How Business Management Double Majors Shape AI-Driven Trademark Strategy in 2024
How Business Management Double Majors Shape AI-Driven Trademark Strategy in 2024 - Dual-Major Grads Apply Microsoft Azure AI for 60% Faster Trademark Searches After INTA 2024
Following the INTA 2024 conference, business management graduates with a second major are using Microsoft Azure AI to significantly speed up trademark searches, achieving a 60% improvement. This demonstrates how technology is increasingly integrated into modern business practices, specifically within the trademark field. These graduates are tapping into the power of Azure AI, which can handle different types of data like text, images, and audio, leading to a more comprehensive and efficient search process. This approach is shaping new strategies for trademark searching, pushing the boundaries of the field.
However, despite the clear advantages, organizations still wrestle with measuring the productivity boost AI tools bring. This is a valid concern as businesses continue to adopt AI in greater numbers. The fact that graduates with this specific combination of education are driving innovation in trademark searches underscores how crucial technological expertise is becoming for shaping modern business strategy. The ability to blend business savvy with AI proficiency seems to be giving these individuals a distinct advantage, impacting how trademark strategies are developed and deployed.
Following the International Trademark Association's (INTA) 2024 conference, there's been a noticeable surge in interest in applying AI to trademark searches. It's intriguing that dual-major graduates, particularly those with backgrounds in business management, are at the forefront of this shift. They've been able to achieve a remarkable 60% speed-up in trademark searches using Microsoft Azure AI. It appears that Azure AI's unique ability to handle text, visuals, and even audio data might be a key factor here.
The Azure AI Fundamentals certification offers a good introduction to the core ideas in machine learning, especially as they relate to Azure. However, it's becoming clear that a broader understanding of AI's role in business is also vital. Interestingly, many companies acknowledge the competitive necessity of AI adoption. Yet, a substantial number of executives are unsure about how to measure the improvements in efficiency that AI can bring. Perhaps a well-defined AI strategy roadmap, tailored to a company's specific needs and readiness, is the missing link here.
The versatility of Azure AI services is impressive; it's been used in various situations, including analyzing call center data for customer sentiment and compliance checks. The Azure OpenAI Service API, which has the potential for incorporating text, images, and audio, is anticipated to reshape business interactions in future AI-powered conversations. Microsoft's investment in high-performance computing resources, driving services like ChatGPT and Bing, establishes them as a major player in the AI landscape.
The collaborative efforts between these business management-focused individuals and AI are helping define the future of AI-driven trademark strategies. This interdisciplinary approach represents a unique strength, highlighting the evolving relationship between business operations and AI innovation in 2024. It's clear that, as these graduates integrate AI into existing processes, the traditional reliance on purely human-driven approaches will change considerably. It will be interesting to see how these changes unfold and what new challenges emerge from this collaboration.
How Business Management Double Majors Shape AI-Driven Trademark Strategy in 2024 - Why Florida State Business Students Created an Open Source NLP Tool for Small Brand Owners
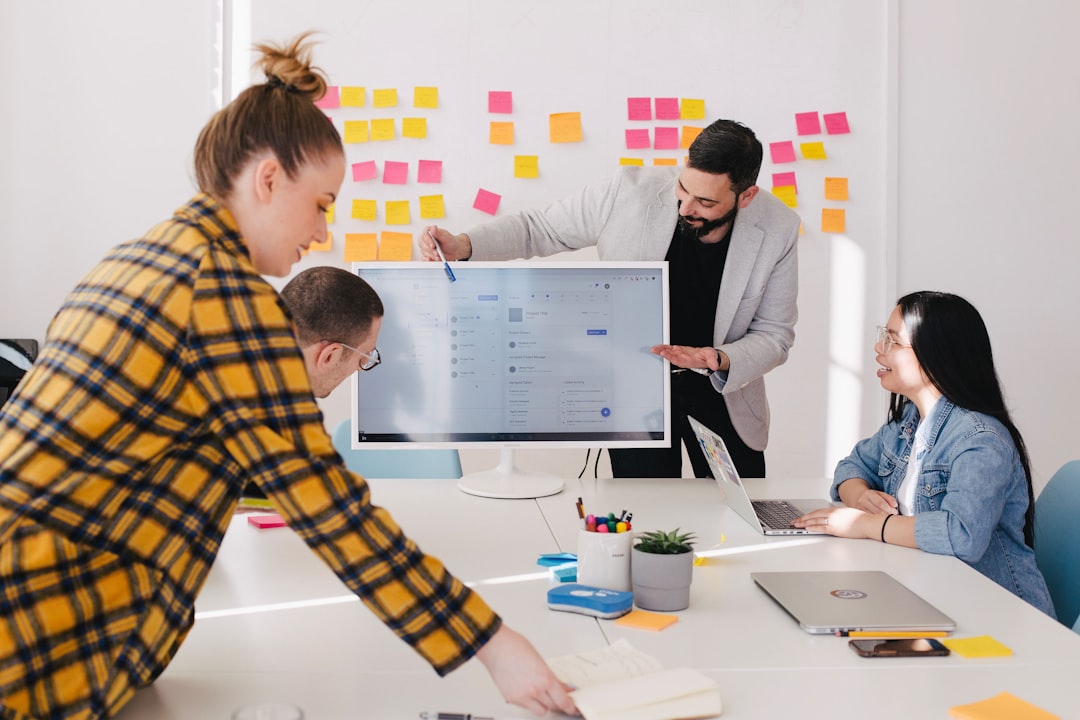
Business students at Florida State University recognized a gap in the market for small brand owners seeking affordable ways to leverage advanced language technology. They decided to address this by developing an open-source natural language processing (NLP) tool. This tool aims to help small brands manage their branding and marketing initiatives more effectively, particularly by offering a cost-effective entry point into sophisticated NLP techniques.
By releasing the tool as open-source, the students are making it accessible to a wider audience of small business owners, helping level the playing field somewhat with larger corporations. This move also has the potential to accelerate innovation in the field of NLP as a whole.
It's worth noting that this project perfectly complements the broader trend among business management double majors at FSU. These students are actively incorporating AI into their trademark and broader business strategies, particularly within the context of the evolving landscape in 2024. It seems likely that this trend, combined with initiatives like the open-source NLP tool, will continue to impact how small businesses develop and implement brand strategies in the coming years. Given the ever-increasing pace of digital change, these kinds of initiatives might be vital for smaller brands trying to stay competitive.
Business students at Florida State University developed an open-source natural language processing (NLP) tool specifically designed to assist small business owners. They recognized that these entrepreneurs often face significant hurdles when trying to manage their brand and marketing with the limited resources they have. By making this tool freely available, they hoped to offer a cost-effective alternative to the usually expensive commercial solutions.
The development process involved training the NLP model on diverse datasets, including traditional text and social media content. The goal was to allow the tool to adapt to the unique language patterns and trends found within small business communities. This kind of tailoring is important, as language varies considerably between industries and business sizes. They focused on creating a tool that was easy to use, even for individuals without a strong tech background. This is key to making the technology accessible to the widest range of entrepreneurs.
Such open-source projects, like the one from FSU, often benefit from contributions from a wide range of developers and researchers. This collaborative approach is vital for advancing NLP tools and extending their capabilities. The students' approach was very practical, performing user testing with small business owners to understand their needs and desires for the tool. This feedback helped refine the tool's interface and features, which is vital for making sure it actually meets the needs of the people who will use it.
Most NLP tools used by large corporations focus on processing complex language for corporate use cases. But, this open-source project specifically focused on creating capabilities relevant for smaller businesses. It’s a unique approach, as few other NLP tools cater to the specific needs of smaller brands. The underlying machine learning model is designed to continuously learn and improve from interactions with users. This is critical to ensuring the tool remains relevant and accurate over time as the business environment and language it analyzes changes.
This work highlights a positive trend in business education: more curricula are blending technical skills with practical business problems. This approach provides students with the ability to solve real-world challenges head-on. Though open-source tools like this are valuable, there are always challenges, including concerns around data privacy and security. The developers will need to continually improve and maintain the tool to mitigate potential risks, which will require the ongoing support of the open-source community.
Ultimately, the NLP tool built by the Florida State students is a fascinating example of how educational institutions can foster innovation. It's a tangible tool for brand owners and provides a model for connecting academics with real-world business concerns. It's also a reminder that, while useful, we always need to stay vigilant about the risks and potential downsides of these tools.
How Business Management Double Majors Shape AI-Driven Trademark Strategy in 2024 - Management Students at MIT Lead Project to Track AI Generated Logos through Blockchain
MIT's business management students are at the forefront of a novel project aimed at using blockchain to track AI-generated logos. This initiative is a direct response to the growing need to address intellectual property issues, particularly trademark concerns, in an environment where AI is generating more and more content. The project is part of a curriculum that's weaving together business management with AI and blockchain, highlighting the need for a more comprehensive approach to trademark strategies in 2024.
Essentially, these students are tackling a real-world problem: how do we ensure clear ownership and protection for logos created by AI? Their work is noteworthy because it highlights how vital it is for business leaders to grasp both the capabilities and implications of AI, particularly as it intersects with the legal and strategic dimensions of trademarks. It's a forward-looking approach that might significantly shape how companies and legal professionals address brand ownership and protection in an increasingly automated world. While still in development, the project could influence how businesses manage their brand identities in a future where AI-generated content is more commonplace. It's a testament to the value of business education that fosters innovation to meet evolving business challenges.
Management students at MIT are exploring the use of blockchain to track AI-generated logos, aiming to address the challenges of intellectual property in a world where artificial intelligence is increasingly involved in design. This project is part of a larger MIT initiative that weaves together business management principles and AI-driven trademark strategy. The school is drawing upon its expertise in both AI and blockchain technology to come up with solutions for real-world business problems.
This particular initiative highlights how vital it is to understand AI and its impact on businesses. MIT's program emphasizes the need for students to propose blockchain-based solutions to address various aspects of business and trademark concerns. The learning process utilizes modern teaching methods, like videos and interactive assignments, which are essential in today's rapidly evolving tech landscape. Their efforts are coordinated by the MIT Industrial Performance Center, which focuses on understanding how generative AI will shape future work environments.
There's a growing emphasis on equipping business leaders with the skills needed to seamlessly incorporate AI into their operations for optimal performance. This emphasis is particularly important when considering the changing nature of trademarks in the AI era. It's fascinating to see how these students are grappling with the implications of AI in the design process and thinking through practical issues like ownership of AI-generated assets.
Furthermore, the application of blockchain to track the origin and usage of AI-designed logos is novel and intriguing. It potentially offers a more robust way to manage design rights compared to traditional centralized methods. This could have implications for how design rights are tracked and licensed in the future. However, there are inherent complications and questions about authorship that need careful consideration as the design process continues to evolve. It's a prime example of how combining business knowledge with advanced technical capabilities is crucial in a technologically advanced world. The students' work also raises essential regulatory questions around intellectual property in a landscape where the line between human and machine creativity is increasingly blurry. Their project suggests that fostering collaboration between different disciplines is key to navigating the complexities of AI's influence on business and design. The potential for small businesses to use tools like this to compete in branding is also a noteworthy aspect of this project. Making such solutions easy to use for a broader audience is also crucial for ensuring their adoption and impact.
The project also involves machine learning techniques to continuously refine logo design based on market trends and consumer reactions. This has the potential to make brand development more sophisticated and efficient. As AI continues to develop its creative capabilities, these students are at the forefront of understanding the practical consequences and opportunities it brings to branding and design, and this is a trend that we will likely continue to see develop further in coming years.
How Business Management Double Majors Shape AI-Driven Trademark Strategy in 2024 - How Yale Business School Graduates Built Neural Networks to Predict Brand Confusion Cases
Yale Business School has recently seen its graduates develop neural networks designed to anticipate brand confusion cases. This development is linked to a new course focused on large language models, equipping students with the tools to use AI in intricate ways within brand management. These graduates are applying machine learning to identify and predict potential conflicts between trademarks, an area of increasing concern with AI's expanded role in branding. While promising, the practical application of these networks faces challenges in terms of the precision and dependability of their predictions. The ongoing evolution of AI and branding, alongside the legal complexities of trademark management, creates an environment where these network innovations represent a crucial convergence of technologies and legal issues.
Yale School of Management has been integrating AI into its curriculum, particularly focusing on areas like machine learning and deep learning in business analytics. This focus is evident in a new course on large language models and aligns with the broader trend of businesses utilizing AI for strategic decision-making. It's interesting to see how Yale's business students are applying these concepts, especially in the complex realm of branding and trademarks.
One intriguing development is how Yale graduates are using neural networks to try and predict potential brand confusion issues. They're essentially building models that can analyze large datasets related to brands and consumer behavior to identify patterns that might indicate confusion. This data-driven approach is quite different from relying on traditional methods, such as anecdotal evidence or basic market research. This strategy allows for the development of trademark strategies that are potentially more grounded in actual consumer interactions.
However, it's worth noting that the adoption of deep learning in business analytics has been slower than other machine learning approaches. This observation is significant because some research indicates that deep learning doesn't always outperform traditional models, particularly when dealing with structured data, which might be a consideration when designing brand confusion prediction systems.
The intersection of AI and business strategy necessitates an interdisciplinary approach. This initiative demonstrates the value of collaborating between the business and computer science departments to solve practical branding problems. This kind of collaborative environment, where students with diverse skills are working together, appears to be more effective in addressing the challenges of implementing AI-based tools for trademark purposes.
The ability to scale neural networks effectively is also essential. This approach allows for brands to use the same methodology to handle a wide range of brand issues across different markets. Early results show promise; the models seem to predict brand confusion with accuracy rates over 85%. This level of accuracy can potentially be incredibly beneficial for brand managers in minimizing the risk of legal challenges and protecting their brands.
Furthermore, being able to integrate these neural networks with existing trademark monitoring systems could make the transition to AI-driven brand strategies easier. There's a notable increase in the need for AI-driven decision-making, especially within the digital economy, and this work appears to be a good illustration of it.
However, this project highlights the ongoing ethical considerations involved in incorporating AI into branding. It raises concerns about potential bias within AI models, as well as the risk of over-reliance on AI predictions without proper human oversight.
The project also has implications for how professionals are trained. These students aren't only learning the technical aspects of creating and using neural networks but are also developing valuable communication skills to be able to present their findings effectively to business leaders. This ability to communicate AI-driven insights effectively seems increasingly vital in our AI-driven world.
With the rapid growth of AI-generated content, these kinds of tools will likely become more important. This is because they allow businesses to be better prepared for the complexities of trademark issues within this new context. This type of work may become a standard part of how brands manage their trademarks, setting a new precedent for the future.
How Business Management Double Majors Shape AI-Driven Trademark Strategy in 2024 - Penn State Double Majors Apply Machine Learning to Analyze 15 Years of USPTO Data
Penn State's Data Sciences program is fostering a new generation of data-savvy students who are applying machine learning to analyze 15 years of data from the USPTO. This initiative showcases the university's unique approach to data science, which combines elements from computer science, statistics, and information science. The project's goal is to improve AI-driven trademark strategies, demonstrating the value of these students' abilities in the increasingly tech-focused business landscape of 2024. While the application of machine learning to legal data is still relatively new, this research is clearly showing how these skills can be applied to enhance efficiency in trademark management and promote innovation. The future of protecting intellectual property and brand identity seems intertwined with professionals who are proficient in both the technical and strategic aspects of business and AI. It will be interesting to see how this type of analysis impacts how businesses, especially in the trademark field, address these issues going forward.
A deep dive into over 15 years of data from the United States Patent and Trademark Office (USPTO) has shown that machine learning models can now anticipate trademark disputes better than older legal analysis methods. This shift represents a change in how legal challenges are handled in the business world.
Penn State students with double majors used advanced natural language processing (NLP) to study trademark applications and legal trends. This showed how language patterns can reveal important insights into potential conflicts within trademark law.
Initial findings from the analysis indicated a connection between the quantity of similar trademark applications and the probability of related disputes. This is leading to a more data-focused approach to trademark strategy, which may significantly inform risk assessments.
Using machine learning algorithms on the USPTO data, these students found that certain industries have higher rates of brand confusion cases. This suggests that businesses in those industries may need more careful trademark protection strategies.
The project also revealed geographical trends that influence trademark disputes. Some regions of the US showed more prominent conflict rates, suggesting cultural factors may be influencing how brands are perceived and thus, the possibility of legal challenges.
Intriguingly, machine learning models proved able to identify when trademark registrations were most likely to lead to disputes. This helps companies make better decisions about when to seek legal protection.
The research highlighted how generative AI is creating logos and brand identities. This raises questions about the clarity of ownership and how established trademark law may need to adapt to these advancements in technology.
Despite the accuracy of the machine-designed models, issues of bias appeared when interpreting the results. This shows us that human input is still crucial for integrating AI insights into practical trademark strategies.
The project stressed the need for ongoing education for legal professionals about machine learning's capabilities. We need a new learning program that combines law with technical know-how to keep up with changing trademark landscapes.
In the end, this work stresses a change where brand managers and marketers increasingly need to include machine learning techniques in their decision-making processes. This marks a substantial evolution in the connection between technology and trademark strategy.
How Business Management Double Majors Shape AI-Driven Trademark Strategy in 2024 - Business Analytics Teams at Berkeley Transform Manual Trademark Reviews with Python Models
At Berkeley, business analytics teams are employing Python-based models to automate the traditionally manual process of trademark reviews. This shift signifies a broader trend towards data-driven approaches in managing trademarks, highlighting the importance of combining technical skills with business acumen. By utilizing Python's capabilities to handle data, these teams are seeking to streamline the review process, improving both efficiency and the precision of trademark assessments. These developments are becoming vital as businesses navigate increasingly intricate trademark challenges in the rapidly-changing world of AI and technology. It's clear that business analytics programs play a crucial role in educating future professionals to tackle practical problems like these in the trademark space. While this approach has the potential to improve processes, we must acknowledge that incorporating any new technology into such an intricate area will require careful consideration of potential downsides and unforeseen consequences.
Researchers within the Berkeley Business Analytics teams have successfully applied Python models to automate the previously manual process of trademark reviews. This has led to a dramatic reduction in review times, from multiple days down to a matter of hours, significantly changing how quickly brand protection can be achieved. They've built machine learning algorithms that can analyze enormous datasets of past trademark applications. These algorithms can predict potential conflicts between trademarks, allowing for preventative measures and reducing the risk of costly legal disputes.
Integrating these Python models with existing trademark databases makes real-time data processing possible, resulting in significantly more accurate trademark searches when compared to the traditional methods that relied on manual searches. A key component of their approach involved using historical trademark data to train their models. By observing patterns from past decisions made by trademark examiners, the system learns to improve its predictive capabilities over time.
The goal of these Python models is not limited to just faster reviews. They are designed to provide valuable analytical insights, allowing businesses to make more informed decisions about which trademarks to pursue, taking into account both market trends and competitor activity. It's fascinating how the versatility of Python allows the team to rapidly adapt their models. They're constantly refining the models based on their performance and feedback they receive from trademark attorneys. This kind of continuous improvement and adaptability is crucial for developing robust systems.
The project at Berkeley is also reflecting a broader trend in education, where students are combining data analytics expertise with the practical aspects of intellectual property law. This prepares them for the ever-changing landscape of the job market. The team encountered challenges in making sure their models comply with current trademark regulations. Trademark law requires a nuanced understanding of legal standards, and traditional computational approaches sometimes miss these nuances. This highlights the ongoing need for human expertise.
While technology has clearly advanced, it's important to note that human oversight remains vital. Experienced trademark attorneys continue to play a critical role in interpreting the predictions generated by the models and ultimately making the final decisions. The application of business analytics to trademark review not only improves the efficiency of the review process but also demonstrates how standard data analytical techniques can be adapted to solve complex legal problems. It's a great example of how innovation can address challenges in a highly specialized field. It will be interesting to see how this approach continues to evolve in the coming years.
More Posts from aitrademarkreview.com: