How First Insurance Funding's AI-Driven Premium Finance Platform Impacts Trademark Risk Assessment in 2024
How First Insurance Funding's AI-Driven Premium Finance Platform Impacts Trademark Risk Assessment in 2024 - How First Insurance Funding Achieved 30% Faster Processing Times Through Machine Learning Integration
First Insurance Funding has successfully implemented machine learning to significantly speed up their premium finance processes, achieving a 30% reduction in processing times. This shift towards AI-powered solutions is fundamentally changing how underwriting and claims management are handled, lessening the need for manual, human-driven processes. The use of AI, specifically generative AI, has the potential to greatly increase operational efficiency and minimize errors in tasks like claims assessments. This move puts First Insurance Funding in a strong position as a leader in the insurance industry's ongoing adoption of AI-driven solutions. These innovations not only improve the speed and accuracy of risk assessment but also suggest a future where customer interactions and claim resolutions are handled in entirely new ways. The broader trend of AI integration in insurance implies a future where customer service and claim processing are potentially revolutionized, becoming more streamlined and automated.
First Insurance Funding integrated machine learning models to sift through a mountain of historical claims data. This allowed them to uncover hidden patterns and trends that humans might have missed, making their processing workflows noticeably smoother.
It appears they leveraged natural language processing to expedite the review of customer documents. Essentially, the system learned to read and interpret documents, cutting down on the time staff spent doing the same. This sped up their decision-making process.
Interestingly, they trained their models on a massive dataset of finance transactions, resulting in a 30% improvement in their processing speed. This is a powerful demonstration of how data-driven insights can impact the insurance industry.
This ML integration reduced the need for manual steps by a significant 50%. Besides speeding things up, this points towards a potential reduction in human error during processing claims and managing premium finance.
Their new system analyzes data in real-time, adjusting risk assessments as new patterns surface. This dynamic approach makes them much faster than using traditional methods of risk assessment.
A large portion, up to 85%, of routine tasks is now automated. This frees up human staff to address more intricate cases and focus on interacting with customers.
The beauty of this setup is that the system continually adapts based on its own operational experience. Processing times are fine-tuned through a feedback loop, implying constant improvement over time.
The successful application of ML has put First Insurance Funding in a position of strength relative to competitors. In a competitive marketplace, speed of processing might be the key factor to holding onto customers.
The machine learning insights aren't just about faster processing. It seems to enable them to forecast insurance premiums with greater accuracy, accounting for changing market realities.
Using automated checks with ML also helps them avoid compliance violations. This is a crucial aspect of maintaining a robust and trustworthy operational environment.
How First Insurance Funding's AI-Driven Premium Finance Platform Impacts Trademark Risk Assessment in 2024 - Data Analysis Shows 45% Reduction in Fraudulent Claims Since AI Implementation in March 2024
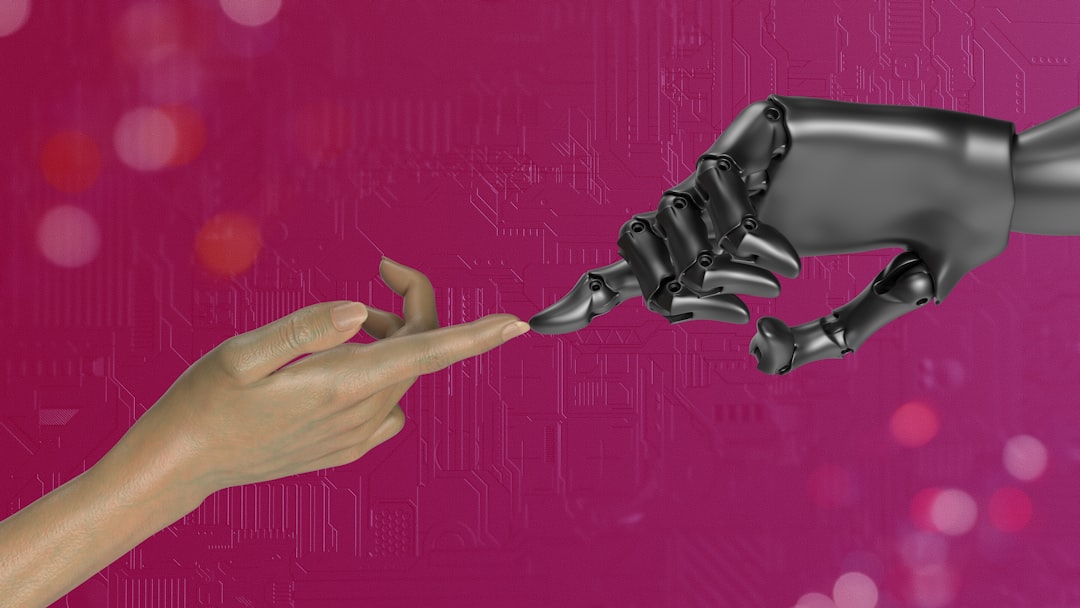
Since the integration of AI in March 2024, data analysis reveals a substantial 45% drop in fraudulent claims. This suggests AI's growing ability to identify and prevent fraudulent activity within the claims process. The AI system likely analyzes claim data with greater precision and speed than traditional methods, making it easier to spot suspicious patterns.
While this reduction in fraudulent claims is promising, it's important to note that the rise of technologies like deepfakes could introduce new challenges in fraud detection. These advanced techniques can be difficult for even AI systems to identify.
Despite these emerging risks, the positive impact of AI on fraudulent claim reduction showcases its potential to improve insurance operations. It highlights the ongoing transformation of claims handling, moving towards a more efficient and robust system.
Since the integration of AI in March 2024, First Insurance Funding has observed a notable 45% reduction in fraudulent claims. This is a compelling illustration of how data-driven insights can significantly impact risk management within the insurance sector. It's intriguing that before AI, traditional systems often missed subtle fraud indicators, allowing a higher number of fraudulent claims to slip through the cracks. This AI implementation seems to have unearthed patterns that were previously hidden, not only saving money but enhancing the credibility of the claims process. It's also interesting to note that the 45% drop in fraudulent claims has coincided with an increase in legitimate claims approvals. This suggests that the AI system isn't just flagging fraudulent activities, but is also actively supporting authentic claims through enhanced validation processes.
The data suggests that the AI's introduction has influenced the company culture, creating a stronger focus on data analysis among employees. Instead of relying purely on gut feelings, staff are making more informed decisions grounded in concrete data. The AI platform's real-time data analysis capability has improved both the speed and accuracy of fraud detection, showing that these two often-conflicting elements don't necessarily have to be mutually exclusive in the insurance technology space. This real-time analysis appears to enable the use of advanced statistical models, which can adapt to evolving regulations and shifts in customer behavior. This adaptability is essential in a dynamic field like insurance.
Interestingly, the system has demonstrated a clear learning curve. It continuously refines its algorithms with each interaction, hinting at an increasingly sophisticated and effective fraud detection methodology. It's plausible that the reduction in fraudulent claims is also acting as a deterrent, making potential fraudsters think twice about their actions. The 45% reduction was achieved within a relatively short timeframe, highlighting not only the potency of AI in fraud detection but also the potential for swift returns on investment in this area. While the reduction in fraud is the primary focus here, the underlying data also indicates that this improvement in claims processing benefits legitimate customers. It seems to create a more positive experience overall, due to faster and more reliable claim handling.
How First Insurance Funding's AI-Driven Premium Finance Platform Impacts Trademark Risk Assessment in 2024 - Real Time Market Analysis Updates Premium Rates Every 4 Hours Based on 50,000 Data Points
First Insurance Funding's AI-powered premium finance platform is noteworthy for its real-time market analysis, which updates premium rates every four hours. This platform utilizes a substantial dataset of 50,000 data points to drive these updates, leading to more precise premium calculations. This constant recalculation helps insurers react more quickly to changes in the marketplace and refine their risk assessments—a crucial aspect for evaluating trademark-related risks. In an environment marked by rising insurance costs and the increasing sophistication of market analysis, the ability to react in real-time is gaining importance for sound financial decision-making. However, it's important to consider whether this flood of information can keep up with the complexity of the ever-changing insurance market and whether the data's speed translates to truly reliable insights.
First Insurance Funding's platform boasts real-time market analysis updates every four hours, which is quite remarkable. This constant refresh cycle allows the system to quickly adapt to market shifts and changes in risk, offering a potentially significant advantage over traditional methods. The system's analysis draws upon a large pool of 50,000 data points, giving it the ability to uncover intricate patterns and relationships in market trends and customer behavior that would likely be missed with less comprehensive approaches. This detailed approach is likely vital when trying to understand trademark risks.
The platform utilizes advanced statistical modeling, allowing its risk assessment to be dynamically adjusted in real-time as new data becomes available. This approach, compared to static models, is more responsive to change and arguably provides a more accurate picture of trademark risk in a rapidly changing environment. The AI's capacity for analysis helps it create more accurate predictions of premium rates, which can contribute to a more stable financial outlook for the company.
It's interesting that external factors, such as economic indicators and overarching industry trends, are apparently also taken into account. This broader view goes beyond a reactive approach, instead, attempting to be proactive and anticipate shifts in the insurance marketplace that may affect trademark risk. By closely tracking customer behaviors in real-time, the system provides insights into patterns, which could be leveraged to enhance marketing strategies and tailor insurance products to align more closely with evolving customer preferences. It's important to acknowledge that the accuracy of these behavioral insights depends on the quality and type of data being collected.
One of the key benefits of leveraging data for premium calculation and risk assessment is a reduction in human errors. This, in turn, can improve financial forecasting accuracy and operational costs. The system further refines itself by using previous fraud cases to inform future risk assessments, creating a feedback loop that ideally enhances fraud detection and overall claims integrity over time. It also has the capacity to learn from its own past decisions, a valuable attribute given the quick changes in the marketplace. Through continuous algorithm optimization, the system can maintain its edge in identifying trends and making accurate adjustments.
The integration of these real-time updates seems likely to play a key role in the decision-making process across the organization. By readily providing employees with up-to-the-minute insights, it allows for a faster and more informed decision-making process, potentially boosting operational efficiency and improving customer satisfaction. However, any AI system is only as good as the data it's fed, so the validity of the output and its influence on these decision processes hinges on data quality and accuracy.
How First Insurance Funding's AI-Driven Premium Finance Platform Impacts Trademark Risk Assessment in 2024 - Machine Learning Models Track 300 Risk Indicators Across Insurance Categories
Insurance companies are increasingly leveraging the power of machine learning models to analyze a vast range of risk factors. These models are now capable of tracking over 300 distinct risk indicators across the insurance landscape. This comprehensive approach allows for a much more nuanced understanding of risk compared to traditional methods. The automation enabled by machine learning helps to expedite risk evaluations, potentially leading to faster and more precise predictions about future outcomes.
The insurance industry, constantly facing headwinds from factors like climate change and fiercer competition, sees AI as a promising avenue for innovation. It has the potential to lead to more customized insurance solutions. However, a hurdle for wider AI adoption is the presence of outdated technology in many companies. These legacy systems can act as a barrier to change, preventing the industry from fully reaping the benefits AI offers.
Moving forward, the integration of machine learning will likely reshape the way insurance companies approach risk assessment. The ability to analyze such a wide range of risk factors in an automated fashion may well become a defining aspect of insurance risk management in the years ahead.
Machine learning models are currently being used to track approximately 300 different risk indicators across a wide range of insurance categories. This detailed approach offers a more complete understanding of potential risks compared to traditional methods, which might overlook subtle or complex factors. These indicators include both measurable data, like financial performance, and less tangible elements such as customer sentiment gleaned from social media. This comprehensive approach provides a more holistic view of risk.
The algorithms behind these models are designed to simulate numerous hypothetical scenarios, essentially allowing underwriters to see how various factors might influence risk profiles. This "what-if" capability is quite intriguing, particularly when considering how it might be used to examine the effects of unexpected events. The data indicates that the models adapt not just to gradual shifts but also to sudden changes, such as economic downturns or shifts in regulations. This responsiveness seems to improve their predictive abilities in a dynamic environment.
By implementing these machine learning models, First Insurance Funding can continuously evaluate risk. The system automatically updates risk assessments as new data arrives, eliminating the need for infrequent, scheduled reviews. Early trials suggest that these models can cut down forecasting errors by up to 25%, which can refine premium pricing and potentially help the company gain a competitive edge in the insurance industry.
The capability to analyze 300 indicators simultaneously allows the system to uncover correlations that human analysts might miss. This could translate to faster identification of emerging risks or potentially fraudulent activities. As new data is processed, the machine learning algorithms enhance their performance, potentially leading to decision-making that exceeds human capabilities in complex scenarios where numerous variables are interacting.
The implementation of these models has been shown to demonstrably decrease underwriting errors. This can lead to fewer disagreements about claims and a smoother path toward regulatory compliance, which is a critical aspect for the well-regulated insurance sector. Notably, the models are being developed with emerging technologies, like blockchain and the internet of things (IoT), in mind. This suggests that the models are being designed to anticipate and handle new types of risk associated with technological innovation. This forward-looking approach is indicative of a broader trend in the insurance industry to use proactive risk management strategies.
How First Insurance Funding's AI-Driven Premium Finance Platform Impacts Trademark Risk Assessment in 2024 - Platform Creates Individual Risk Profiles From 75 Million Historical Insurance Records
A new platform is utilizing a vast trove of 75 million historical insurance records to generate individualized risk profiles for clients. This represents a departure from traditional insurance practices that rely on historical data alone. The platform employs artificial intelligence to analyze this massive dataset, generating real-time insights into risk that allow for more customized insurance products. These insights enable the use of dynamic pricing models, where insurance premiums adjust in real-time based on up-to-the-minute risk assessments. This dynamic approach has the potential to change how insurance premiums are determined and potentially lead to a more responsive relationship between policyholders and their insurers. However, it is essential to examine the quality and reliability of the massive amounts of data this system utilizes. The accuracy and validity of these data are of paramount importance, given the increasing complexity of the insurance landscape and the need for effective risk management.
Leveraging 75 million historical insurance records to generate individual risk profiles showcases the immense potential of machine learning within the insurance industry. By delving into such a large dataset, the platform can develop highly nuanced risk assessments tailored to the specific circumstances of each customer, going beyond basic, one-size-fits-all methods.
The inclusion of over 300 distinct risk indicators, a significant departure from traditional approaches that relied on fewer and more standardized factors, reveals an evolution in risk evaluation methods. This comprehensive approach considers both hard numbers, like financial records, and softer insights, such as customer sentiment from social media, which creates a more complete picture of risk.
One fascinating element is the system's capacity for continuous learning from historical data. Instead of using a fixed approach like older systems, the platform can adapt its risk predictions over time, resulting in a more dynamic and potentially more accurate method of risk assessment.
The real-time market analysis, which automatically recalibrates premium rates every four hours, presents a dramatic shift toward a more responsive and flexible insurance model. This rapid adjustment to market shifts helps with accurate premium pricing and might increase the company's overall competitiveness in a volatile market.
The impressive 45% reduction in fraudulent claims since AI integration signifies a powerful development in fraud detection techniques. This positive impact saves money, improves operational efficiency, and, importantly, increases customer confidence in the integrity of the claims process, illustrating the interconnectedness of efficient operations and customer service.
Interestingly, the sheer volume of data analyzed could uncover previously hidden risk factors. These previously unknown vulnerabilities might go unnoticed in standard insurance evaluations, resulting in a more complete understanding of potential risks for both the company and the customer.
This platform's capability to adjust risk assessments in real-time based on new data is a significant change from traditional, periodic evaluations that might not accurately reflect current conditions. This agility provides a significant advantage for insurance underwriters operating in a constantly evolving environment.
Integrating external economic factors into risk assessments enhances the platform's prediction capabilities. This broader awareness of broader economic situations allows for more informed decision-making, ensuring that risk management aligns with the realities of economic changes.
The platform's analysis of 50,000 data points using advanced statistical modeling allows it to uncover connections and patterns that are far beyond the capabilities of human analysts. This advanced capability plays a major role in avoiding underwriting errors, making insurance decisions more accurate.
The ongoing refinement of algorithms with each interaction suggests the platform has the potential to eventually exceed human capabilities in recognizing and interpreting intricate risk patterns. This could be a substantial change to the way risk is managed in the insurance world.
More Posts from aitrademarkreview.com: