained on. This highlights the importance of ongoing refinement to ensure that brand voice consistency assessments are as unbiased as possible.
9. AI tools can analyze historical brand data to anticipate how the brand voice might evolve. By looking at sentiment trends over time, brands can adjust their communication strategy to anticipate shifts in customer preferences and maintain a relevant brand image.
10. We're seeing increased integration of AI-driven brand voice consistency evaluation within overall customer experience monitoring efforts. This integrated approach offers brands a chance to develop a broader strategy that enhances both communication and customer satisfaction.
This is a very active research area, and it's fascinating to see how AI is changing the way brands think about consistency in their voice and communication. There's still a lot to learn, but it's clear that AI will play an increasingly important role in brand management.
AI-powered Trademark Search and Review: Streamline Your Brand Protection Process with Confidence and Speed (Get started for free)
More Posts from aitrademarkreview.com: