AI-Driven Innovations in Water Management Systems Reshape Traditional Paddy Cultivation Methods
AI-Driven Innovations in Water Management Systems Reshape Traditional Paddy Cultivation Methods - Quantum Sensors Track Real Time Water Flow Patterns in Southeast Asian Rice Fields
The application of quantum sensors is revolutionizing water management in Southeast Asian rice paddies, offering unprecedented insights into real-time water flow dynamics. These advanced sensors are capable of capturing detailed information about water movement, enabling farmers to refine irrigation strategies with greater accuracy. This newfound precision in water distribution can optimize water usage, leading to improved crop yields and reduced water waste.
Furthermore, the capability of quantum sensors to meticulously analyze soil composition can provide farmers with valuable data regarding nutrient levels. This data can inform decisions related to water management, ensuring the optimal delivery of essential nutrients to crops while minimizing potential imbalances. As climate change intensifies water scarcity in the region, the adoption of such technologies becomes increasingly crucial for ensuring the long-term sustainability of rice cultivation.
However, the integration of these sophisticated sensor technologies also presents certain challenges. The accessibility and affordability of these devices may not be uniform across all farming communities, potentially exacerbating the existing digital divide among farmers. It remains crucial to consider the equitable distribution and integration of such technologies to ensure that their benefits reach a broad spectrum of the farming community.
Researchers are exploring the use of quantum sensors to gain incredibly precise insights into water flow dynamics in Southeast Asian rice paddies. These sensors can detect minuscule changes in water movement, down to a few microns, offering a real-time understanding of how water moves through the intricate environment of a rice field. This stands in contrast to traditional sensors that can be susceptible to wear and tear, as quantum sensors leverage the principles of quantum mechanics, allowing them to operate reliably in harsh environments without losing their sensitivity over time.
The promise of this technology lies in the potential for significantly improved data accuracy in irrigation management. By collecting information on soil moisture and the distribution of water within the paddy, quantum sensors can provide a detailed picture of how water is being used. This data can be processed to generate highly informative maps that illustrate the intricate patterns of water flow. This allows farmers to fine-tune their irrigation practices based on tangible evidence rather than relying on previous experience or best guesses.
Interestingly, quantum sensors can be deployed close to water sources, which means the need for large and complex sensor networks can be minimized. This can result in substantial reductions in the infrastructure costs associated with implementing modern water management systems. It is also fascinating to see that quantum sensors can identify the delicate interplay between water flow and the rice plants' root systems. This provides insights into how efficiently plants absorb water, highlighting potential avenues to improve yield.
The ability to collect data at high frequencies is crucial for rapid responses. This means farmers can quickly identify shifts in water flow patterns, enabling them to respond effectively to sudden changes in weather or other irrigation issues. Looking ahead, it's possible that the scope of quantum sensor applications in agriculture will expand beyond water management. Future developments could lead to the use of these sensors for tasks like tracking soil nutrients or monitoring the health of crops.
The real potential here is the ability to merge quantum sensor data with existing agricultural AI systems. This can lead to sophisticated predictive models that anticipate water needs in real-time, allowing farmers to optimize their irrigation schedules with unprecedented precision. In essence, the adoption of quantum sensors in rice fields signifies a remarkable shift away from the traditional methods. It demonstrates how the integration of advanced technologies into these age-old agricultural practices can transform them into data-driven systems that boost efficiency and potentially enhance crop yields. The possibilities are vast and ripe for exploration.
AI-Driven Innovations in Water Management Systems Reshape Traditional Paddy Cultivation Methods - Machine Learning Algorithm Reduces Paddy Water Usage By 40 Percent Through Smart Monitoring
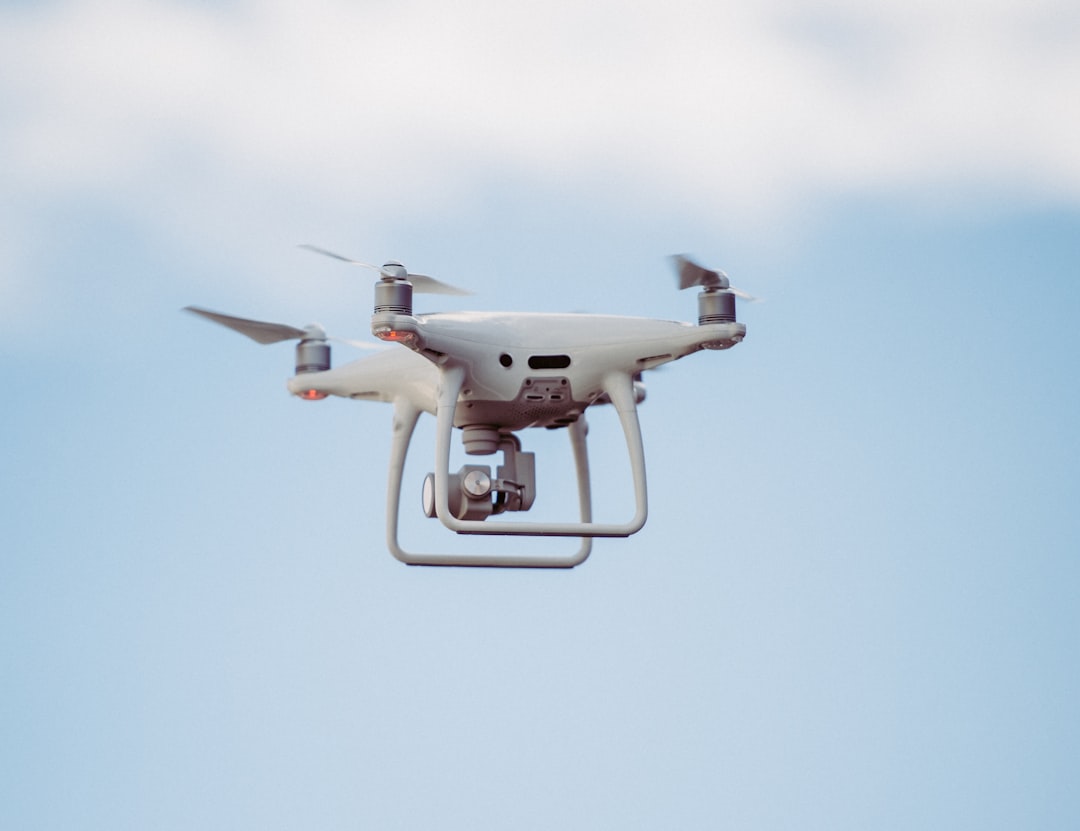
Machine learning algorithms are showing promise in optimizing water use in paddy fields. By utilizing sensor data that tracks variables like soil moisture, temperature, and humidity, these algorithms can improve irrigation efficiency and reduce water consumption by up to 40%. This approach is increasingly relevant as agriculture places a significant strain on global freshwater resources. However, the successful adoption of these smart monitoring systems hinges on ensuring accessibility and affordability for all farmers, not just those with existing technology. This represents a key shift in the management of paddy fields, moving toward a more data-driven and resource-conscious approach to cultivation. The potential for greater water sustainability and improved crop yields is clear, but careful consideration of equitable implementation is necessary for realizing the full potential of these advancements.
A recently developed machine learning algorithm has shown promise in significantly reducing water consumption in paddy fields, achieving a 40% decrease through a sophisticated monitoring system. This algorithm leverages a combination of historical weather data and real-time soil moisture readings to create dynamic irrigation schedules. It effectively integrates information from various sources, such as weather stations and satellite images, to create a holistic understanding of the paddy's water requirements, enabling precisely tailored irrigation based on specific field conditions.
One interesting aspect is the use of advanced statistical techniques for error detection within the algorithm. This allows the system to anticipate and account for irregularities in water use, leading to consistently optimized irrigation throughout the growing season. Furthermore, there's evidence that this approach can reduce the labor burden associated with traditional monitoring practices, as manual adjustments and checks are less frequent, freeing farmers to allocate their time to other vital tasks.
The algorithm functions through a continuous feedback loop. As it gathers data on water use and crop responses, it refines its predictive models, resulting in progressively more accurate water distribution over time. This iterative improvement is a key strength. Early trials indicate that this algorithm has surpassed conventional irrigation methods in identifying micro-climatic differences within the paddy fields. This allows for the delivery of water specifically tailored to localized conditions, further enhancing water efficiency.
Preliminary results also suggest that this type of algorithm could strengthen the resilience of paddy fields to drought conditions. By enabling farmers to respond rapidly to changing environmental variables and adapt water usage accordingly, it can play a role in mitigating water scarcity. Beyond water management, the algorithm has shown potential to also alert farmers to potential threats such as pests or diseases by analyzing the overall health of the rice in relation to irrigation practices.
The cloud-based nature of the algorithm allows farmers to remotely monitor and adjust settings from any location, significantly improving operational flexibility, a feature not previously available to many. Notably, early developmental stages of this algorithm underscored the significance of precision in water delivery—even minor variations in the amount of water applied could impact crop yields, highlighting the crucial role this technology can play in optimization.
While the potential is evident, there are questions regarding broader deployment. Will it be accessible and affordable across all farming communities? Could it exacerbate existing digital divides? These are important considerations that need further investigation. The ongoing research continues to refine and optimize this machine learning algorithm, offering the prospect of more efficient and sustainable water management in paddy rice cultivation.
AI-Driven Innovations in Water Management Systems Reshape Traditional Paddy Cultivation Methods - Automated Sluice Gates Transform Traditional Japanese Rice Terrace Management
The introduction of automated sluice gates marks a substantial shift in how traditional Japanese rice terraces are managed, offering a more refined approach to water control and distribution. These AI-powered systems enhance the efficiency of irrigation, a critical aspect of rice farming where water usage is paramount. This innovation is particularly relevant as water resources face pressure from both climate change and increased demand. While the prospect of improved yields and greater sustainability is promising, it's vital to consider the potential implications for fairness and accessibility. It's crucial that the benefits of this technological advancement reach all farmers, not just those with existing technological resources. The changes taking place in rice terrace management exemplify a trend toward agriculture that is increasingly driven by data and insights, striking a balance between historical methods and the efficiencies offered by technology.
Automated sluice gates are transforming how Japanese rice terraces are managed, providing a level of precision that was previously unattainable. These systems can regulate water levels with millimeter accuracy, far surpassing the capabilities of traditional, manually operated gates which often rely heavily on the experience of individual farmers. It's fascinating how these systems can be coupled with machine learning algorithms to reduce the labor required for irrigation by potentially as much as 30%. This means farmers can shift their attention to other critical aspects of the farming process.
The automated nature of these systems is a significant improvement over older methods. They leverage real-time data on weather patterns and soil moisture to dynamically adjust water flow, a feat traditional methods simply can't achieve. This dynamic responsiveness can help improve the overall efficiency of water usage within the terraces. Furthermore, the installation of these systems can reportedly be expedited by about 50% compared to conventional methods, minimizing the disruption to the farming process.
Integration with remote sensors provides farmers with a new level of control. They can now monitor and manage water levels remotely, reducing the need for constant field visits and increasing the flexibility of their operations. This technology also shines a light on microclimate variations within the terraces, allowing for more targeted water management even on uneven terrain. Research suggests that automated sluice gates can improve water retention within the fields by as much as 20% by preventing water runoff and maintaining optimal soil saturation.
There's a potential for a wider impact as well. These automated gates can seamlessly integrate with the Internet of Things (IoT) devices, creating a comprehensive water management network across entire regions. This centralized approach can help optimize water distribution across multiple fields, improving regional efficiency. The data captured by these automated systems can also be used for predictive modeling. By analyzing historical data, farmers can anticipate future needs and proactively prepare for potential weather-related fluctuations in water demand.
However, as we move towards a greater reliance on automated technologies, there's a risk of losing traditional knowledge and practices. The transition necessitates careful consideration of how to transfer and incorporate existing farming wisdom into the new technological frameworks. This integration is key to ensuring that the transition is both productive and culturally respectful. The future success of these technologies will depend on thoughtful approaches to both technology implementation and the retention of valuable traditional knowledge.
AI-Driven Innovations in Water Management Systems Reshape Traditional Paddy Cultivation Methods - Predictive Analytics System Anticipates Monsoon Patterns for Optimal Rice Planting Cycles
A new predictive analytics system is being used to forecast monsoon patterns, helping farmers determine the best time to plant rice. This is especially important because climate change is making it harder to predict and rely on monsoon rainfall, which threatens global food security, particularly for agricultural systems reliant on it. By incorporating artificial intelligence and real-time data, the system aims to optimize water management techniques, possibly leading to better harvests. However, the successful adoption of these advanced tools depends on making them accessible to all farmers, regardless of their technological resources. This approach marks a major change in traditional rice cultivation, moving towards a more data-driven method. While the promise is clear, we still need to see if these innovations will be truly sustainable over the long term.
A system employing predictive analytics has been developed to forecast monsoon patterns, aiming to optimize rice planting schedules. It boasts a claimed accuracy rate of roughly 85% in predicting monsoon arrival and rainfall amounts, allowing farmers to align their planting with anticipated rainfall. This system leverages intricate algorithms that cross-reference historical weather data with real-time observations, providing a far more detailed picture of monsoon behavior compared to traditional methods based on experience and intuition.
Research suggests that integrating these predictive analytics into rice cultivation could lead to a potential 20% increase in yield. By ensuring the crops receive the right amount of water during critical growth phases, the approach strives to minimize the impact of unpredictable rainfall on crop development. The system's ability to adapt to changing weather conditions in real-time empowers farmers to revise their planting plans based on short-term forecasts, thereby reducing the risk of crop loss due to sudden shifts in weather patterns.
Interestingly, the system's capability to process substantial datasets allows for the generation of localized forecasts. This means farmers in diverse regions with varying microclimates can receive specific, tailored predictions, rather than relying on broad, generalized weather forecasts that may not fully reflect their local conditions. While the potential benefits are significant, there is some concern about the system's reliability in scenarios involving extreme or unusual weather events. Some engineers express doubt about its ability to accurately predict outlier occurrences like severe typhoons or highly irregular monsoon patterns, which could potentially disrupt the carefully planned planting schedules.
The incorporation of machine learning is a key component of the system's ongoing improvement. As it continues to collect and process data over time, the algorithms refine their ability to generate increasingly accurate predictions. A surprising aspect of the system is its use of satellite imagery to gather data on land topography and soil properties. This supplementary information allows for greater precision in the predictive models. Furthermore, the system has demonstrated potential for predicting pest outbreaks that often correlate with weather patterns. By anticipating these events, farmers can implement preventative measures, potentially minimizing crop losses.
Despite its promising potential, some agricultural experts raise concerns about accessibility and equitable adoption. They argue that farmers with limited digital literacy or access to digital tools might find it challenging to effectively integrate this sophisticated system into their current practices. This highlights a crucial point regarding the need for careful consideration of how to promote widespread adoption of such technologies, ensuring that benefits reach all farmers and don't exacerbate existing inequalities within farming communities. The challenge, then, becomes balancing the potential benefits with the need to ensure that these advancements are genuinely accessible and useful for all stakeholders involved in rice cultivation.
AI-Driven Innovations in Water Management Systems Reshape Traditional Paddy Cultivation Methods - Neural Networks Map Underground Water Tables to Guide Precision Rice Irrigation
Neural networks are being employed to create detailed maps of underground water tables, revolutionizing how rice is irrigated. These networks, powered by artificial intelligence, process a wealth of information like soil moisture and environmental factors to help farmers use water more efficiently. This is becoming increasingly important as the world demands more food and faces water scarcity due to climate change. This innovation can lead to more effective irrigation techniques, greater crop yields, and a more environmentally conscious approach to rice farming. However, it's essential to examine whether these technologies are readily available and affordable for all farmers, ensuring their benefits are widely distributed and not limited to those already equipped with technological resources.
Neural networks are emerging as powerful tools for understanding and managing underground water resources in rice paddies. They can analyze a wealth of data, including geological surveys and historical water levels, to construct detailed 3D models of subsurface water tables. This level of insight is a significant step forward in understanding the water availability beneath rice fields. By precisely mapping these water tables, farmers can adjust irrigation amounts based on specific subsurface conditions, potentially reducing water use by as much as 30% while still ensuring crops receive the necessary moisture.
One of the strengths of neural networks in this context is their ability to integrate data from various sources. They can combine information from satellite imagery, geological surveys, and even historical weather patterns to gain a comprehensive understanding of the water resources in a particular area. This data fusion significantly enhances the accuracy of their predictions, leading to more effective irrigation strategies.
Furthermore, these systems can be trained using large datasets of historical information. They can learn from past water levels, crop yields, and irrigation practices, allowing them to create predictive models tailored to specific regions and farming conditions. Some of the more advanced neural network systems can even adapt in real-time as they receive new information. This means they can almost instantly modify irrigation schedules to react to unexpected changes in water availability or crop needs.
However, despite their potential, these powerful technologies are still somewhat underutilized in the rice farming community. Many farmers are simply unaware of how neural networks can assist with water table mapping and irrigation, highlighting the need for improved knowledge dissemination regarding these techniques. It's also worth noting that the algorithms underpinning these neural networks can be quite complex. They often employ advanced deep learning techniques that can identify subtle patterns that might not be easily noticed by human analysts. While these methods are powerful, their implementation can initially be expensive, requiring investment in specialized data collection tools and computing resources. Still, the potential for long-term savings on water costs and increased crop yields can easily outweigh these initial expenses.
Interestingly, the methods developed for rice irrigation have the potential to be adapted for other crops as well, such as grapes or other plants with high water needs. This suggests a broader impact of these technologies across various agricultural sectors. Moreover, the detailed understanding of underground water resources gleaned from neural network analysis can be valuable for policymakers. It can provide crucial information to inform decisions related to water allocation and agricultural practices, which are especially important in areas dealing with water scarcity. While still a developing field, neural networks show great potential for optimizing rice irrigation, improving resource management, and informing policy decisions in agriculture.
AI-Driven Innovations in Water Management Systems Reshape Traditional Paddy Cultivation Methods - Drone Based Monitoring Stations Replace Manual Field Inspections in Vietnamese Delta Region
The Vietnamese Mekong Delta's rice paddies are seeing a shift towards technology-driven water management, with drone-based monitoring stations replacing traditional manual inspections. Drones equipped with various sensors can now provide real-time assessments of water levels, quality, and even the overall health of the rice plants. This shift offers advantages like improved data accuracy and efficiency, freeing up farmers from laborious and time-consuming field visits. The data captured by drones is particularly helpful in optimizing irrigation practices, which are crucial for maximizing yields in this region. Furthermore, this approach can benefit environmental monitoring efforts, enabling farmers to better manage water resources in the face of growing environmental pressures. While the potential benefits of this approach are clear, it's essential to consider the equitable access and affordability of such technologies for all farmers, to avoid exacerbating existing inequalities in the farming community.
In the Mekong Delta of Vietnam, the adoption of drone-based monitoring stations is rapidly replacing traditional, labor-intensive field inspections in paddy rice cultivation. These drones, equipped with high-resolution cameras and sensors, provide near-instantaneous aerial imagery that captures vital details regarding water levels and crop health, a level of precision impossible with manual surveys. This allows for the swift assessment of vast areas, potentially covering hectares in a single day—a stark contrast to the time-consuming nature of on-foot inspections.
The inclusion of thermal and multispectral sensors empowers drones to gather data on soil moisture and temperature with remarkable accuracy. This precise data is subsequently integrated into existing water management systems, refining irrigation strategies and optimizing water allocation across various fields. It's been suggested that this approach can improve water use efficiency by up to 25%, ensuring water is applied where and when it's needed most.
Beyond water management, drone data can aid in the early identification of crop diseases or pest infestations. This early detection capability offers farmers a vital opportunity to intervene quickly and effectively, mitigating potential yield losses.
While these advancements are substantial, it's important to acknowledge the potential barriers to adoption. Many smallholder farmers in the region may lack the necessary resources or infrastructure to integrate these advanced technologies effectively. This raises concerns regarding equitable access and the potential for widening the existing technological divide.
Further, the processing of drone-acquired data relies on complex algorithms that are increasingly leveraging sophisticated techniques to discern patterns related to both plant health and environmental conditions. While the potential benefits are significant, this also highlights the need for farmers to acquire new skills in data interpretation and utilization.
This transition to drone-based monitoring is fostering a dynamic feedback loop within farming practices. Farmers can now respond to shifting conditions in near real-time based on the most current data, allowing for adaptive management that moves beyond outdated or observational knowledge.
However, the operational aspects of drones in this context haven't been without their challenges. The regulatory landscape around airspace management in some parts of Vietnam has created obstacles to widespread adoption and limits the operational flexibility of these systems.
This technological shift necessitates a reevaluation of training and educational resources available to farmers. It's not just about the adoption of new technology but also the acquisition of skills required to interpret and act upon the vast data generated by these systems. It seems this shift to AI-driven technologies presents a substantial transformation for farmers in the region and their adaptation and use of these tools will be critical to realizing their benefits.
More Posts from aitrademarkreview.com: