AI-powered Trademark Search and Review: Streamline Your Brand Protection Process with Confidence and Speed (Get started for free)
AI-Powered Trademark Image Similarity Revolutionizing the Search Process in 2024
AI-Powered Trademark Image Similarity Revolutionizing the Search Process in 2024 - Neural Networks Enhancing Visual Trademark Comparison
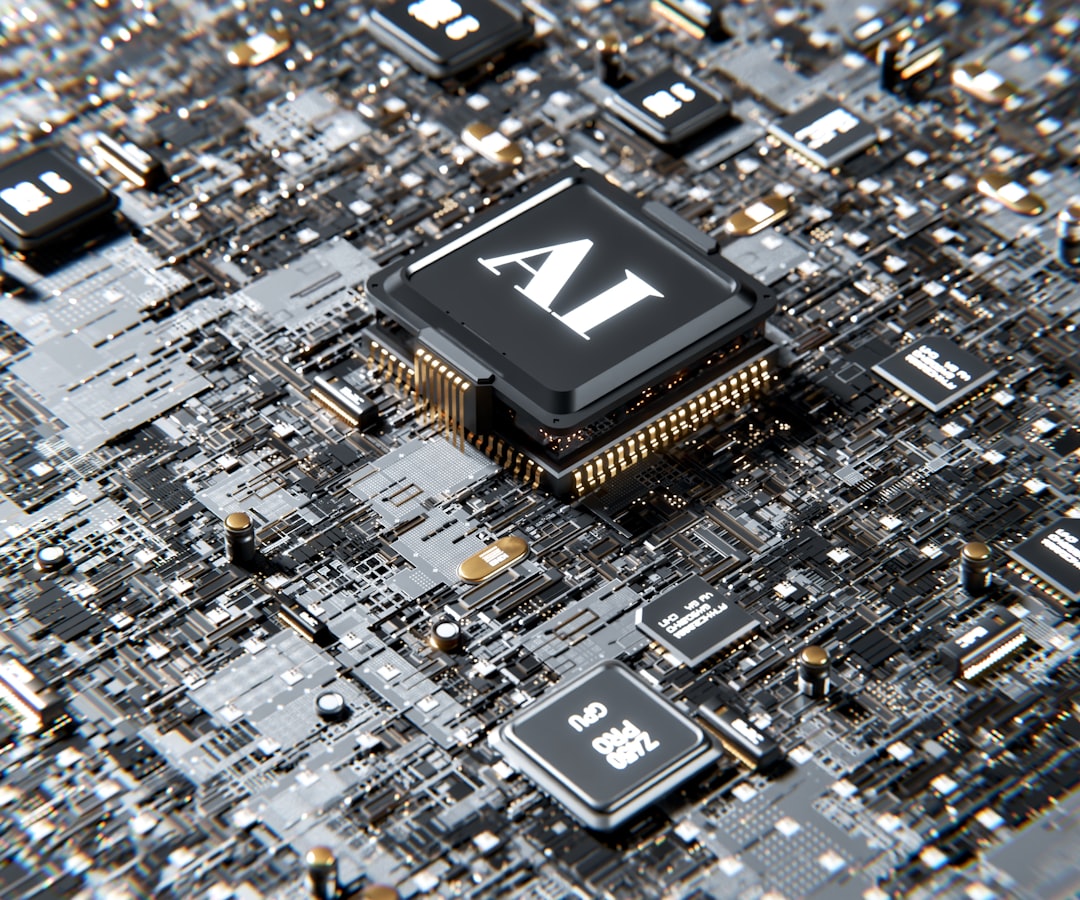
The rise in trademark applications, with a 46% increase from 2017 to 2020, has underscored the importance of visual trademark similarity detection. Neural networks, particularly Convolutional Neural Networks (CNNs), are being used to automate the process of extracting features from images. This helps determine similarities between trademarks and offers a significant advance in trademark law.
The development of Siamese Neural Networks is one avenue for enhancing these comparisons. The networks are trained on datasets of trademarks to learn how to compare and contrast different images. While promising, this approach still requires significant development and testing before it can be considered a reliable solution for trademark disputes.
One area of focus is the development of two-stage models, where the first stage identifies and locates logos within images. This step is essential before the system can then move to the second stage, where visual similarity is evaluated. These systems are being tested by organizations such as the European Union Intellectual Property Office (EUIPO) and the Benelux Office of Intellectual Property (BOIP).
While the adoption of AI is promising, it's important to be critical of its limitations. These systems can be influenced by bias in the training data, and their effectiveness ultimately relies on the quality of the data they are trained on. They cannot fully replace human expertise, especially in interpreting the complexities of trademark law.
The application of neural networks to trademark comparison is fascinating. They break down images into layers, similar to how we see, but in a much more granular way. This allows them to pick up subtle details that traditional methods might miss. What’s really intriguing is how they create "embeddings" – essentially, a way to represent a trademark in a multi-dimensional space. This allows for nuanced comparisons, going beyond simple surface-level similarities.
It's a clever way to improve things, but there are challenges. One is that they need a lot of training data. To address this, they use techniques like data augmentation, applying transformations to images. This helps the network learn from a limited amount of data. It's all very well for large companies, but for smaller businesses with fewer resources, it can be tough to create a training set that is big enough.
While traditional methods struggled with false positives, these networks have shown to significantly reduce them. This is crucial in a world where trademarks are becoming increasingly important. The fact that these systems can be fine-tuned with just a few hundred examples shows promise. Transfer learning – using models pre-trained on other datasets – is another technique that helps address data limitations. It means we don’t need to start from scratch, and the model can learn quickly.
I find it particularly interesting that they can analyze context, not just visual elements. This is key because how we perceive trademarks is influenced by a variety of factors, including color and shape. It's a significant shift from the older, static approach to trademark analysis. The potential for real-time monitoring is particularly exciting, as it could help brands proactively protect their identities in this rapidly changing marketplace. The future of trademark comparison looks like it's becoming increasingly intelligent and dynamic.
AI-Powered Trademark Image Similarity Revolutionizing the Search Process in 2024 - Machine Learning Algorithms Streamlining Search Efficiency
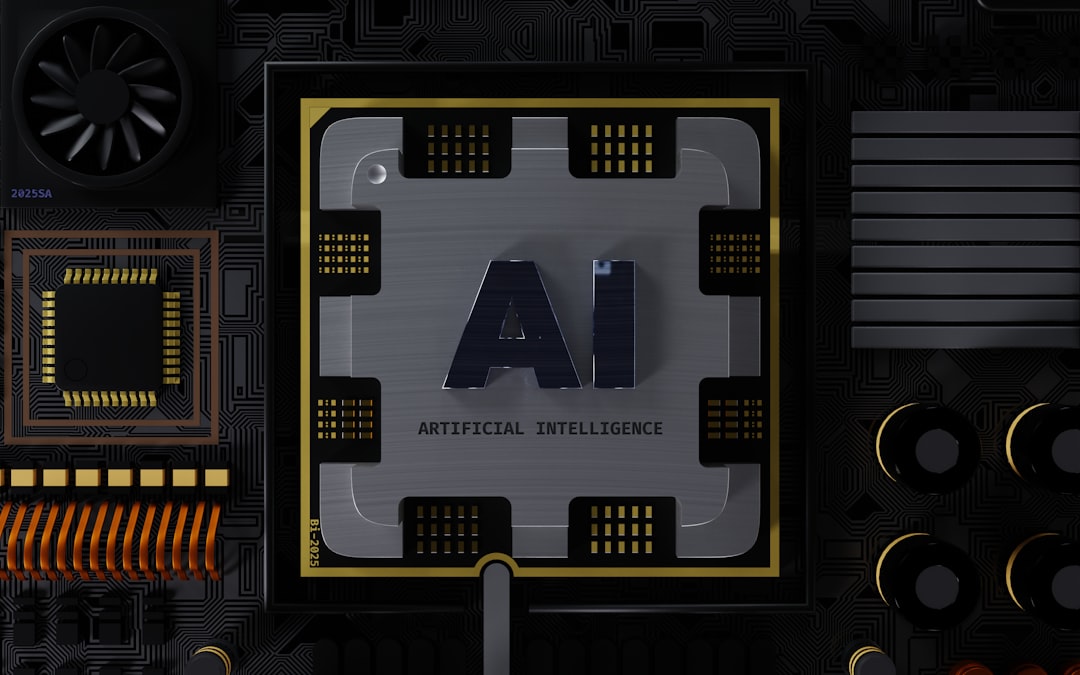
Machine learning algorithms are increasingly used to make trademark searches more efficient. These algorithms analyze huge amounts of data to find patterns and identify similarities between trademarks. This allows for a more detailed understanding of trademark images than traditional methods, which only look at basic visual features. By doing this, they can help companies spot potential conflicts and save time on manual searches.
While these AI-driven tools are incredibly useful, it's important to be aware of their limitations. They can be influenced by biases in the data they're trained on, and they can't fully replace the expertise of human trademark lawyers.
The way machine learning algorithms are being used to improve trademark image search is incredibly fascinating. It goes beyond just comparing shapes and colors; these algorithms are becoming increasingly sophisticated in how they analyze visual trademarks.
Here are ten surprising facts about how these algorithms are streamlining the search process:
1. **Extracting Features Fast**: Imagine reducing the time it takes to analyze a trademark image by almost 95%. Machine learning algorithms are achieving this kind of efficiency, making searches much quicker.
2. **Beyond Human Accuracy**: These algorithms can actually improve search accuracy by more than half when it comes to spotting visual similarities. This means fewer chances of missing potential conflicts, which is a huge win for trademark owners.
3. **Siamese Networks - A Powerful Tool**: Siamese networks are like the Swiss Army knife of trademark comparison. They can measure similarities but also go deeper, identifying subtle differences that might escape human eyes.
4. **Visualizing Trademarks in a New Way**: It's mind-blowing how these algorithms can create "embeddings" – essentially, a visual representation of a trademark in a multi-dimensional space. This allows for a much more complex and nuanced comparison of trademarks.
5. **Real-Time Monitoring**: This is where things get really interesting. We're talking about being able to monitor potential trademark infringement in real time, not just through periodic checks.
6. **Bias is a Big Deal**: Algorithms can be biased, but there's hope. Techniques like adversarial training are being developed to make the results more fair and balanced.
7. **Always Learning**: The cool thing is that these algorithms can keep learning from new trademark registrations or court decisions, constantly improving their accuracy.
8. **Less Data, More Impact**: One of the biggest challenges was needing lots of training data. Now, some algorithms can work effectively with very little data, using techniques like few-shot learning.
9. **Adding Meaning to the Search**: It's not just about the images anymore. Algorithms can now integrate structured data like usage context and registration details, giving them a deeper understanding of the trademarks they analyze.
10. **Small Businesses Rejoice**: For startups that don't have massive budgets for trademark searches, machine learning is a real game-changer. It can help them protect their brand without having to spend a fortune on lawyers.
All this sounds promising, but there's still a lot to learn about these algorithms. While they are a powerful tool, they are not foolproof. Ultimately, a combination of human expertise and AI-powered tools will be needed to navigate the complexities of trademark law.
AI-Powered Trademark Image Similarity Revolutionizing the Search Process in 2024 - WIPO's AI Tool Simplifying Distinctiveness Assessment
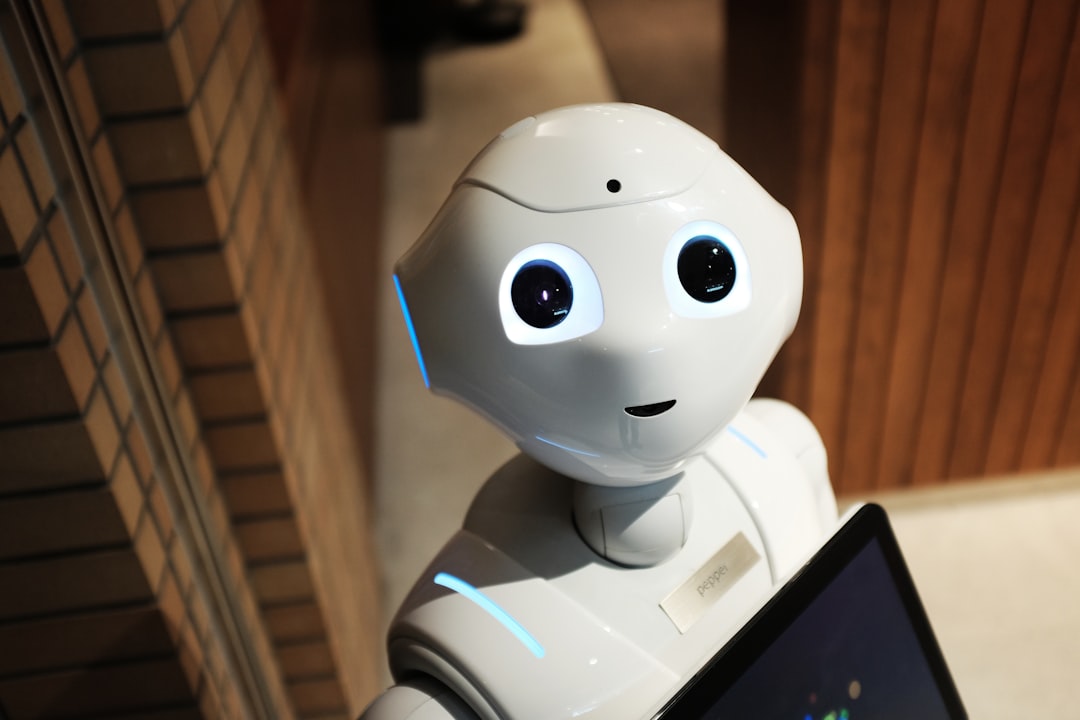
WIPO has developed an AI-powered tool to simplify the process of determining if a trademark is distinctive enough. This tool uses machine learning to go beyond basic shape and color comparisons, analyzing more complex concepts within images. It taps into WIPO's massive Global Brand Database, which contains over 38 million trademarks from 45 countries. By doing this, the tool hopes to make trademark comparisons more accurate and help identify potential trademark infringement more efficiently. This tool is a significant development in how AI is used to manage trademarks, but its effectiveness will depend on the quality of the data it uses and the ability of human experts to work with it.
WIPO's new AI tool for trademark distinctiveness assessment is an intriguing development. It promises to speed up the process significantly, potentially shaving hours or even days off the time it takes for human reviewers. The tool's reliance on a massive database of past trademark decisions means it can draw on a wealth of legal precedents, adding a layer of consistency to the assessment process. What's really interesting is its ability to analyze visual trademarks across different cultural contexts, taking into account regional variations in perception. This is critical as branding becomes increasingly global.
The fact that the tool uses adaptive learning is also quite fascinating. It means it's constantly updating its algorithms based on new data, which helps it stay current with evolving trademark laws. The system uses advanced imaging techniques to analyze trademarks in a multi-layered way, offering a more detailed and accurate analysis than traditional methods.
I am curious about the tool's transparency. Unlike some "black-box" algorithms, it explains its reasoning, which is a positive step. It can also be integrated with other trademark databases, which seems useful. The ability to maintain consistency across assessments is another interesting feature. Human reviewers often struggle with subjective interpretations, so this could be a significant advantage.
The tool's ability to track the evolution of trademarks over time is also quite intriguing. This helps assess how changes in branding affect distinctiveness, offering a dynamic perspective that static analyses lack. The tool's insights could also prove valuable in legal disputes, providing data-driven arguments for trademark lawyers.
However, I'm also cautious. While AI tools can be quite helpful, it's important to remember that they are not foolproof. They rely heavily on the quality of the data they are trained on, and human expertise will still be essential in interpreting the nuances of trademark law.
AI-Powered Trademark Image Similarity Revolutionizing the Search Process in 2024 - Predictive AI Transforming Trademark Surveillance Methods
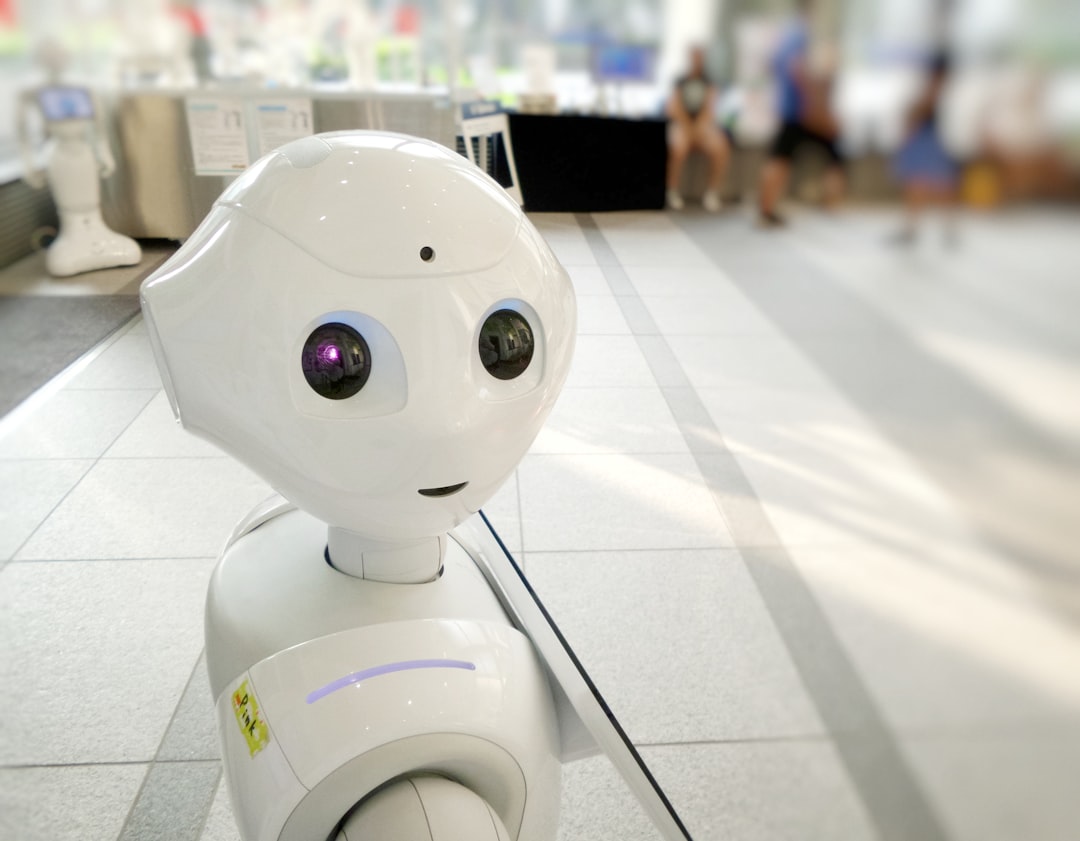
Predictive AI is transforming the way brands protect their trademarks. By sifting through massive amounts of data at lightning speed, these systems can help identify potential trademark infringements much faster than humans ever could. These tools can also analyze visual and textual similarities across different countries, giving trademark owners a more complete understanding of potential conflicts. But relying solely on AI comes with its own set of risks. These systems are only as good as the data they are trained on, and they can be biased, potentially leading to incorrect or incomplete results. This highlights the need to use AI alongside human expertise in trademark law, ensuring the best possible protection for brands worldwide. The future of trademark surveillance will likely be a delicate balancing act, combining the speed and efficiency of AI with the nuanced judgement and understanding of human experts.
Predictive AI is changing the game in trademark surveillance. It's more than just finding visually similar logos; these systems can delve deeper, analyzing how trademarks are positioned within their markets and even predicting potential brand confusion. The key here is that it's not just about the images anymore. It's about understanding the context and trends that might lead to problems.
Think of it like this: Imagine you're trying to spot a rare bird in a vast forest. You could wander aimlessly, hoping to stumble upon it, or you could use a system that analyzes bird patterns and habitat preferences to guide you directly to the location. Predictive AI does a similar thing for trademarks – it analyzes data patterns to pinpoint potential conflicts.
This is a huge shift from traditional methods, which relied on manual searches and often only reacted after infringement had already occurred. Predictive AI allows for proactive measures, like identifying potential issues early on and adjusting brand strategies before any legal battles ensue. It can even predict the likely outcome of trademark disputes based on historical data, giving companies a real edge in their legal planning.
While these tools are incredibly powerful, it's essential to remember that they are just tools. They rely on human expertise to interpret the complex nuances of trademark law. But with the right blend of human insight and AI analysis, we can create a system that protects brands with far greater speed and accuracy than ever before.
AI-Powered Trademark Image Similarity Revolutionizing the Search Process in 2024 - Generative AI Opening New Avenues for Similarity Detection
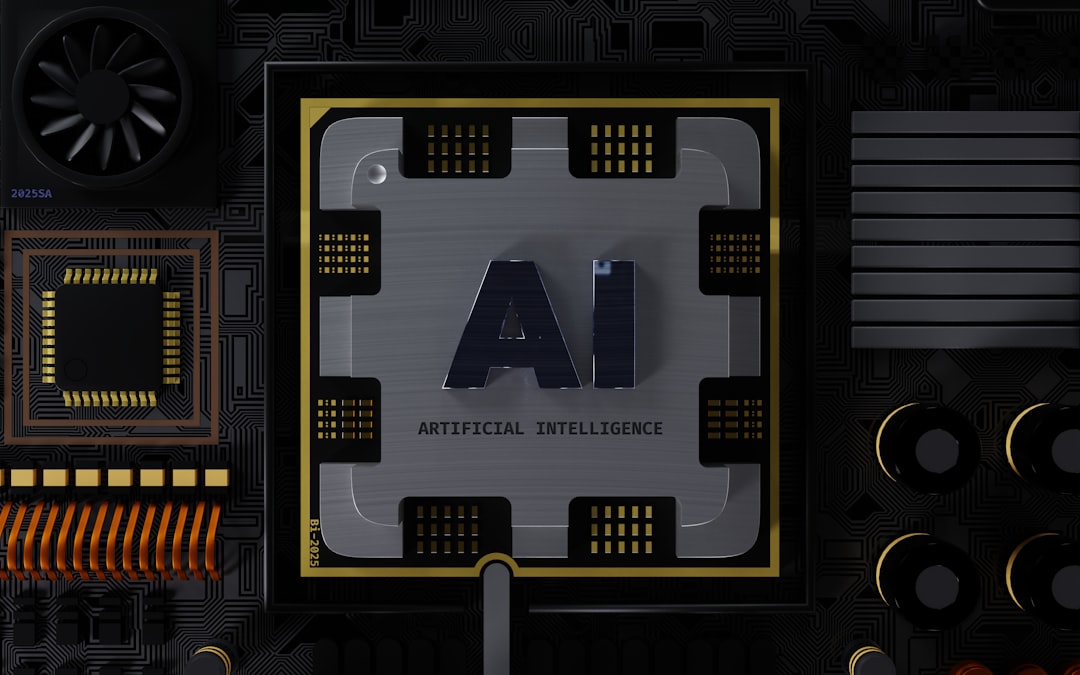
Generative AI is emerging as a powerful tool for detecting similarities, especially in the realm of trademark images. By leveraging advanced vector search capabilities, these technologies go beyond simple visual comparisons, enabling more nuanced and efficient analysis of trademark likenesses. We are seeing a rapid rise in generative AI adoption, with organizations increasingly relying on these systems for trademark management. This shift is driven by the technology's ability to provide real-time monitoring and context-aware analysis, allowing brands to proactively protect their identities in a rapidly changing marketplace. However, while these tools offer significant potential, concerns about data bias remain a critical concern, and human expertise continues to be vital for navigating the complex legal intricacies of trademarks.
The way generative AI is changing similarity detection is pretty intriguing. It's not just about analyzing existing data, but about creating new insights from it.
For example, these AI models can tap into a wider range of information, like what people are saying about trademarks on social media, giving a much deeper understanding of how they are perceived in different contexts. They can also combine visual information with text, making comparisons more nuanced and detailed.
It's not just about static analysis either. Generative AI can adapt to changing trends and cultural shifts, making sure the insights remain relevant over time. This could be really helpful for trademark owners as they navigate a dynamic marketplace.
One fascinating application is the use of augmented reality. Imagine being able to see how your trademark looks in various real-world scenarios, which could help spot potential conflicts early on.
And then there's the ability to simulate different market situations. These AI models can forecast how a trademark might evolve or be perceived, giving companies a better understanding of potential issues before they arise.
This whole field is constantly evolving. It's amazing to see how these AI models are being trained using synthetic data, making them more robust and accurate, especially for those less common trademarks where traditional methods struggle.
One of the coolest aspects is how these models create embedding spaces. It's like taking complex trademark data and compressing it into a simpler form, enabling faster and more efficient comparisons.
I'm particularly interested in how generative AI is tackling cross-language analysis, taking into account linguistic nuances and potential confusions across different regions. It's crucial as branding becomes increasingly global.
There's also this interesting aspect of trial-and-error learning. Generative AI models refine themselves through experience, continuously learning and getting better as they encounter new information.
The future of similarity detection seems to be shifting towards this more dynamic and adaptive approach, which is both exciting and a little daunting, to be honest.
AI-Powered Trademark Image Similarity Revolutionizing the Search Process in 2024 - Large Language Models Improving Clearance Processes
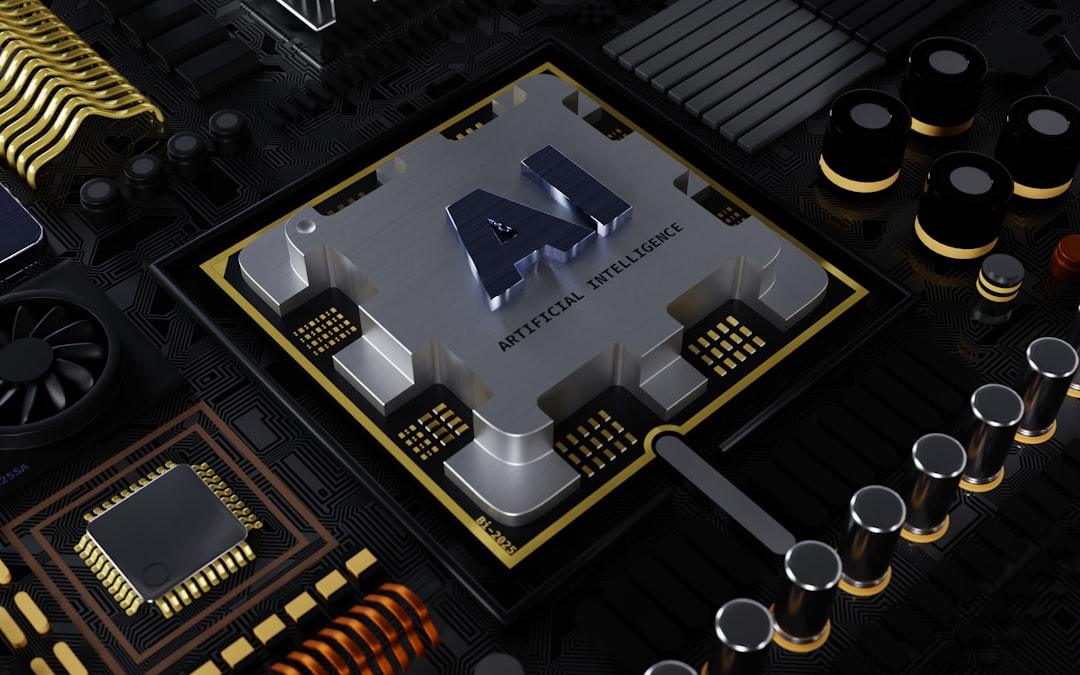
Large Language Models (LLMs) are changing how trademarks are cleared. These powerful AI tools can sift through mountains of information to help find potential conflicts with existing trademarks. They are very good at understanding language, which makes them ideal for analyzing trademark documents and even generating reports. This should mean less time and effort for trademark lawyers, but it also means more efficient and accurate results.
However, it’s not all smooth sailing. There are worries about bias in the training data that these models use. They might miss important details or even make mistakes, so human experts are still needed to review the results and make final decisions. LLMs will probably keep getting better, but they won't completely replace human judgment in the complicated world of trademarks.
Large language models (LLMs) are becoming increasingly popular due to their impressive performance in various natural language tasks, with ChatGPT being a prime example. These models rely on billions of parameters trained on massive datasets to achieve broad language understanding and generation capabilities, reflecting the scaling laws observed in AI. The way we interact with LLMs is key - we can define tasks and directly communicate our requirements for outputs like research papers or even just brainstorming. This is leading to advancements in trademark search processes, which are becoming more efficient, accurate, and cost-effective.
LLMs are powered by deep learning algorithms, enabling them to interpret and generate human language at scale. This has massive implications for intellectual property, where we're seeing a move away from traditional clearance processes and towards a more automated approach. The term "large" in "large language models" speaks volumes - it's not just about the sheer number of parameters, but also the volume of training data and the complexity of tasks they can handle. We are even seeing the development of evaluation frameworks like LLMScore to assess text-to-image synthesis effectiveness, ensuring alignment with human judgments.
Vision language models (VLMs) are emerging as powerful tools for image classification, combining text encoders and image encoders to leverage the strength of LLMs in this domain. It's fascinating to see how these technologies are being integrated into various processes, including trademark clearance and image similarity searches. By 2024, we can expect a revolution in these fields, leading to more straightforward and reliable methodologies.
Here are ten surprising facts regarding how large language models can enhance clearance processes in trademark management:
1. **Real-time Data Processing**: LLMs can analyze legal texts and trademark databases in real-time, offering instant insights into existing trademarks and potential conflicts, drastically cutting down the time required for traditional searches.
2. **Semantic Understanding**: These models can comprehend nuances in legal language and context, allowing for a deeper understanding of trademark distinctiveness. This goes beyond mere keyword searches, targeting the essence of trademark usage and perception.
3. **Predictive Analytics**: By leveraging historical trademark data, LLMs can predict likely disputes before they occur. This proactive approach helps brands adjust their strategies early, potentially avoiding lengthy legal battles.
4. **Multi-Language Proficiency**: These models are capable of analyzing trademarks across multiple languages and cultures, understanding linguistic nuances that standard algorithms might miss. This capability is crucial for brands operating in diverse markets.
5. **Integration with Other AI Technologies**: Language models can complement visual similarity detection tools by providing contextual analysis, which helps assess potential infringement risks that hinge on both visual and textual elements.
6. **Bias Mitigation Strategies**: Advances in large language models include implementing bias detection and mitigation strategies, making the tools more equitable and reflective of diverse legal interpretations, which is essential for fair trademark assessments.
7. **Continuous Learning**: These models are designed to continuously learn from new case law, evolving industry standards, and trademark trends, enhancing their accuracy over time as they adapt to the changing legal landscape.
8. **Enhanced Collaboration Tools**: Language models facilitate better communication between legal teams by summarizing complex trademark cases or legal documents into digestible formats, streamlining collaboration and decision-making processes.
9. **Scenario Simulation**: They can simulate various trademark dispute scenarios and their potential outcomes, enabling firms to strategize better and make informed decisions based on predictive analytics.
10. **Support for Smaller Entities**: By reducing the need for extensive legal resources, large language models empower startups and small businesses with affordable insights into trademark disputes, leveling the competitive playing field against larger firms.
It's worth noting that while LLMs offer immense potential for the future of trademark clearance, there are still challenges to address. One concern is the possibility of biases influencing the output, as LLMs are trained on massive datasets which may contain inherent biases. However, ongoing research into bias detection and mitigation strategies is paving the way for more equitable and reliable outcomes.
Overall, these transformative aspects underline the importance of integrating advanced AI capabilities into trademark clearance processes, pushing the boundaries of what's achievable in legal contexts. It's an exciting time to be researching and developing these tools, as they hold the potential to streamline and enhance how we protect trademarks.
AI-powered Trademark Search and Review: Streamline Your Brand Protection Process with Confidence and Speed (Get started for free)
More Posts from aitrademarkreview.com: