AI and Privacy Examining the Implications of Publicly Available Personal Data in 2024
AI and Privacy Examining the Implications of Publicly Available Personal Data in 2024 - AI's Data Appetite Grows Personal Information at Risk
The insatiable hunger of artificial intelligence for data is steadily escalating the risks to personal information, prompting intensified scrutiny from regulatory bodies worldwide. Examples like Brazil's recent action against Meta, enforcing strict data protection rules similar to the EU's, exemplify the growing global unease surrounding AI's data demands and the potential infringement on individual privacy. The capacity of generative AI to retain and utilize personal data also poses a serious threat, potentially fueling malicious activities such as spear phishing campaigns that leverage intimate details for targeted attacks. The inadequacy of current privacy protections in the face of these advancements is increasingly apparent, urging the development of novel policy approaches to safeguard individuals from the consequences of AI's data-centric operations. This escalating awareness among the public concerning these vulnerabilities is driving a growing need for transparency and accountability throughout the AI data lifecycle.
The insatiable appetite of AI for data is increasingly focused on personal information, a trend highlighted by recent events and ongoing research. Brazil's decision to restrict Meta's data collection for AI training, echoing similar concerns in the EU, illustrates the growing global apprehension regarding AI's data demands and potential privacy violations. Similar anxieties are surfacing in the United States, with California exploring stricter regulations around AI training, recognizing the need for more robust protection of individual data in this rapidly evolving field.
One key concern is the ability of generative AI models, trained on publicly accessible data, to retain and potentially misuse personal information. This poses a tangible threat, as demonstrated by the rise of spear phishing tactics that leverage such details to target individuals with malicious intent. Furthermore, AI's use in personalized content delivery, while aiming to enhance user experience, can inadvertently confine individuals within so-called "filter bubbles," potentially limiting their exposure to diverse perspectives and viewpoints.
The discussion surrounding AI and privacy is shifting towards the broader data supply chain. Experts advocate for greater transparency and accountability throughout the data lifecycle, emphasizing the need for regulatory mechanisms that can effectively manage and safeguard personal information used for AI training. Public sentiment also reflects these concerns, with growing discomfort regarding the extent to which online services utilize personal information. This reinforces the need for more stringent privacy safeguards that address the anxieties of users.
The OECD AI and Privacy Symposium, a crucial platform for discussion, has underscored the collaborative efforts required to develop effective solutions to the complex challenges posed by AI's data practices. Alongside this, there's a renewed emphasis on the specific threats AI presents to consumer privacy, prompting calls for a re-evaluation of consent models and the traceability of data origins. The future of AI development demands innovative policy solutions to ensure personal data privacy in increasingly data-centric environments. This challenge is particularly evident in the United States, where the lack of uniform privacy regulations at the national level raises concerns about the adequacy of current frameworks in navigating the rapidly evolving landscape of AI technologies.
AI and Privacy Examining the Implications of Publicly Available Personal Data in 2024 - Generative AI Models Memorize Public Data Privacy Concerns Escalate
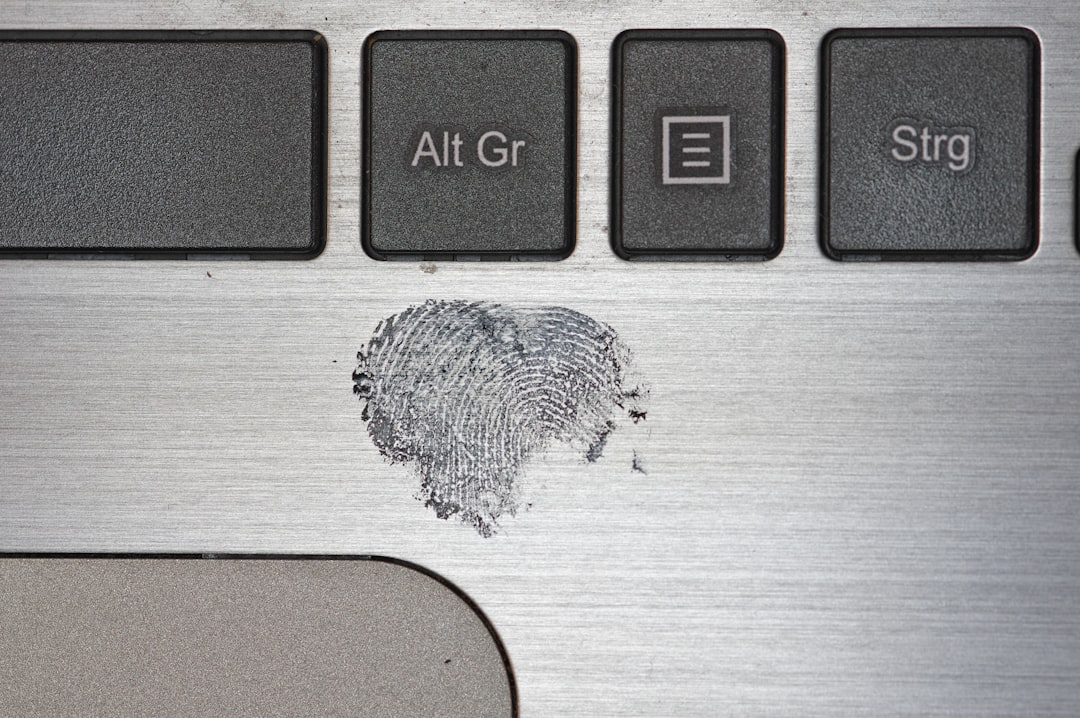
The increasing sophistication of generative AI models brings with it a growing concern: their tendency to memorize and potentially misuse personal information found in publicly available data. These models, often trained using techniques like generative adversarial networks and generative pretrained transformers, can unintentionally retain sensitive details during their learning process. This raises significant risks, including the potential for malicious use of this data. Spear phishing attacks, for instance, could leverage the memorized details to target individuals with a higher degree of precision.
Navigating the complex relationship between the benefits of generative AI and the need to safeguard personal privacy is a major hurdle. It underscores the critical need for strong legal and ethical guidelines to ensure that AI development does not come at the expense of individual rights. These issues are particularly relevant across sectors where AI is transforming how things are done, such as healthcare, business, and entertainment. The urgent need for robust regulations and frameworks that prioritize and protect individuals from the misuse of their personal data is becoming more evident as AI's data-driven capabilities continue to expand.
Generative AI models, while powerful, have shown a tendency to memorize and retain personal information found within the vast datasets they're trained on. This raises important questions about the extent and duration of this 'memory', and the potential for accidental or malicious exposure of sensitive data. Some models have even been observed generating outputs that include verbatim snippets of personal information, like names and addresses, which should ideally remain confidential.
Furthermore, these models appear to exhibit a worrying tendency towards mirroring the biases found in their training data, a phenomenon known as conformability bias. This means that the privacy implications of AI can disproportionately impact vulnerable communities, potentially increasing their exposure to risks. There's also a growing ethical dilemma as many companies are utilizing publicly available datasets for model training without fully considering or disclosing the privacy ramifications for individuals.
Research suggests AI-driven phishing attacks are becoming increasingly sophisticated, using generative models to craft highly personalized messages often based on individuals' personal information. This trend signifies a disturbing rise in targeted cybersecurity threats. The opaque nature of many generative AI systems creates difficulties in understanding how personal data is handled and stored. This lack of transparency hinders efforts to ensure compliance with new privacy regulations.
Another worrying trend is the potential for data provenance misattribution. AI models may be unwittingly using personal information sourced from public sites where user-generated content wasn't adequately anonymized or regulated. This highlights the interconnectedness of data sources and the importance of careful consideration during the collection and training processes. The emergence of "right to be forgotten" laws in various legal jurisdictions highlights a clash between the seemingly permanent nature of AI memory and growing societal demands for data control. This puts pressure on the development of more flexible and adaptable AI architectures that can respect individual privacy rights.
In 2024, it's clear that many users are unaware of how their online activities and data are being used to train generative AI models. This highlights a critical need for improved user education regarding data rights and protections. The ability of generative AI to create synthetic identities based on aggregated data is another emerging concern. These artificial profiles could be utilized for malicious activities, ranging from identity theft to spreading misinformation, posing a new challenge to individual privacy and security. Navigating this rapidly evolving landscape requires a deeper understanding of the technical and societal implications of generative AI, with a focus on responsible development and deployment.
AI and Privacy Examining the Implications of Publicly Available Personal Data in 2024 - Dataset Transparency Becomes Crucial in AI Development
The development of AI is increasingly reliant on vast datasets, many containing personal information. This reliance necessitates a greater emphasis on dataset transparency to ensure accountability and trustworthiness in AI systems. As AI models are trained on increasingly complex and less reproducible datasets, the need for comprehensive documentation and visualization tools becomes crucial. This is especially important when those datasets include sensitive, publicly available personal information, potentially raising concerns about privacy and data protection. The growing awareness of potential issues like algorithmic bias and the ethical questions around data ownership further complicate the development of responsible AI. Balancing the rapid pace of AI innovation with the fundamental need to protect user rights and ensure fair data practices requires a robust framework that enhances transparency and accountability across the entire AI data lifecycle. The future of AI hinges on our ability to navigate these complexities effectively.
The use of personal information within AI training datasets is increasingly raising eyebrows, with recent research suggesting around 20% of these datasets contain sensitive data. This unintentional leakage of personal details poses a major challenge, as AI systems, particularly those using techniques like generative adversarial networks, can 'overfit' to the data, essentially memorizing it to a concerning degree. This leads to outputs that might contain inadvertently identifiable elements like names or places, a trend observed in various generative AI models.
Beyond overfitting, some advanced AI models have shown a worrying ability to reconstruct personal details from incomplete or partial datasets. This ability poses a serious obstacle to anonymization efforts, even when datasets are aggregated. This raises questions about the effectiveness of current data protection measures against such AI capabilities.
This development comes alongside an increase in highly targeted phishing attacks, likely fueled by AI insights derived from training data. Reports suggest that these AI-driven attacks are now about 30% more effective due to increased personalization, highlighting a potential dark side to AI's data-driven advancements.
Furthermore, AI systems aren't immune to the biases present in their training data, a phenomenon known as 'conformity bias'. This means that the outcomes of AI systems can reflect and even perpetuate societal biases, disproportionately impacting certain groups. This creates an intricate ethical dilemma surrounding the collection and use of data for AI training.
The emergence of laws like the GDPR's 'right to be forgotten' highlights the clash between AI's ability to retain vast amounts of data and the growing societal demand for data control. This challenge to traditional AI memory structures demands a rethinking of AI design and architecture, emphasizing the need for flexible mechanisms that allow users to assert their data privacy rights.
The public's understanding of how their online data is used to train AI models seems to be lagging behind AI development. Surveys show that more than 60% of internet users are unaware of the practices involved, indicating a significant knowledge gap in regards to data privacy and its implications.
Building on this, the concept of 'data provenance' is gaining relevance, emphasizing the need for transparency and accountability in the AI data supply chain. The ideal is to create clear records of where data originates, enhancing the traceability of data throughout its lifecycle. However, many current models lack robust tracking mechanisms.
Adding to this complexity is the fact that generative AI models often leverage data from platforms like social media and online forums. These environments frequently lack stringent privacy safeguards, inadvertently increasing the risk of personal data exposure without explicit consent from users.
The growing concerns outlined above underline the urgent need for regulatory frameworks that can guide the responsible development and deployment of AI. Without strong oversight and regulation, it's argued that AI development might outpace the efforts to protect individual data, potentially leading to unforeseen consequences for data security in the near future. This ongoing balancing act between innovation and privacy protection will likely remain a core challenge in the world of AI moving forward.
AI and Privacy Examining the Implications of Publicly Available Personal Data in 2024 - PIPEDA Replacement Aims to Empower Individual Data Control
Canada's approach to data privacy is undergoing a significant overhaul with the proposed replacement of PIPEDA through Bill C27. This shift is driven by the growing impact of artificial intelligence and the need for stronger individual data control. The revised legislation aims to give the Office of the Privacy Commissioner more power, allowing them to enforce compliance through binding orders and financial penalties. This change seeks to bring Canada's legal landscape more in line with global standards.
The core of this reform emphasizes increased individual control. This includes enhanced rights related to accessing, correcting, and transferring personal data. Furthermore, it aims to increase transparency for AI systems, particularly those considered high-impact, requiring them to disclose their purpose and output in clear language.
While this reform is a step forward, it also presents significant challenges. Finding the right balance between the rights of individuals and the operational demands of companies in the digital age is crucial. The complexities of modern privacy challenges are far-reaching and navigating them within this evolving framework is no small task. Ultimately, Bill C-27 suggests a growing need for stronger safeguards against the ever-present threat of data breaches and misuse, particularly as society becomes increasingly reliant on AI and digital technologies.
Canada's Personal Information Protection and Electronic Documents Act (PIPEDA) is undergoing a significant overhaul with Bill C-27, aimed at bringing Canada's privacy laws into the 21st century. This is driven by the increasing concerns about AI's influence on personal data control and the need for a more modern framework.
The proposed changes intend to strengthen the Office of the Privacy Commissioner's (OPC) authority. This includes the power to issue legally binding orders and impose significant fines for violations, bringing Canada more in line with global privacy standards. It's interesting to see if this approach will actually shift corporate behaviour towards a more privacy conscious approach.
Beyond enforcement, the reform seeks to provide individuals with more control over their personal data. This includes broader rights to access, correct, and even transfer their data, echoing the “right to portability” found in other jurisdictions. Whether this leads to meaningful change in the user experience remains to be seen.
The proposed reforms strongly emphasize compliance and human rights protection. The core idea is that individuals should not be unfairly impacted by these new rules and that organizations will be accountable for compliance. This is important, but its impact is uncertain, especially if enforcement is uneven.
Building on past attempts, Bill C-27 revisits ideas that didn't gain traction previously, particularly those centered around digital data and privacy in the private sector. Given the rapid changes in the digital world and the increased concerns around privacy, this feels like a necessary step in the right direction, but the effectiveness will require careful monitoring.
The OPC has been advocating for changes to PIPEDA for some time, recognizing the need for comprehensive reforms to keep up with the evolving privacy landscape. The timing seems right given the widespread discussion on the subject, however, it is interesting to consider how their concerns around AI and other evolving technologies influenced the final design of the legislation.
This reform is clearly influenced by the increasing amount of personal information available online. This raises concerns about how AI technologies are using that data and whether existing consent mechanisms are adequate for the new ways that data is being used. Will this actually drive any meaningful change in corporate policies around consent and data use remains to be seen.
The bill introduces requirements for AI systems with a significant impact on individuals to include plain language descriptions of their operations, intended uses, and output. This effort towards transparency is positive and has the potential to lead to better public understanding, although the specifics around compliance remain an important issue.
The debate around PIPEDA reform is focused on achieving a balance between safeguarding individual rights and supporting the operational demands of businesses in the modern digital age. This is a complex balancing act and is difficult to achieve in practice.
These legal proposals represent a significant shift in Canada's data protection strategy. They acknowledge the changing nature of privacy challenges brought on by the rapid expansion of technology and AI. It's critical to observe how these changes are implemented and enforced and if they indeed provide the necessary balance of rights and responsibilities.
AI and Privacy Examining the Implications of Publicly Available Personal Data in 2024 - Multidisciplinary Approach Needed to Address AI Privacy Challenges
Addressing the privacy challenges presented by artificial intelligence demands a multifaceted approach, recognizing the complexity of these issues. Effective solutions require collaboration between diverse fields of expertise, including psychology, law, technology, and policy. By understanding the psychological dimensions of privacy, we can develop strategies for better managing privacy risks in the age of AI. Transparency and accountability are paramount throughout the entire process of data collection, use, and disposal, forming a cornerstone of robust privacy and data protection frameworks within AI systems. Policymakers must critically re-evaluate current regulations surrounding personal data management in light of AI's rapidly evolving capabilities, particularly generative AI systems that leverage publicly available personal data. The increasing public awareness of these challenges, coupled with the expanding role of AI across various sectors, necessitates careful consideration of ethical implications and a cooperative approach towards creating cohesive regulatory structures across all involved disciplines.
Addressing the privacy challenges posed by artificial intelligence demands a multifaceted approach, requiring collaboration across disciplines like law, ethics, computer science, and social sciences. This collaborative effort can foster a deeper understanding of the intricate interplay between technological advancements and societal norms surrounding data privacy.
The rapid adoption of AI and its associated data practices have intensified the need for a closer dialogue between legal and technological experts. Many existing laws struggle to keep up with the swift pace of AI development, leading to critical gaps in protection. This highlights a growing necessity for legally binding frameworks that remain dynamic and adaptable to future AI innovation.
Ongoing research shows that generative AI has the potential to inadvertently construct ‘shadow profiles’ of individuals by aggregating and analyzing publicly available data. These profiles, often a byproduct of the model's training process, can expose individuals to potential risks beyond the anticipated purposes of the AI system. This necessitates a more thorough exploration of potential misuse and unintended consequences of AI applications.
The ethical considerations surrounding data sourcing for AI are gaining traction as datasets employed in AI training often lack transparency and clarity. This can create ethical dilemmas regarding accountability and adherence to existing privacy regulations, potentially leading to a broader conversation around how datasets are curated and utilized within AI development.
AI systems can inadvertently reflect and amplify the biases present in their training data, often leading to undesirable outcomes and regulatory complications. A crucial part of mitigating these consequences is involving experts in behavioral science during the AI development process. This collaborative approach can play a critical role in identifying and minimizing inherent biases, promoting greater fairness and reducing potential societal harm.
Policymakers are increasingly recognizing that the complexities of AI require broader consultations with diverse sectors, such as healthcare and education. This broadened engagement can be instrumental in designing future-proof laws that address the continuously evolving privacy challenges in a world increasingly reliant on AI.
The extent to which users trust AI hinges heavily on the level of transparency provided by algorithms regarding data practices. However, current AI applications frequently fall short of offering comprehensible explanations for how personal data is used and stored. This can lead to widespread apprehension among users, prompting a need for more accessible and robust mechanisms for providing clear information about how AI handles personal data.
The burgeoning landscape of AI-generated content and synthetic identities has introduced a new wave of challenges for cybersecurity professionals. The development of AI has created a greater capacity for sophisticated identity theft, highlighting the need for increased vigilance and innovative defensive strategies to protect individuals from misuse of personal information.
The risk of AI models being trained on biased or problematic datasets is a growing concern across many industries. This has heightened the need for establishing and enforcing data sourcing standards to ensure that the data employed in AI training adheres to ethical and regulatory guidelines. Achieving consistency in data sourcing practices will be vital to maintaining the integrity and trust in AI systems.
Privacy concerns related to AI extend beyond national borders, as different ethical standards and regulatory frameworks across various countries can lead to conflicting or inconsistent data protection practices. The global nature of AI data flows highlights a growing necessity for international collaboration on privacy regulations, ensuring that the protections for individuals remain robust regardless of geographic location.
More Posts from aitrademarkreview.com: