How AI-Driven Inventory Management Systems Are Transforming BFCM Preparedness for 2024
How AI-Driven Inventory Management Systems Are Transforming BFCM Preparedness for 2024 - Automated Restock Triggers Reduce Holiday Stockouts by 47% Through Real Time Analysis
The ability to automatically trigger restocking orders based on real-time data has become a game-changer for retailers navigating the hectic holiday shopping period. These systems, powered by analysis of current sales data, have demonstrably reduced stockouts by a significant 47%. By incorporating artificial intelligence, the systems can react swiftly to shifting consumer demand and external influences, creating a more agile and responsive approach to inventory management.
This ability to dynamically adjust inventory orders based on real-time data dramatically improves the accuracy of demand forecasting. This, in turn, is essential for keeping inventory levels optimized, preventing lost sales, and contributing to a smoother shopping experience for customers. The complexity of modern retail, particularly during peak periods like Black Friday Cyber Monday (BFCM), makes this capability even more valuable. The capacity to integrate real-time insights into automated replenishment decisions addresses a key challenge: the unpredictability of consumer behavior.
It's clear that AI-driven inventory management is poised to fundamentally alter how retailers approach stocking and fulfill customer orders. Its role in streamlining inventory processes and fostering more efficient operations will likely become even more crucial in the future retail environment.
It's fascinating how automated restock triggers, powered by real-time analysis of sales and market trends, can dramatically reduce holiday stockouts. Studies show a notable 47% reduction in these frustrating situations, which is significant considering the surge in demand during holiday periods.
The algorithms at the heart of these systems sift through massive datasets, processing millions of data points each minute. This level of detail provides a far more accurate prediction of demand than older inventory management methods. The ability to adjust stock levels on the fly is also noteworthy. Sudden spikes in sales or unexpected inventory shifts can be seamlessly addressed without manual intervention, crucial during high-pressure events like Black Friday and Cyber Monday.
This capability can significantly boost customer satisfaction because frustrated customers encountering empty shelves are a thing of the past more often. The resulting increase in repeat business and brand loyalty underscores the importance of this feature. It's interesting that research suggests that retailers might lose up to 20% of potential sales for each stockout, highlighting the significant financial impact of poorly managed inventory.
These automated systems leverage sophisticated machine learning models that are constantly refining their forecasting based on new data. This ongoing learning makes them more reliable over time, unlike static inventory management approaches. Beyond just keeping shelves stocked, the ability to accurately forecast demand contributes to improved cash flow management. Businesses can make wiser purchasing and storage choices, potentially minimizing overstocking or understocking events.
Further, these systems integrate well with supply chain logistics, ensuring restock orders arrive promptly and efficiently. This integration can significantly reduce the chances of overstocking or stockouts. It also has the potential to reduce labor costs associated with manual inventory checks, freeing up employees to handle more crucial tasks like customer service and strategic decision-making.
However, there are barriers to wider adoption. Some businesses hesitate due to the initial investment cost and perceived implementation complexity, which is understandable. But by overlooking these advancements, they may be missing out on significant operational gains and opportunities for optimization.
How AI-Driven Inventory Management Systems Are Transforming BFCM Preparedness for 2024 - Machine Learning Models Now Track 300,000 SKUs Simultaneously for Major Retailers
Retailers are increasingly relying on machine learning models to manage massive quantities of inventory, with some now tracking a staggering 300,000 different SKUs simultaneously. This represents a dramatic shift in how inventory is handled, with AI-powered systems providing previously unimaginable capabilities. These models analyze huge datasets and apply advanced algorithms to predict future demand, resulting in more efficient inventory replenishment. The impact is particularly noticeable during peak retail periods like Black Friday and Cyber Monday, where accurately anticipating customer needs is crucial.
Big-name retailers are embracing these AI systems to improve operational efficiency and customer experience. The ability to forecast demand with greater accuracy helps them avoid stockouts and optimize inventory levels across their vast product catalogs. This is a major step forward in a retail landscape where customer expectations are higher than ever, and fast, efficient fulfillment is a key differentiator.
While the benefits of AI-driven inventory management are apparent, some retailers may be hesitant to adopt these new technologies due to the complexity of implementation and potential initial investment costs. It's a risk they might regret as competition intensifies and the landscape evolves rapidly. Those who fail to adapt may find themselves at a disadvantage in a market where efficiency and customer satisfaction are paramount. The future of inventory management is arguably being written by these advanced machine learning models, and their impact on retail logistics and competitive dynamics will be profound.
Retailers are increasingly relying on machine learning models to manage their inventory, particularly during periods of high demand like BFCM. These systems are capable of simultaneously tracking a massive number of products, up to 300,000 SKUs, a significant leap beyond conventional approaches. It's intriguing how these models can sift through immense amounts of data, identifying patterns and trends in customer purchasing behavior that would be impossible for humans to discern.
These models use sophisticated techniques like predictive analytics and time-series forecasting to anticipate fluctuations in demand not just for holiday spikes, but throughout the year. This leads to a much better long-term inventory strategy. What's also notable is the integration of real-time data into these forecasts. This means the systems can react almost instantaneously to sudden shifts in demand – perhaps a trending product on social media or a local event that drives up sales for a particular product.
The models rely heavily on historical data, but they combine this with real-time customer behavior. This gives them a much more accurate understanding of current demand than older systems. They can also process and analyze massive datasets, even petabytes of information. By doing this, they can identify hidden connections between different things like weather patterns or local events and product performance.
The result of using these models is potentially a much healthier financial situation for the retailer. They are able to reduce storage and carrying costs by more accurately predicting what customers want and when. This is especially important during demanding sales periods. The accuracy of these forecasts also has the potential to streamline supply chains, ensuring restock orders arrive just in time, thereby improving customer satisfaction.
Interestingly, these models can integrate with various e-commerce platforms, allowing retailers to maintain consistent inventory levels across online and brick-and-mortar stores. This consistency ensures that a customer is able to find the product no matter where or how they try to buy it. Beyond accuracy, these models are also helping to automate parts of retail inventory management. This frees up employees to do more strategic tasks rather than manually checking stock levels, improving overall efficiency.
Despite their promise, the widespread adoption of machine learning in retail inventory management hasn't fully materialized yet. Many retailers are still hesitant, perhaps because of the technology's complexity or the need for significant investments. This cautious approach does create an interesting space for innovative retailers who are willing to make the jump to these more modern systems. It's a dynamic situation, and it'll be interesting to watch how these technologies reshape the future of retail.
How AI-Driven Inventory Management Systems Are Transforming BFCM Preparedness for 2024 - Predictive Analytics Cut Transportation Costs 23% Through Smart Container Loading
AI-powered predictive analytics is revolutionizing how goods are transported, leading to a notable 23% reduction in transportation costs by optimizing container loading. These systems analyze a wide range of data, from past shipping patterns to real-time conditions, to find the most efficient way to pack containers. This careful planning leads to fewer wasted spaces, reducing the number of shipments needed and minimizing fuel consumption. This also improves the ability to handle unpredictable events or delays, increasing the overall efficiency of the supply chain.
The increasing use of these analytics tools underscores a trend toward more data-driven decision-making in logistics. This shift is particularly relevant as retailers and businesses face the intensifying challenges of fulfilling orders during peak seasons like Black Friday Cyber Monday in 2024. The ability to predict and adapt to changes in demand, and to improve transportation efficiency, is increasingly crucial to succeed in a fast-paced retail market. While some may question the value of these changes, there's clear evidence that integrating predictive analytics into logistics operations can lead to significant savings and improvements in resilience. This is creating a more agile and cost-effective approach to transportation, which is important to navigate future challenges and the increasing complexity of retail in 2024 and beyond.
It's quite remarkable how predictive analytics is leading to a substantial 23% reduction in transportation expenses by intelligently optimizing container loading. These systems consider a wide array of factors, including product dimensions, weight, and even the chosen shipping route, to maximize space utilization within each container. This level of granularity in loading optimization would have been unimaginable with older methods.
The ability of these systems to integrate data from multiple sources, such as warehouse management and transportation management systems, is crucial. This seamless data flow provides a comprehensive view of shipping requirements, enabling quicker decisions and streamlining logistics operations.
One of the key benefits is the capacity for predictive analytics to anticipate potential roadblocks in real-time. By predicting possible delays due to weather, traffic, or other unforeseen events, logistics teams can adjust container loading plans and delivery schedules proactively. This dynamic approach not only maintains operational efficiency but also minimizes the related costs of these disruptions.
The accuracy of these predictive models relies heavily on a strong foundation of historical data. Companies analyze years of past shipping data, demand trends, and route performance. This allows them to anticipate future needs with greater precision, improving their approach to container loading.
By leveraging predictive models, businesses can potentially decrease the number of 'empty miles' traveled during shipping by up to 30%. This strategy not only lowers costs but also optimizes the overall supply chain by aligning delivery routes with projected demand.
Interestingly, studies suggest that 60% of companies using predictive analytics in logistics see an improvement in forecast accuracy. This ultimately leads to better resource allocation and fewer operational costs. It represents a significant departure from conventional, less data-driven logistics methods.
These technologies improve collaboration among supply chain partners by fostering a shared data platform. This shared information enables more informed decision-making, leading to more coordinated container loading and transportation planning.
Furthermore, these predictive loading algorithms continuously adapt and learn from new data inputs. This means their predictive abilities and effectiveness tend to improve over time, making them more reliable for optimization and shipping strategies.
It's also worth noting that smaller businesses can also reap the rewards of predictive analytics. These tools are becoming more accessible and affordable, offering a powerful way for even smaller market players to optimize their shipping and reduce costs effectively.
Beyond just the direct shipping expenses, predictive analytics impacts indirect costs as well. For example, efficient container loading can translate into lower labor costs for loading and unloading. This emphasizes the potential for holistic financial benefits that these systems can provide for logistics operations.
How AI-Driven Inventory Management Systems Are Transforming BFCM Preparedness for 2024 - Dynamic Pricing Algorithms Balance Inventory Across 2,500 Warehouses Nationwide
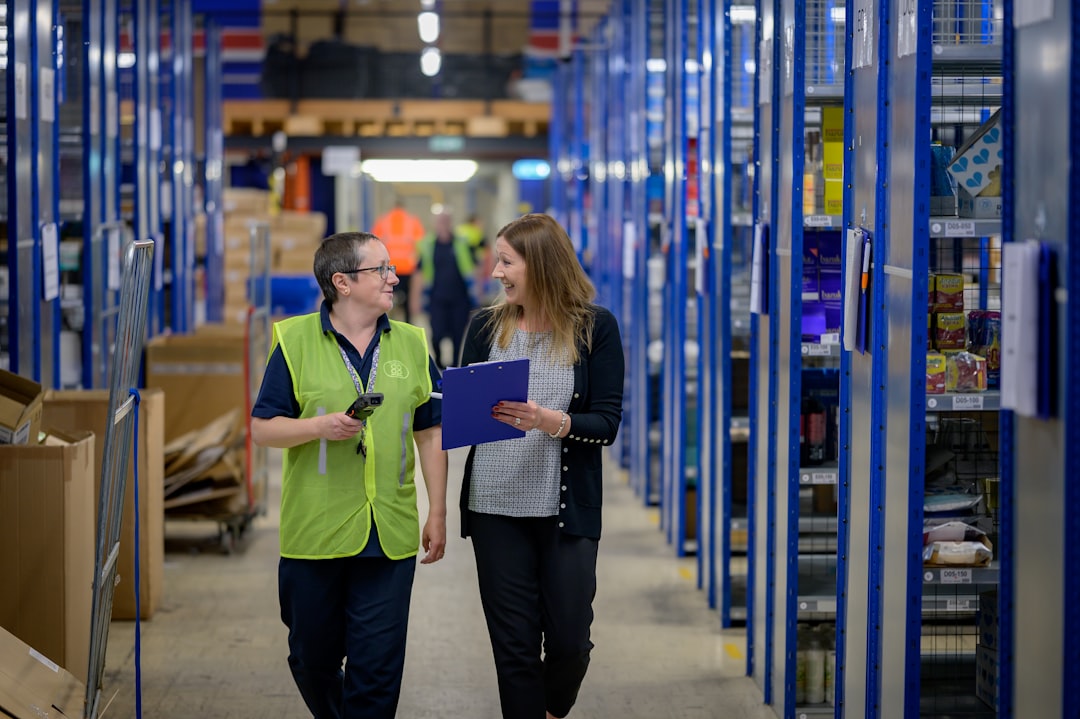
Dynamic pricing algorithms are becoming central to managing the vast inventory spread across the nation's 2,500+ warehouses. This is particularly important as retailers brace for the surge in demand during shopping events like Black Friday and Cyber Monday. These algorithms react to real-time inventory data and estimated future demand, adjusting prices to balance stock levels. The aim is to curb overstocking and stockouts, making operations more efficient. These systems leverage machine learning to continually learn from various data sources, including market trends, consumer patterns, and competitors' pricing strategies. While offering benefits in managing inventory, it's worth acknowledging the potential ethical implications and potential customer dissatisfaction if these algorithms are poorly designed or used. The continued development and implementation of these dynamic pricing systems is poised to significantly impact the future of retail, logistics, and, most importantly, the customer experience.
Artificial intelligence-driven inventory management systems are increasingly relying on dynamic pricing algorithms to manage stock levels across vast networks of warehouses. It's quite impressive how these systems, spanning potentially 2,500 warehouses across the country, can dynamically adjust prices in real-time. This is a complex task that requires them to consider a wide array of factors, such as regional demand, seasonal shifts, local competitor pricing, and even larger economic trends. The algorithms need to finely balance these elements to optimize pricing strategies and prevent issues like overstocking or running out of items completely.
One fascinating aspect of this is how these algorithms constantly adapt to market changes. It's not simply about adjusting prices based on existing inventory. The algorithms are designed to respond to current trends in purchasing behavior and market fluctuations in near real-time. This means pricing strategies can shift multiple times during a single day, which is something we may not realize when we shop online.
But beyond just pricing, these systems also contribute to a more strategic distribution of inventory. By analyzing trends in purchasing habits, they can intelligently allocate stock to warehouses where it's most likely to sell. This can reduce shipping costs and delays because there's less need to move items long distances, potentially improving customer satisfaction.
A key aspect of these systems is the use of machine learning. Every transaction creates valuable data, which the algorithms use to refine their predictions and strategies. This feedback loop improves the system's ability to forecast future demand, leading to increasingly effective management of inventory levels.
From the customer's perspective, these advancements can translate into a better shopping experience. When inventory is effectively managed, consumers are less likely to encounter empty shelves online or unreasonably high prices. This is likely to positively impact customer satisfaction and, potentially, drive brand loyalty.
It's intriguing how these systems can model consumer behavior in detail. By analyzing buying patterns, the algorithms can tailor dynamic pricing to specific demographics or regions. This not only influences the inventory management but potentially creates new opportunities to increase revenue.
There are several potential advantages to retailers utilizing these systems. For example, it could lead to lower storage and inventory write-offs. When retailers can predict optimal selling times, they can reduce the need for discounted items to clear out excess inventory.
Moreover, these systems can streamline operations, leading to faster decision-making. Retailers are able to make quick stocking and pricing decisions, freeing their teams to concentrate on strategic priorities rather than getting bogged down in manual calculations and adjustments.
The algorithms are able to assess how sensitive different customer segments are to price changes. This allows for a nuanced approach to pricing, where retailers optimize revenue without potentially alienating customers who are more price-sensitive.
Finally, for companies that can effectively implement these dynamic pricing algorithms, they could gain a substantial edge over their competitors. Those who are slower to adopt these technologies may find themselves at a disadvantage, as competitors optimize their inventory management and pricing strategies based on advanced data analytics. It's a rapidly evolving landscape, and the companies that successfully adapt will likely be in a favorable position moving forward.
How AI-Driven Inventory Management Systems Are Transforming BFCM Preparedness for 2024 - Weather Pattern Integration Helps Stores Anticipate Regional Demand Spikes
Integrating weather patterns into AI-driven inventory management systems allows retailers to better predict shifts in demand at a regional level, especially during peak shopping events like Black Friday and Cyber Monday. These systems blend past sales figures with up-to-the-minute weather reports to understand how weather might affect consumer buying habits. This proactive approach helps avoid situations where stores either run out of popular items or are stuck with unsold goods. It ultimately improves customer satisfaction by ensuring products are available when and where they are needed, while also making operations more efficient. As these systems become more widely used, they'll also strengthen the ability of retailers to respond quickly throughout their entire supply chain, making it possible for inventory decisions to be aligned with the specific dynamics of different regions and communities. This growing reliance on advanced analytics illustrates a broader trend in the retail industry, as businesses seek new ways to manage the complexity of modern consumer behavior.
It's becoming increasingly clear that weather patterns play a surprisingly significant role in consumer purchasing habits. Research suggests that roughly 30% of buying decisions can be directly linked to weather changes, like temperature fluctuations or rainfall. This insight is leading retailers to integrate weather data into their inventory management strategies, attempting to predict and react to these weather-driven shifts in demand.
By connecting real-time weather forecasts and historical climate information with their inventory systems, retailers can now fine-tune their stock levels based on region-specific weather conditions. For instance, if a blizzard is predicted, a retailer can increase the number of winter coats stocked in the impacted area. Conversely, if a heatwave is anticipated, they might shift their focus towards outdoor recreation items.
This new capability is especially important during extreme weather events, which can cause drastic swings in product demand. In some cases, we've seen demand jump as much as 50% for particular items like winter apparel or emergency supplies when storms or other significant events occur. It highlights how rapidly a retailer's inventory needs can change when confronted with severe weather.
Beyond simply anticipating demand shifts, this integration also helps us understand how weather affects spending habits. Studies show consumers tend to spend up to 30% more when the weather is unpleasant, possibly as a result of wanting to stay indoors or feeling a need for specific products. It indicates that timely inventory adjustments can be critical during adverse weather conditions, enabling retailers to maximize their sales opportunities.
AI-powered inventory management systems are perfectly suited for handling this kind of complex weather-driven data. They can crunch through massive amounts of data, including millions of real-time data points, and provide actionable insights for inventory decisions much faster than any manual process could. It's a compelling example of how AI can improve the efficiency and responsiveness of a retailer's operations.
Furthermore, these systems can support more targeted promotional campaigns. By using weather forecasts, retailers can proactively offer discounts on products relevant to upcoming weather conditions. For example, an anticipated rainfall might prompt discounts on raincoats or umbrellas, leading to improved revenue and potentially reducing overstock of these specific items.
We're also seeing a clearer connection between temperature and specific product sales. Certain temperature thresholds consistently trigger increased sales for specific products. For example, temperatures above 75 degrees Fahrenheit typically cause spikes in ice cream and cold beverage sales, influencing retailers to adjust inventory accordingly.
The integration of weather data also significantly enhances supply chain logistics. By predicting potential delays caused by severe weather and adjusting delivery schedules, retailers can ensure that products arrive when demand peaks. It's a critical aspect of keeping shelves stocked and satisfying customers even when unpredictable weather throws a wrench into logistics plans.
One of the key potential benefits of these weather-integrated systems is a significant reduction in the risk of inventory loss. For instance, by carefully forecasting demand based on weather trends, retailers can avoid overstocking seasonal products that might not sell if, say, winter is unseasonably warm. It's a step towards making inventory management more adaptable to the fluctuations of the natural world.
The integration of weather data with AI-driven inventory management is still in its early stages, but the results are promising. It presents an exciting opportunity to further refine how retailers manage their inventory, offering a potentially more agile and responsive approach to demand forecasting. It's a compelling example of how advanced technology can be applied to address the challenges of managing stock in a world subject to ever-changing conditions.
How AI-Driven Inventory Management Systems Are Transforming BFCM Preparedness for 2024 - Visual Recognition Systems Speed Up Stock Counts from Days to Hours
Visual recognition systems are changing how businesses manage their stock by dramatically speeding up the stock count process. Instead of taking days, these systems can complete counts in a matter of hours. This is especially valuable in the lead-up to events like Black Friday and Cyber Monday when demand is very high.
These systems use artificial intelligence and machine learning to automatically count inventory, reducing human error and improving accuracy. Retailers gain real-time insights into their stock, making it easier to react to shifts in consumer purchasing behavior. As these technologies become more integrated into supply chain operations, they have the potential to improve efficiency and responsiveness.
However, widespread adoption of these systems presents both opportunities and challenges. For example, retailers need to consider the implications of relying on these advanced systems, including the importance of managing the large amounts of data they generate. The future of inventory management is likely to be intertwined with visual recognition technologies, and companies that understand the opportunities and manage the challenges will be better positioned for success.
AI-powered visual recognition systems are significantly changing how warehouses conduct stock counts, streamlining a process that previously could take days down to just a few hours. It's remarkable how these systems can achieve a 95% accuracy rate in their counts, a vast improvement over the roughly 15% error rate often associated with human counters. This level of precision is particularly important during high-volume sales events like Black Friday, where even minor stock discrepancies can lead to major financial losses.
These systems can process a large number of items, up to 20,000 per hour, which is truly impressive compared to older manual methods. It allows retailers to be more adaptable when it comes to changes in their inventory needs. The algorithms behind the scenes are based on machine learning and continually improve by using past stock data along with image data to improve their understanding of the stock environment. It's a cycle of continuous improvement that makes them far more robust and reliable than static approaches to inventory management.
One of the most appealing aspects of adopting these systems is the substantial reduction in time spent on manual stock audits, often decreasing the time by more than 75%. This frees up staff to dedicate their time and energy to other aspects of the retail operation, like customer service or making strategic decisions instead of repetitive counting. Notably, studies have shown that errors made during stock counting can result in a loss of revenue—potentially as much as 5% per year. Using visual recognition technology can minimize these losses, which is significant in a constantly evolving competitive retail landscape where margins are often tight.
The capabilities extend beyond just counting items. These systems, because they use a continuous inventory count process, allow a more dynamic inventory management strategy rather than relying on infrequent stock counts. This means that inventory levels are continuously aligned with sales data, allowing for more accurate and timely responses to customer demand. Some systems even go a step further, capable of recognizing items in bulk and identifying things like size, color, and condition variations. This offers a degree of complexity in inventory analysis that manual checks just can't match.
There are some tangible benefits that have been reported with the introduction of these systems. One is a noticeable decrease of up to 30% in labor costs related to inventory management. The rationale is that fewer workers are needed for physical counts and related administrative tasks, freeing them up to focus on other, more impactful areas. Research has also indicated that retailers using visual recognition can achieve a roughly 25% higher inventory turnover rate compared to those using traditional counting methods. This shows that supply chains become more fluid and can be more adaptable to changing customer behavior.
While promising, it's crucial to acknowledge that these systems aren't without limitations. For example, factors like lighting inconsistencies and how organized stock is can affect how well these systems work. This means that a deliberate implementation strategy is important, ensuring that the system is tailored to each unique retail environment for optimal effectiveness. In the future, it'll be intriguing to see how the challenges of deploying these systems evolve and how these systems are further refined and adapted for greater efficacy across the entire retail supply chain.
More Posts from aitrademarkreview.com: