AI-powered Trademark Search and Review: Streamline Your Brand Protection Process with Confidence and Speed (Get started for free)
Prelaunch Marketing Strategies 7 Data-Driven Approaches for AI Trademark Products
Prelaunch Marketing Strategies 7 Data-Driven Approaches for AI Trademark Products - AI-Powered Competitor Analysis for Trademark Products
In the prelaunch phase of a trademark product, understanding the competitive landscape is crucial. AI-powered competitor analysis offers a powerful approach to achieve this. These tools, using sophisticated algorithms, can provide real-time data about what competitors are doing and how the market is reacting. This can help businesses fine-tune their pricing, target customers more precisely, and ultimately, aim for a larger slice of the market.
AI excels at processing large volumes of information quickly. This allows for a rapid assessment of competitors' product lines, helping to identify industry benchmarks and inform strategy. Integrating these AI tools into existing marketing systems can streamline the process of using data to make decisions, demonstrating the potential of AI to reshape how we approach marketing strategy.
It's important to remember that AI is a tool. While it delivers valuable insights, human judgment is essential. Critically evaluating the output of AI, coupled with a thoughtful approach to strategy and outreach, is needed to maximize its effectiveness. Simply relying on AI without critical human analysis can lead to flawed interpretations and poorly informed decisions.
AI-powered competitor analysis is transforming the landscape of trademark product development by automating and enhancing the process of understanding the competitive environment. These tools can rapidly analyze vast quantities of trademark data, uncovering patterns and insights that would be practically impossible for humans to achieve in a reasonable timeframe. For example, AI can detect potential trademark infringements not just through exact matches but also by recognizing subtle similarities in design elements, potentially uncovering hidden conflicts.
Furthermore, AI can delve into the realm of consumer sentiment by analyzing publicly available information, providing a glimpse into how consumers perceive competitor brands and their products. This can be incredibly useful in fine-tuning a brand's positioning and messaging prior to launch. While traditionally this type of information gathering required painstaking manual research, AI can rapidly analyze vast amounts of online discussions, reviews, and social media content, delivering a comprehensive picture of the competitive landscape in a fraction of the time.
Another intriguing aspect of AI in this context is the ability to predict potential trademark disputes. By analyzing historical trends in litigation and trademark applications, machine learning models can identify potential conflict areas and help businesses plan accordingly. However, it's important to approach these predictions cautiously, as there's always an element of uncertainty involved. AI can also simulate different trademark strategies, essentially running "what-if" scenarios to see how changes in branding might affect market share and consumer perception. This allows companies to explore various options and understand the potential impact of their decisions in a virtual environment before making costly commitments.
The ability of AI to provide real-time updates on competitor activity is a huge advantage. Unlike traditional market research, which often operates on a delayed cycle, AI-powered solutions can adapt quickly to changes in the market, enabling businesses to respond promptly to evolving competitor strategies. While this is a significant benefit, it's essential to consider that the rapid pace of change also means that constant vigilance and data validation are necessary.
Additionally, AI can incorporate cultural and geographical context into its analysis, potentially highlighting nuances in consumer behavior that may be overlooked otherwise. Linking trademark strength to sales data is also a key advantage. AI solutions can leverage publicly available data to connect the performance of a trademark with actual sales metrics, allowing for a more tangible and quantifiable evaluation of trademark value.
However, it's crucial to acknowledge the limitations of AI. While it offers powerful capabilities, it is vital to exercise caution and human oversight. Algorithmic biases can skew results, so a healthy skepticism and careful validation of AI-derived insights are essential to ensure the accuracy and reliability of the analysis. This continuous interplay between human expertise and AI assistance is key to harnessing the full potential of AI-driven competitor analysis.
Prelaunch Marketing Strategies 7 Data-Driven Approaches for AI Trademark Products - Predictive Analytics for Consumer Demand Forecasting
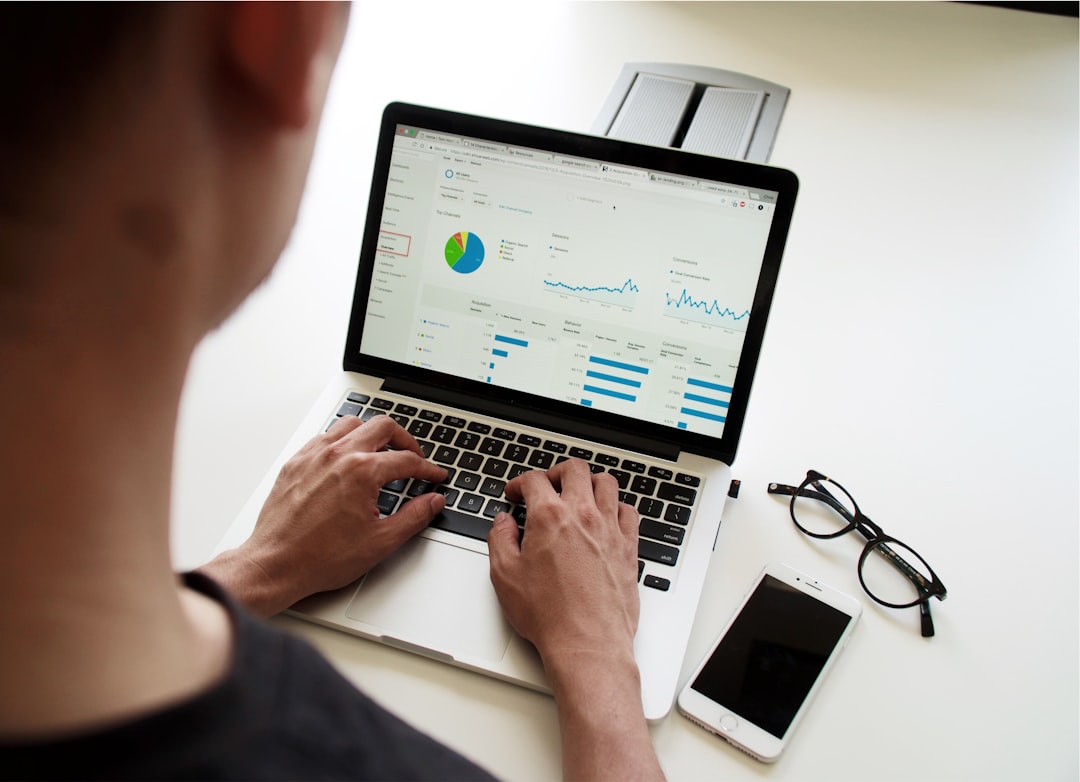
Predictive analytics is becoming a vital component in forecasting consumer demand. It leverages historical data, sophisticated algorithms, and machine learning to generate more precise forecasts compared to traditional methods. This approach helps marketers discern hidden patterns and trends within consumer behavior, providing a clearer picture of future demand. The ability to anticipate consumer actions, particularly in situations like the pandemic that highlight the unpredictable nature of markets, is a key advantage of this method.
The field of predictive analytics is experiencing a surge in interest and is expected to expand significantly in the coming years. This growth reflects a growing recognition among businesses that incorporating data-driven insights into demand forecasting can be beneficial. But, fully realizing the potential of predictive analytics demands a fundamental shift in mindset. Marketers need to actively challenge ingrained biases and develop a data-centric approach to forecasting, continuously refining their strategies based on the output of these sophisticated algorithms.
While the predictive power of AI is undeniable, it's important to exercise a healthy degree of skepticism. Human oversight is crucial in interpreting and validating the insights derived from these models. By combining the power of AI with careful human judgment, businesses can effectively harness the full potential of predictive analytics for more robust and accurate consumer demand forecasting.
Predictive analytics, with its blend of historical data, statistical methods, and machine learning, offers a more sophisticated approach to forecasting than relying solely on traditional, often simpler, methods. While the potential for improvement is significant, with some studies suggesting a 75% increase in accuracy, it's crucial to remember that proper implementation is key to unlocking these benefits.
The market for predictive analytics itself is expanding rapidly, projecting to grow from a substantial $14 billion in 2023 to a staggering $953 billion by 2032, reflecting the growing recognition of its value across various sectors. This rapid growth suggests a strong belief in the transformative capabilities of predictive methods within industries. Many marketing leaders already foresee the importance of AI-driven predictive analytics, with a recent Gartner study highlighting that a majority, 74%, of Chief Marketing Officers believe it will be fundamental for their marketing strategies in the coming years. It's interesting to see how this evolving understanding of AI's capabilities within marketing is reshaping traditional approaches.
However, successfully implementing predictive analytics requires a shift in mindset. It often involves a conscious effort by decision-makers to overcome ingrained biases and embrace the exploration of new, potentially unconventional data sources. This can be particularly challenging during times of uncertainty, such as the COVID-19 pandemic, when established patterns are disrupted and new consumer behaviours emerge.
Developing a robust predictive marketing strategy necessitates a structured approach that starts with clearly defined marketing goals. This framework forms the foundation for integrating the insights gleaned from data analytics and predictive models. Predictive analytics excels at uncovering subtle patterns and trends within vast datasets, which can lead to a better understanding of possible future outcomes and the probabilities associated with them.
This convergence of data science and artificial intelligence is revolutionizing marketing. The ability to extract actionable, data-driven insights is becoming increasingly vital for organizations looking to stay ahead of the curve. It's fascinating how this merging of disciplines is producing new capabilities within a field that is constantly adapting to technological advances.
Deep learning-based predictive models are particularly useful in the context of consumer demand forecasting. These models can handle enormous volumes of demand data, facilitating short, medium, and long-term predictions. This ability to forecast over diverse timeframes is quite powerful, and it is worth exploring how we can best leverage these tools within various market environments.
The implications of different predictive analytics approaches span a wide range of industries, with applications extending from energy demand prediction to optimizing complex logistical operations like automated warehouse management. This demonstrates the broad applicability and potential of predictive analytics to address diverse challenges within various sectors.
AI-driven predictive analytics, particularly those employing machine learning algorithms and natural language processing, is rapidly becoming more common in efforts to improve customer engagement strategies. It's captivating to consider the potential of combining these techniques to enhance communication and personalize experiences in a way that enhances customer satisfaction and loyalty. While still a relatively new frontier, these AI-powered approaches could lead to a fundamentally different way to design customer interactions.
Prelaunch Marketing Strategies 7 Data-Driven Approaches for AI Trademark Products - Social Media Sentiment Analysis for Brand Positioning
Understanding how people feel about your brand before a product launch is essential. Social media sentiment analysis offers a window into public opinion, allowing you to gauge how people perceive your brand, products, and even your competitors. Tools that track social media mentions, keywords, and conversations in real time can provide a sentiment score, a numerical representation of the overall emotional tone expressed. This helps marketers adjust strategies based on how people are feeling.
By analyzing the context and themes surrounding brand mentions, businesses can develop a deeper understanding of consumer sentiment, moving beyond simple positive or negative classifications. It's not just about what is being said, but how it's being said. This approach is vital for brands seeking to resonate with their target audience and foster a positive brand image.
However, it's important to approach social media sentiment analysis with a balanced perspective. While AI-powered tools offer efficiency and insights, the outputs must be interpreted with care. The underlying algorithms may contain biases that could lead to incorrect interpretations, making human oversight crucial for ensuring that the insights align with strategic goals. As technology progresses, effectively incorporating sentiment analysis into marketing strategies will become increasingly important for shaping brand perception and achieving prelaunch success.
In the realm of prelaunch marketing, understanding how people feel about a brand is becoming increasingly important. Sentiment analysis, using methods to gauge emotional responses from text, can provide a way to measure how people react to a brand on social media. This can be done by assigning a sentiment score to social media posts or comments, offering a quantitative way to track emotional reactions. Marketers can use this information to fine-tune campaigns based on the collective feelings they see expressed.
Tools for social listening are essential for this process. These tools allow marketers to keep an eye on brand mentions, keywords, hashtags, and the ongoing conversations related to their products in real-time. By paying attention to the tone of these interactions and the context in which they occur, we can get a pretty good understanding of the sentiment expressed towards a brand.
The process of sentiment analysis often involves several steps. First, data needs to be collected from relevant platforms – things like social media and review websites. This raw data, which consists of text in the form of comments and posts, is then converted into insights that are meaningful for the brand.
A huge amount of people are on social media. By Q3 of 2023, it was reported that 94% of internet users were active on social media each month. This means there's a massive potential audience whose opinions can be captured and analyzed using sentiment analysis.
AI is playing a larger role in sentiment analysis. It can provide insights into public perception on social media, and help inform brand decisions based on evidence rather than speculation.
When brands understand how people feel about them, they can improve their image and connect more deeply with their target audience. Social media analytics tools that are designed for sentiment analysis can provide deep insights into consumer feedback.
As technology keeps progressing, sentiment analysis is becoming more crucial in the formation of marketing strategies. It helps brands grasp and respond to public opinions about their brand, in effect, letting them know what people are thinking and feeling about them.
Tools that streamline the process are being developed. For example, Buffer offers features that allow sentiment to be tagged onto posts – categorizing them as negative, questions, or orders. This helps brands better manage responses and prioritize their efforts based on the type of interaction.
Sentiment analysis can influence marketing strategies quite a bit. Companies can assess how well their campaigns are performing by seeing the feedback on social media. This can lead to adjustments and optimizations to achieve better results.
The ability of sentiment analysis to generate actionable insights that enhance decision-making makes it very important, particularly when planning for product launches. This approach to decision-making, rooted in data and public sentiment, is becoming standard practice.
While this offers a potentially powerful method of informing marketing strategy, it is important to remember that this is still a relatively new area of study and the methods used have limitations. Further development and critical research are required to ensure that the approaches used in sentiment analysis are both reliable and accurate.
Prelaunch Marketing Strategies 7 Data-Driven Approaches for AI Trademark Products - Personalized Email Campaigns Using Machine Learning
Personalized email campaigns, powered by machine learning, are gaining traction as a key approach for prelaunch marketing. These campaigns allow businesses to craft emails that resonate with individual recipients by leveraging data on their past interactions and preferences. Machine learning enables sophisticated audience segmentation, which allows marketers to send more relevant content, resulting in higher engagement and potentially, more sales. Further, AI algorithms can predict how people might respond to messages, helping marketers optimize the timing and content of their campaigns. This involves creating dynamically generated email content tailored to specific users.
While the ability to personalize and optimize email campaigns is alluring, marketers need to be mindful of potential downsides. Over-reliance on technology can lead to overlooking human considerations in the design of campaigns. Machine learning algorithms are susceptible to biases, which could lead to unintended consequences and poor decisions. Maintaining human oversight in these processes, combined with a thorough understanding of ethical implications, is crucial for successful implementation.
As the use of AI in marketing continues to develop, personalized email campaigns will likely become a standard tactic for reaching potential customers before product launches. The ability to connect with individuals on a more personal level is a powerful aspect of marketing, yet this power must be used with a deep understanding of both the potential benefits and risks.
Personalized email marketing, powered by machine learning, is about tailoring messages to individuals based on their data and behavior. It's not surprising that a significant portion of businesses – around 74% – are using personalization, often starting with something simple like using the recipient's name. While this might seem basic, it underscores a core principle of connecting with people on a more individual level.
The application of AI in this field leverages machine learning algorithms to fine-tune various aspects of email marketing, from optimizing content to determining the best time to send emails and identifying the right groups to target. We're seeing a fascinating interplay of predictive and generative AI in email marketing. Predictive AI, using past data, can offer insights into future behavior, while generative AI takes this a step further by creating content specifically tailored to what individuals seem to be interested in. It's notable that a sizable proportion – about 39% – of marketing leads believe that using AI tools helps them make better, data-driven decisions.
Continuous improvement is key. With AI, we can constantly test and refine aspects of email campaigns like subject lines, content variations, and even the timing of emails. This constant optimization is enabled by AI's ability to analyze results quickly. Ultimately, the goal is to build stronger customer relationships and get people to interact more with the brand. It's about converting interest into action.
However, it's crucial to move beyond the simple tactics and leverage AI's capabilities for more sophisticated segmentation and analysis of data beyond basic demographics. Just knowing that someone is 35 years old might not be enough; understanding their interests and patterns can give us a more nuanced understanding of their preferences.
The integration of AI-driven tools is becoming increasingly vital for companies seeking to improve engagement and get better conversion rates. This means going beyond the basics and delving deeper into AI's potential. In fact, AI-driven email strategies are quickly evolving into the standard approach for reaching customers with impactful messages in the digital landscape. It's a trend that suggests that the future of effective communication will likely rely on AI's capabilities to personalize and optimize marketing efforts.
Prelaunch Marketing Strategies 7 Data-Driven Approaches for AI Trademark Products - Dynamic Pricing Strategies Based on Real-Time Market Data
Dynamic pricing, a method of adjusting prices based on real-time market conditions, is gaining traction as a way to optimize revenue. This approach uses sophisticated algorithms and machine learning to analyze factors like demand, supply, and competitor actions, allowing businesses to respond quickly to changes in the market. By dynamically adapting pricing, companies can potentially maximize profits and stay competitive in today's dynamic market landscape.
A key advantage of dynamic pricing is the ability to leverage diverse sources of information to optimize pricing decisions. Insights from sales teams, customer service representatives, and even market research can be integrated into a comprehensive pricing framework. This creates a feedback loop where pricing strategies can be continuously refined based on real-world observations. This shift from traditional static pricing models to dynamic ones necessitates a change in mindset, where data-driven insights guide price adjustments.
However, this evolving approach isn't without potential drawbacks. As dynamic pricing strategies become more prevalent, concerns about fairness and the perception of price gouging emerge. Finding a balance between optimizing revenue and ensuring that pricing remains fair and transparent to consumers is essential for fostering long-term customer trust. Furthermore, the complexity of dynamic pricing systems can require significant investment in technology and expertise, making it potentially inaccessible for smaller businesses.
In a rapidly changing market, the ability to adapt pricing strategies in real-time can provide a major competitive edge. By leveraging unified data models, companies can integrate information from diverse sources into a central hub, enabling more accurate and efficient price adjustments. Real-time analytics play a crucial role in identifying shifts in consumer behavior and competitor actions, providing a foundation for data-informed pricing decisions. But, this increased reliance on technology requires a vigilant approach to ensuring data accuracy and integrity, particularly in fast-paced markets. Overall, the implementation of dynamic pricing demands a careful balance between leveraging data-driven insights to maximize profits while ensuring the practice remains ethical and fosters a positive customer experience.
Dynamic pricing, where prices are adjusted in real-time based on market conditions, is an interesting approach to maximizing revenue. It uses the idea of price elasticity of demand, essentially figuring out how much the quantity of something people want changes when the price changes. This understanding helps create pricing models that aim to get the most money out of a situation depending on how responsive customers are at any point in time.
It's also notable that a sizable portion of businesses that use dynamic pricing are actively monitoring what their rivals are charging. This kind of real-time competitive analysis can be advantageous in staying competitive and maintaining profitability without sacrificing margins. Businesses aim to hold a good position in the market by adapting prices based on how competitors are behaving.
Researchers have also found that consumers appear to be willing to pay a bit more for goods if dynamic pricing is used carefully. It’s about understanding how people perceive value and making adjustments accordingly. This aspect of dynamic pricing relies on psychology, aiming to find prices that are likely to be accepted by consumers.
The use of machine learning is another key part of modern dynamic pricing. Advanced models can adjust prices thousands of times a day, taking into account many different factors, like changes in demand, supply, or even weather patterns. This capability enhances the accuracy of pricing.
We are seeing the rise of segmented pricing, where businesses adjust prices based on customer data like past purchases or browsing behavior. Dynamic pricing, in this way, can be highly personalized, potentially leading to higher conversion rates and greater customer loyalty.
The rate at which prices are altered using dynamic pricing can vary a lot, sometimes changing every few minutes, or a few hours. These changes can be particularly rapid in industries like travel and hospitality, where demand fluctuates frequently.
It's important to note how dynamic pricing can impact inventory management. Businesses can clear stock more quickly by adjusting prices based on demand, which lowers holding costs and reduces the chance of items becoming obsolete.
The use of dynamic pricing in subscription models is also gaining traction. Companies offering online services can use dynamic pricing to customize subscription fees based on individual usage and engagement. This approach can create a more satisfying experience for users and potentially increase the likelihood that they'll continue subscribing.
It's important to remember that dynamic pricing can have some ethical considerations. Some people may feel it's unfair if prices change quickly based on demand, particularly if it seems like businesses are taking advantage of consumers. This can affect how people perceive a brand, and so it's important for businesses to be mindful of this risk.
Studies have shown that businesses using dynamic pricing can improve profits by up to 25% compared to those with fixed pricing. This highlights how dynamic pricing can significantly change competition and potentially influence long-term financial success.
Prelaunch Marketing Strategies 7 Data-Driven Approaches for AI Trademark Products - Targeted Influencer Outreach Through AI-Driven Matching
AI-powered influencer matching is revolutionizing how brands connect with influencers for pre-launch marketing. These systems use algorithms to sift through huge amounts of data about influencers, such as their audience, content, and values. This allows brands to identify influencers that truly align with their brand image and target market. This method is a more efficient way to reach out to influencers, and it helps ensure partnerships are likely to be successful because the influencer's audience and the brand's audience are well-aligned.
Brands that use AI-driven influencer outreach may have an advantage in the evolving influencer marketing field, leading to more impactful collaborations and stronger connections with potential customers before a launch. However, it is important to keep human oversight as a key part of these processes. AI tools are helpful, but they are prone to biases, and these need to be carefully considered to avoid negative results or unintended consequences. This balance between AI support and human analysis is crucial for maximizing the benefits of AI-powered influencer outreach.
AI is increasingly shaping how brands connect with influencers, especially in the crucial prelaunch phase of a product. One fascinating aspect is using AI to match brands with influencers more effectively. By analyzing data like engagement rates, audience demographics, and content styles, AI can refine the search for influencers who are likely to be a good fit for a particular product or brand. This allows marketers to focus their efforts on influencers who have a high probability of generating a good return on their investment.
The real-time nature of AI is another compelling aspect of this approach. Market trends and consumer preferences are constantly shifting, and influencer performance can change rapidly. AI tools can process this data quickly, enabling brands to adjust their outreach tactics to stay current and relevant. In essence, it allows marketers to respond to market dynamics in a more agile manner.
Furthermore, AI can even try to anticipate how influencers might react to outreach. By studying their past collaborations and engagement patterns, machine learning algorithms can predict which influencers are more likely to be receptive to brand messages. This, in turn, leads to a more efficient and successful communication strategy.
AI also lets us map the influencer landscape in a way that was not possible before. We can see how various influencers are connected and identify potential opinion leaders who might otherwise be overlooked. This can broaden the reach of a brand and expose it to new audiences.
One of the more promising applications is the ability to work with micro-influencers. AI can identify these individuals, who tend to have smaller, but often highly engaged, followings. Since they often charge less than traditional celebrity influencers, this can significantly reduce marketing costs while potentially generating comparable results.
However, it is not just about finding influencers; it's about finding the right influencers. Integrating sentiment analysis tools helps to gauge how the public views specific influencers, which is critical in aligning with brand values before reaching out.
Another benefit is the ability to forecast campaign performance. AI can simulate various influencer partnerships and estimate how likely they are to be successful. This helps marketers make better decisions about who to work with before they invest time and resources in a campaign.
AI can also offer suggestions for optimizing how influencers present a brand. By analyzing historical data, it can suggest variations in message framing and presentation style, leading to more impactful campaigns.
Furthermore, AI can assist with managing ongoing relationships with influencers. By monitoring communication patterns and tracking engagement, it can suggest optimal times and approaches for follow-ups, ensuring that these partnerships remain strong and beneficial.
While AI promises to improve influencer outreach, it is crucial to acknowledge the potential for biases within the algorithms. AI models might favor certain demographic groups or engagement styles, leading to less diversity in influencer selection. This means continuous human oversight is essential to validate AI's suggestions and ensure a diverse representation of voices.
In conclusion, AI-powered influencer outreach holds considerable promise in refining prelaunch marketing strategies. Its ability to provide precise targeting, analyze real-time data, and optimize relationships can greatly improve the efficacy of influencer collaborations. Yet, like any tool, it must be applied judiciously, with human oversight playing a critical role in mitigating bias and achieving desired outcomes.
Prelaunch Marketing Strategies 7 Data-Driven Approaches for AI Trademark Products - Chatbot-Assisted Pre-Order Systems for Early Adopters
Chatbots offer a new way to manage pre-orders, particularly for those eager to be among the first to own an AI trademark product. These systems can make the pre-ordering process smoother, allowing companies to collect payments and learn more about what buyers want. Since many early adopters are already comfortable using AI in other areas, companies can build upon that familiarity to get their products in front of this key demographic. However, it's crucial that businesses avoid leaning too heavily on the automated nature of chatbots. They need to maintain a human element to ensure the interactions feel natural and meaningful. Finding the balance between automation and a personalized approach is important for developing strong customer relationships and ensuring a successful product launch.
Let's explore some interesting aspects of using chatbot-assisted pre-order systems, specifically for attracting early adopters of AI-powered products.
First, these systems can make the purchase decision process much quicker for customers. Research suggests that chatbots can speed up decision-making, which, in turn, can potentially lead to more sales. It's intriguing how a conversational interface can influence buying behaviors.
Another key feature is the continuous availability of these chatbots. Unlike human customer service teams, they can be available 24/7, addressing questions and guiding customers through the pre-order process regardless of the time or location. This constant availability could potentially translate into a better customer experience and, hopefully, more sales.
Furthermore, these systems are valuable for gathering data on how customers behave during pre-orders. Through analyzing this data, companies can start to understand what their customers want and tailor their marketing efforts more precisely. This type of data can also help refine inventory forecasting, leading to smoother operations later on.
It's also worth considering the cost implications. Utilizing chatbot technology can potentially reduce costs related to running customer service operations. Whether or not these cost savings translate into real benefits depends on factors like implementation and maintenance.
The integration of chatbots with social media platforms presents another fascinating angle. By integrating directly into platforms where users spend the majority of their time, companies can streamline the buying experience and also increase engagement with their brands. It's easy to see how this approach can lead to a smoother user journey.
One area of particular interest is how chatbots can personalize the pre-order experience. By leveraging user data, they can provide tailored recommendations, potentially leading to increased customer satisfaction. However, it's critical to be cautious with the use of personal data and to avoid overly intrusive interactions that can lead to a negative user experience.
Chatbots also offer a scalable solution for handling customer requests. They can manage a huge number of requests without sacrificing the quality of service. This scalability can be especially valuable during periods of high demand, ensuring that no customer is left waiting too long to make a purchase. However, it's worth noting that a very poor experience during a heavy load could generate bad publicity.
We also shouldn't overlook the possibility of using chatbots as a feedback loop for gathering customer input during pre-orders. This feedback can be instrumental in helping companies identify and fix potential issues before the product's official launch. This quick feedback mechanism is very advantageous.
One common challenge during online transactions is cart abandonment. Chatbots can help minimize this by providing support and answering questions for users who may be hesitating before completing their purchase. Data suggests that chatbots can reduce these abandonment rates, boosting sales overall.
Finally, it's worth highlighting the capability of AI chatbots to continuously learn and improve through user interactions. Over time, they become more effective at engaging with customers and uncovering valuable insights that could help automate and streamline the pre-order process. However, like other machine learning systems, they must be monitored closely to ensure their responses are appropriate and don't inadvertently perpetuate biases.
It's exciting to explore the potential of these chatbot-assisted pre-order systems and how they might change prelaunch marketing for AI-powered products in the coming months and years. It's important to use these systems wisely, ethically, and with a thorough understanding of their limitations. The goal, ultimately, is to build strong relationships with customers and generate positive prelaunch buzz for these innovative products.
AI-powered Trademark Search and Review: Streamline Your Brand Protection Process with Confidence and Speed (Get started for free)
More Posts from aitrademarkreview.com: