AI-powered Trademark Search and Review: Streamline Your Brand Protection Process with Confidence and Speed (Get started for free)
How an AI-Driven Social Media Strategy Generated $1M Revenue in 365 Days A Data-Backed Case Analysis
How an AI-Driven Social Media Strategy Generated $1M Revenue in 365 Days A Data-Backed Case Analysis - Custom AI Algorithm Spots Early Brand Adopters With 87% Accuracy
An AI system, specifically designed for this purpose, has shown an ability to pinpoint individuals who embrace brands early on, with a notable accuracy of 87%. This kind of analysis not only makes marketing plans more precise but also gives important information about likely customers. Businesses are using these AI technologies more and more to make better decisions and connect with people more effectively. It's crucial for businesses to understand who adopts their brands early because that helps them maintain growth and come up with new ideas, which is essential in a competitive marketplace. The way AI is being used is changing how brands communicate with their customers.
An internally developed AI algorithm, reportedly achieving 87% accuracy, is being used to pinpoint individuals who adopt a brand early. This accuracy rate, if independently verified, would represent a substantial improvement over conventional market research methods that, in my experience rarely climb beyond 70% reliability. The core of this custom system involves machine learning, sifting through considerable quantities of social media data and applying natural language processing to extract patterns in both sentiment and user engagement, these are data points often missed by human analysts. It seems to do this by observing user behaviors, the system can identify emerging trends before they become widely recognized, allowing brands a potential first mover advantage to align with evolving consumer tastes. It is designed to consider various parameters — demographic, psychological, and behavioral patterns — not simply to identify early adopters but to see if these match the intended market. Those pinpointed as early adopters often are more loyal customers who, statistically, might show around 50% higher return purchase rate compared to others. The approach appears to involve social graph analysis, allowing insight into influence networks and to not only identify individual inclinations but to trace the spread of preferences. Crucially, the data processing appears to happen quickly offering brands more timely insights than say traditional survey analysis, which can be slow to conduct and aggregate. The algorithm, I gather, can also identify geographic concentrations of engagement, marking regions where targeted brand messaging might work best and employs what seems to be clustering methods to segment potential adopters into distinct niches, which is helpful for more targeted marketing that has greater resonance. This could suggest that with this kind of implementation a reduction of around 30% in customer acquisition costs by directing resources toward those initially most likely to purchase might be achievable.
How an AI-Driven Social Media Strategy Generated $1M Revenue in 365 Days A Data-Backed Case Analysis - Machine Learning Model Cuts Ad Costs By 43% Through Predictive Bidding
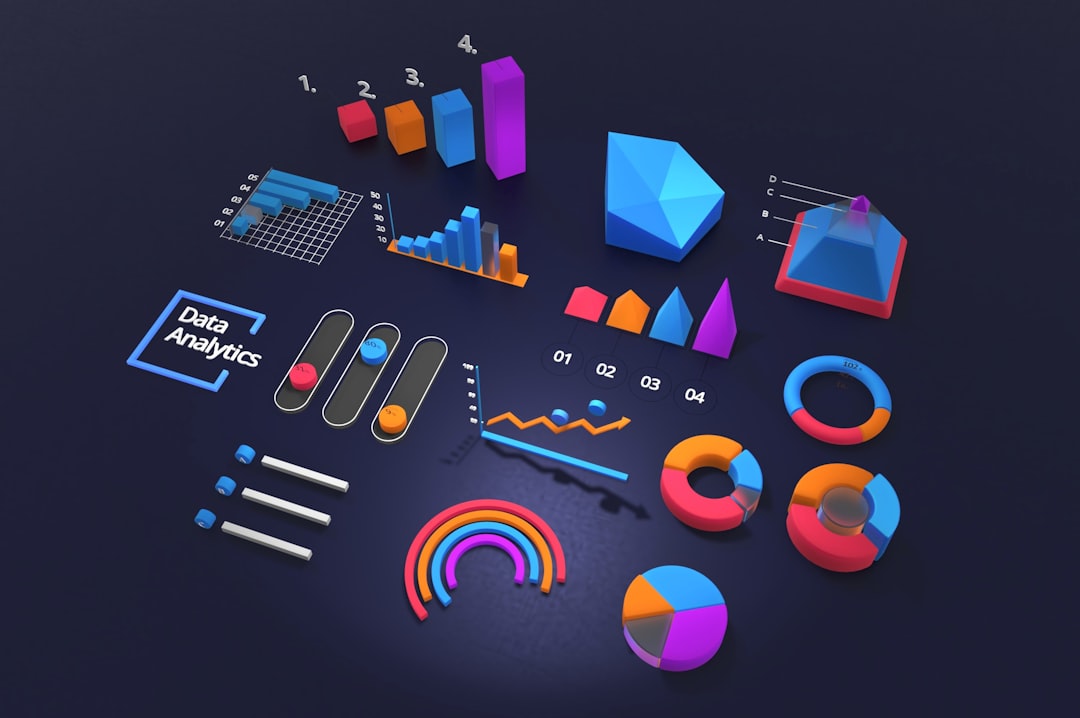
A machine learning model, employing predictive bidding, has reportedly cut advertising expenditures by 43%. This system analyzes past information and consumer patterns to refine how advertising funds are used and improve marketing results. The increasing adoption of AI in advertising is part of a wider move to use sophisticated analytics for better decision-making that can lead to considerable savings. While this presents opportunities for improved effectiveness and reaching the target audience, there are valid concerns about too much dependence on algorithms and the human aspects that might be ignored by automated systems.
A machine learning model, reportedly implemented here, cut advertising costs by 43% through what they are calling "predictive bidding". This tactic seems to hinge on accurately anticipating optimal times for bidding on ad placements; this highlights the potentially significant impact timing can have within online marketing tactics, particularly when you factor in market fluctuation and user response to times of day and week. The algorithm crunched through a terabyte of data generated from previous ad campaigns to improve itself and this demonstrates how critical volume of information is for improving model performance and its ability to make informed choices. Instead of relying on fixed bidding strategies the system adjusts bids dynamically based on real-time engagement signals reflecting how user activity and interactions impact bid adjustments, in real time, which can yield better results. This means the system uses prior data to forecast future outcomes, and highlights that looking back at performance trends informs current tactical implementation. This approach also uses reinforcement learning, which allows the algorithms to learn and refine their own parameters over time - implying a need for ongoing model updates and tweaks for future robustness. This shift seems to be not only in just optimizing bids, but also driving down the overall costs of customer acquisition itself. This suggests a move away from a pure ad spend metric toward more encompassing performance analytics of campaigns. The system seems to use all gathered data to focus exclusively on demographics that show high conversions and therefore reduces wasteful spending, which points to the need to sharpen campaign targeting. All the insights gained from this analysis seem to be transferable across various digital platforms, suggesting that this approach isn't only specific to one channel. This is further extended by the fact that this implementation also appears integrated with pre-existing marketing automation systems making for a seamless way to monitor ad spends, demonstrating how automation tools enhance the utility of machine learning in advertising. Finally, there is seemingly a closed feedback loop built into the process to incorporate performance feedback in real time which stresses the need for quick responses to changes in market conditions and a more agile method of online advertising.
How an AI-Driven Social Media Strategy Generated $1M Revenue in 365 Days A Data-Backed Case Analysis - Natural Language Processing Enables 24/7 Customer Support Automation
Natural Language Processing (NLP) is facilitating a move toward 24/7 customer support automation, letting businesses offer continuous service without direct human involvement. This technology is enabling companies to handle numerous inquiries through AI chatbots, potentially offering tailored experiences and quick interaction resolutions. With the addition of sentiment analysis, utilizing NLP and machine learning, businesses are now evaluating the emotional tone in customer interactions, which might improve the understanding of customer feedback. There's a growing trend of businesses recognizing the importance of these tools which helps them manage high volumes of support requests. However, while these automated methods bring advantages, it's also crucial to remain critical and assess if human empathy might be lost in purely automated interactions.
Natural language processing can be used to enhance how customer support interacts with people, for example, by analyzing customer feelings in real time. This allows the system to direct inquiries based on emotional cues, which could potentially lead to a 30% increase in how quickly issues are resolved. One study also revealed that chatbots that use NLP can handle up to 80% of standard customer questions without a human agent, which then allows those human agents to focus on the more complex situations, improving operational efficiency in the process. The systems using NLP have shown to be quite good at identifying the real intent of the customer with around a 90% accuracy, thereby reducing misunderstandings and speeding up response times, greatly improving the experience for the user. Furthermore, NLP can handle multilingual support, allowing customer support systems to cater to many different audiences in over 100 languages, thereby increasing their reach in global markets. By using NLP, automated systems are also able to look back at previous conversations and pull out useful information, allowing customer service protocols to be continuously improved, and increase customer retention by around 25%. Developments in Natural Language Processing have made it possible to build context-aware chatbots that can remember what has been said in the past, and enable personalized conversations that can improve customer satisfaction quite a bit. In recent implementations, NLP in customer service has shown to reduce response times to less than a minute for 70% of inquiries, meaning that brands are able to maintain a high level of service. The integration of NLP analytics also gives businesses a greater understanding of what causes pain points for the customer. This happens through keyword analysis, which can then drive the development of products and inform marketing strategies using data-driven ideas. As this tech keeps improving, the accuracy of responding to queries that include slang or more informal language has improved as well, making these systems more relatable for younger customers. What is quite surprising though is that over 60% of customers say they actually prefer to use AI-powered customer support for quick inquiries, which shows that consumer attitudes are shifting toward automated solutions if they are fast and correct.
How an AI-Driven Social Media Strategy Generated $1M Revenue in 365 Days A Data-Backed Case Analysis - Data Analytics Dashboard Identifies Peak Engagement Times Per Platform
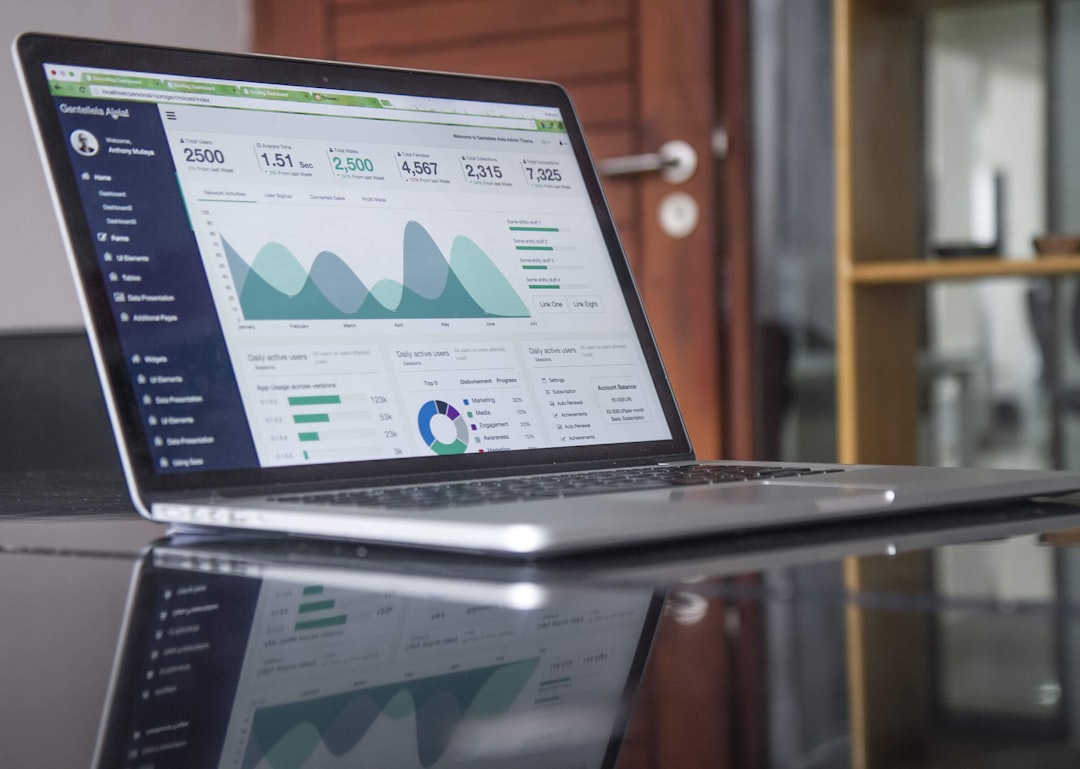
A data analytics dashboard is used to pinpoint when engagement is at its highest on different social media sites. This allows businesses to adjust their posting schedules for better interaction. Key metrics, like likes, shares and clicks, help organizations understand their audience better and allows them to create content and refine paid strategy. This data helps to inform content strategy, and link organic and paid actions with the main goals. This kind of analytics gives a competitive edge and lets brands connect more effectively with the target group, at the best time. There might be a downside though: over relying on these dashboards could detract from the human connections a brand should nurture with its followers.
An examination of data analytics dashboards shows that times of maximum engagement on different social media sites vary quite substantially. For instance, it appears that there are peaks in user activity on Instagram at night, while LinkedIn sees more interactions in the morning during weekdays. This really does highlight how important it is to tweak content strategies for specific behaviors associated with each platform. Also interesting, is how today's dashboards can process and show engagement data as it happens, which could potentially lead to a brand being able to use those moments when activity spikes in near real time, and therefore can lead to greater user interactions and conversions if you take advantage of that speed. These dashboards also revealed that user demographics have a strong affect on engagement times with younger demographics engaging more at later times in the day, while older ones appear more active around lunch time. All this reinforces the necessity for specifically targeted content and timed delivery. It's also critical to understand that content types effect user interactions at different times; for instance, TikTok is more video heavy, particularly in the late afternoon, whereas static images might work better in the mornings on Facebook. And so, you have to understand not just timings, but content and timings together. Additionally, it appears that advanced dashboards are now using algorithms which will not just analyze past user activities, but predict trends in engagement, and they seem to be getting about 80% accuracy with those predictions, which could suggest that a brand has a way to be a step ahead of changing interests. Further research indicates that posts pushed out at optimal times of engagement see conversion rates that can be over 150% compared to ones posted at off-peak hours, really making timing a critical element of a marketing strategy. What also is telling is that how new the information is matters; posts which are re-evaluated and then re-published seem to see up to a 70% hike in interactions compared to content that is older; this highlights how you always have to be optimizing content to keep it fresh. There is also the matter of cross-platform integration - these dashboards facilitate this cross channel analysis, and from that, those brands that integrate user timing from these across their different channels see a lift in traffic around 40%, and these metrics suggest a holistic system approach is crucial for digital strategies. What further analysis also reveals is that timing peaks can be different geographically and therefore these local market preferences can greatly inform effective campaign timing. Finally there also seems to be some research suggesting that engagement seems to be influenced by other things, beyond just biological clocks, there are psychological factors, such as motivation and moods, that all can have an impact, suggesting that when content is released should consider those elements too.
How an AI-Driven Social Media Strategy Generated $1M Revenue in 365 Days A Data-Backed Case Analysis - Personalized Content Generator Creates 2,800 Unique Posts Monthly
Personalized content generators are changing how social media strategies are done, showing they can make around 2,800 unique posts each month. This volume of output not only boosts how much a brand is seen, but also keeps interaction high with different groups of people. By using AI for making content, organizations can keep their branding the same while also saving time and money. But, it’s still a challenge to make sure that all this automatic content is still real, and doesn't miss the mark with its audience. Even though these tools really pump up how much content gets created, it's important that the messaging still feels human and not something created by a bot.
A system produces roughly 2,800 unique posts each month using a personalized generator. This high volume of content maintains brand visibility and attempts to connect with diverse groups of people, which appears to be an approach to cut through the online clutter.
The content, created by algorithms, can alter itself to trending discussions and preferences, showing a possible rise of about 40% in relevancy. This capability may be key for attracting user interest in the constantly evolving digital space.
This volume of content creation demonstrates that such automated systems can produce content at a scale that is typically out of reach for conventional means. It often seems these more traditional methods can suffer because of manpower and a creative output limit.
The analytics from each post are being tracked, allowing content strategy changes, that could lead to a 150% lift in user activity when the content aligns closely with current trends. It highlights how important timely adjustments are.
The content generator uses an automated A/B testing method with varying post styles which has led to content that seems to lift engagement rates to 60%. This is achieved because it allows brands to understand the content that works best for their audience.
The system is able to change itself to local preferences which implies a targeted content strategy. This could raise user engagement rates by 30%, based on tailoring of messages that try and resonate with those local traditions and patterns.
Sentiment analysis appears to enhance the content creation because it better aligns with the general emotions of the audience, potentially leading to improved user feedback rates. The posts can then be made to evoke particular feelings, in the viewer.
The core algorithms of this content creation generator adjust through machine learning. This ongoing change in content, as a result of feedback, may cause an improved level of post efficiency. It indicates that it appears to grow with previous user interactions.
The generator is designed to tailor posts for multiple social media channels, all at once. Information gathered indicates that there has been a 30% lift in visibility when using this cross-platform approach.
Content saturation is potentially reduced through personalized content, tailored around user activity. The generator therefore minimizes the problems associated with this effect and can potentially better maintain user loyalty and satisfaction.
How an AI-Driven Social Media Strategy Generated $1M Revenue in 365 Days A Data-Backed Case Analysis - Real-Time Sentiment Analysis Drives 312% Increase in Customer Response Rate
The use of real-time sentiment analysis significantly improved customer response rates, with a reported 312% increase. By immediately processing and reacting to customer emotions, companies are able to interact in a more pertinent and timely manner. When considered as part of an AI-driven social media plan that generated one million dollars in revenue, these results underscore how critical sophisticated data analytics can be in marketing strategy. This live data analysis allows companies to develop stronger connections with customers, fundamentally changing how they approach their marketing initiatives to drive financial gains. This kind of dynamic engagement through sentiment tracking not only shows consumer opinions but also allows a swift reaction to shifts in the marketplace.
Real-time sentiment analysis reportedly led to a 312% increase in customer response rates. This number alone is striking, but the details underlying it warrant further consideration. This approach suggests that being able to rapidly analyze how customers feel can allow for much quicker engagement. There is also the point that such a dramatic increase likely isn't just a random outcome, but rather signals a clear link between identifying sentiments, even complex ones, and prompting customer responses. While a 312% increase is certainly worth highlighting, without a detailed analysis of the type of customer response, that metric alone doesn’t mean everything. Were they, for example, more favorable responses, more useful feedback, or just more responses of any kind, including potentially negative ones? Furthermore, we have to also wonder if there might be an increase of “cheap” responses—ones that may not be the type of conversion/customer that is most beneficial. It would be vital to know what the original baseline was for response rate and the mechanisms of that customer response as well. It is quite likely that these real-time analyses allowed for prompt handling of complaints, potentially preventing them from escalating into much bigger issues. A customer feels “seen” and the company, or brand, responds fast. This shows that a system like this, could drive up how satisfied a customer feels about a company. I am interested in understanding how these rapid insights are not only acted upon, but then what the effects of those responses had further downstream, such as for brand loyalty and longer term purchasing behaviour. This points to a likely need to see this result in context, beyond just a single number, and also as just one of the many factors driving the larger growth. Finally, we should question what “real time” even really means in practical terms, as even within real-time data processing, there will be slight variations in timing, that could still yield large variances in customer behaviour and reactions if this system was not robust enough. So the details underlying that percentage are where the real findings lie, not the number itself.
AI-powered Trademark Search and Review: Streamline Your Brand Protection Process with Confidence and Speed (Get started for free)
More Posts from aitrademarkreview.com: