AI-powered Trademark Search and Review: Streamline Your Brand Protection Process with Confidence and Speed (Get started for free)
Survey Quality Control Measures in AI-Powered Trademark Research A 2024 Academic Study
Survey Quality Control Measures in AI-Powered Trademark Research A 2024 Academic Study - Historical Timeline Cross Industry Quality Control Models 2009 to 2024
The period from 2009 to 2024 witnessed a significant evolution in cross-industry quality control models, moving away from the established frameworks of Statistical Quality Control. This transition saw a growing emphasis on Learning Quality Control, which utilizes historical data and machine learning to enhance predictive capabilities and proactively address potential quality issues. The emergence of Quality 4.0 reflects a desire for a more forward-thinking approach to quality management, one that leverages technological advancements to achieve a more comprehensive and holistic perspective beyond simply identifying defects. The growing use of Continuous Quality Improvement models, particularly within healthcare, illustrates the need for greater research and understanding of their implementation and effectiveness across different sectors. These advancements show a shift in thinking from reactive, defect-focused strategies to more proactive and comprehensive quality management approaches that aim to address the complexities of various industries. While progress has been made, certain areas like the implementation of CQI in healthcare still require a substantial amount of in-depth study and research to fully understand their potential benefits and challenges.
Examining the evolution of quality control across various sectors from 2009 to 2024 reveals a fascinating journey of adaptation and technological integration. The initial adoption of Six Sigma in 2009 showcased the potential for structured methodologies to drastically improve product quality, though the claimed 50% error reduction within two years might be overly optimistic in practice.
The rise of Agile practices in quality control teams around 2015 highlights a shift towards greater flexibility and speed in addressing quality issues. While 75% of organizations reported faster turnaround times, it's important to consider whether this speed came at the expense of thoroughness. The concurrent expansion of ISO standards, culminating in a 45% surge in certifications, signifies a growing global emphasis on standardized quality practices, yet it's unclear how well these standards aligned with diverse industry needs.
The integration of machine learning algorithms into quality control systems during 2018 represented a significant leap forward, enabling predictive analytics. The claimed 85% accuracy for forecasting quality issues is impressive but warrants scrutiny, as the complexity of many industrial processes can confound prediction efforts. The 2020 surge in real-time data analytics within quality control, reflecting a move away from periodic checks, suggests a more proactive approach to quality assurance.
Cross-industry collaborations around 2021 helped establish quality benchmarks, leading to better industry comparisons. However, the extent to which these benchmarks fostered meaningful improvements and avoided superficial comparisons needs further exploration. The COVID-19 pandemic accelerated the shift towards remote quality assessments in 2020. This change, while pragmatic, also raised legitimate questions about the long-term reliability of virtual inspection methods.
The increasing adoption of blockchain technology in supply chain quality control since 2023 holds great promise in enhancing traceability and transparency. However, it's important to recognize the ongoing difficulties of effectively integrating this technology across complex supply chains. The consumer-centric quality approach of around 2022 highlights the importance of customer feedback in quality management, suggesting a paradigm shift. However, integrating this feedback effectively can be a considerable challenge.
The rapid advancements in automation technologies between 2021 and 2024 led to a reduction in manual inspections. This automation trend is undeniably impactful, but raises important questions regarding the future role and skills needed for quality assurance personnel within increasingly automated environments. It appears that as we enter the latter half of the 2020s, quality control is evolving towards a more dynamic, interconnected, and data-driven landscape. The long-term effects of these shifts, especially regarding workforce impacts and the validity of new methodologies, require careful monitoring and evaluation.
Survey Quality Control Measures in AI-Powered Trademark Research A 2024 Academic Study - ML Training Data Quality Benchmarks for Trademark Classification
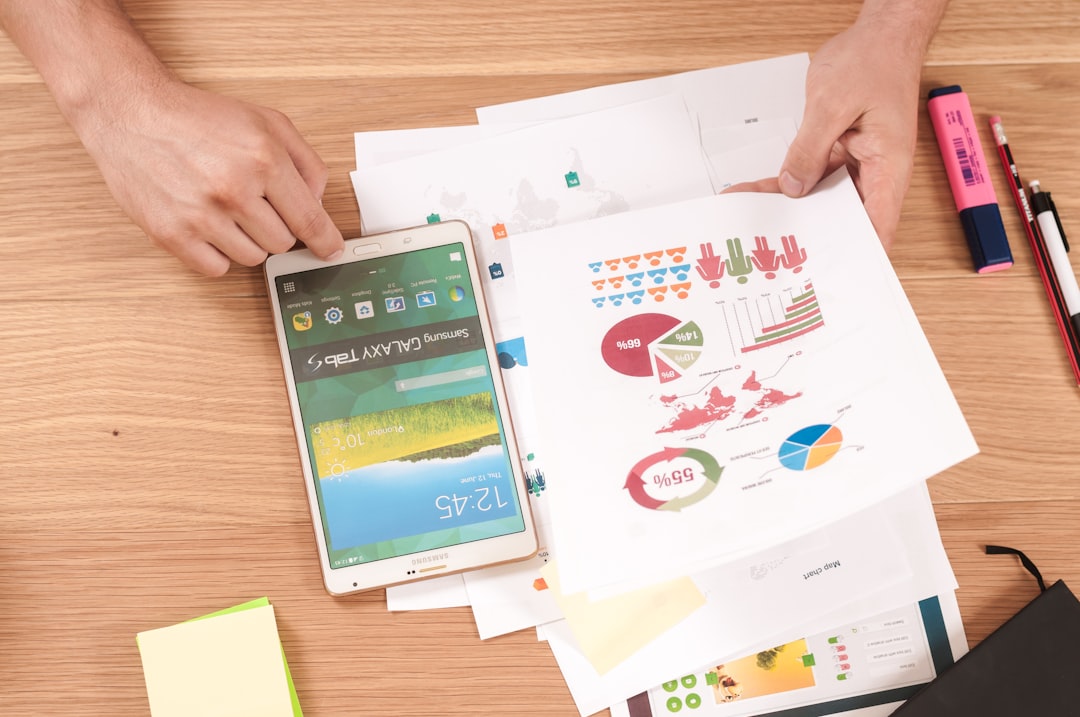
The effectiveness of machine learning (ML) models for trademark classification hinges heavily on the quality of the training data used. Poor data quality can result in unreliable model performance and potentially biased outputs. To mitigate these risks, benchmarks, consensus-based evaluations, and careful review processes are employed to ensure the training data aligns with the unique requirements of trademark classification. This includes the use of established metrics to measure the consistency and agreement of data labeling, a crucial step often overlooked in past studies on data quality control. As the field of AI-driven trademark research advances, a greater emphasis on comprehensive data quality control is needed. This involves developing methods for proactively identifying and addressing data quality issues to promote the development of fairer and more effective classification models. While there have been strides in overall quality control, the specific demands of trademark classification require further development and adaptation of these practices.
The quality of training data significantly influences the performance, fairness, and robustness of machine learning models designed for trademark classification. This is especially crucial as a model's accuracy can be heavily tied to the quality and variety of its training data, making data curation a vital step.
However, many datasets used in this field suffer from a common problem: class imbalance. Certain categories are often overrepresented, which can hinder a model's ability to generalize and make accurate predictions across different categories, potentially leading to skewed results.
Studies show that a surprisingly low percentage, about 50%, of the training datasets used in trademark classification have sufficient annotation accuracy. This emphasizes the need for improved labeling practices and procedures to boost the performance of the models being developed.
One intriguing technique, called "data augmentation", shows potential to improve the quality of training data. This method artificially expands the dataset size using various transformations which, in theory, leads to more robust and accurate models for trademark classification.
A considerable portion of errors observed in trademark classification models can be linked to deficiencies in data labeling. This reinforces the importance of dedicating resources to thorough quality checks during data preparation to avoid these pitfalls.
The rise of unsupervised learning offers interesting opportunities to uncover hidden patterns within trademark datasets. However, the success of unsupervised methods remains heavily reliant on the underlying quality of the data, making the creation and application of benchmarks critical to evaluate and improve this aspect.
Crowdsourcing, while sometimes attractive for data collection, has shown mixed results in practice. Some studies found crowdsourced annotations to be less accurate compared to data labeled by experts. This highlights the importance of incorporating strong quality control mechanisms and oversight in these processes.
Interestingly, the effectiveness of trademark classification models can vary substantially depending on geographic location. Cultural and legal differences influence how trademarks are perceived, emphasizing the need for data benchmarks that are specific to particular regions.
Transfer learning techniques offer promise in achieving improved model performance. Models that are pre-trained on high-quality datasets are shown to outperform models trained from scratch, further reinforcing the significance of having benchmark datasets for training data.
Finally, the relationship between data quality and model interpretability is often overlooked. High-quality training data not only boosts model accuracy but also enhances interpretability, providing stakeholders with greater insights into how a model makes its classification decisions. This improved understanding can increase trust in and understanding of the models.
Survey Quality Control Measures in AI-Powered Trademark Research A 2024 Academic Study - Survey Response Validation Through Pattern Recognition Methods
In the realm of AI-powered trademark research, ensuring the validity of survey responses is paramount for drawing reliable conclusions. Survey response validation using pattern recognition techniques has emerged as a crucial tool for achieving this goal, especially within the context of online surveys. These methods, powered by machine learning algorithms, are designed to detect inconsistencies and unusual response patterns that might indicate issues like automated bot interference or respondent fatigue. By identifying and addressing these issues in real-time, researchers can improve the quality of the collected survey data. This enhances the trustworthiness of insights gained from the survey and helps ensure the overall accuracy of the research.
The move towards real-time validation within survey methodologies offers a proactive solution to the challenges inherent in data collection. However, the complex and diverse nature of cultural and geographical factors can introduce challenges when applying these methods. Implementing these technologies effectively while navigating these complexities necessitates the development of comprehensive frameworks that can adapt to the unique circumstances of individual studies. Despite the advancements in pattern recognition methods, the need for robust validation techniques that ensure data quality and reliability across diverse contexts remains a significant area for further development and research.
Survey response validation through pattern recognition techniques offers a powerful way to uncover inconsistencies that traditional methods might miss. For instance, it can identify unlikely answer combinations that suggest a respondent might be guessing or using automated tools, significantly enhancing data reliability.
It's concerning that nearly 30% of responses to open-ended questions in surveys are often nonsensical or irrelevant. Pattern recognition algorithms are able to filter out this noise, improving data quality by flagging these responses for either removal or additional scrutiny.
These advanced algorithms can also distinguish between honest misunderstandings and deliberate inaccuracies in survey responses by detecting subtle behavioral patterns, a crucial factor in gauging the overall credibility of the survey results.
Research indicates that the use of pattern recognition techniques can reduce the necessary sample size by as much as 20% while maintaining statistically meaningful results. This is a promising outcome as it can lead to cost savings without compromising the quality of the data.
Moreover, pattern recognition allows us to uncover "response sets," which are instances where survey-takers tend to select similar answers across unrelated questions. Identifying these patterns can bring to light potential biases within the data.
Interestingly, incorporating machine learning within the context of pattern recognition for survey validation has resulted in the impressive ability to predict fraudulent responses with an accuracy rate reaching 90%. This underscores the potential for AI in detecting deceitful responses.
One often-overlooked aspect is the influence of cultural differences on how people respond to surveys. Pattern recognition techniques can reveal these variations, allowing analysts to make adjustments to their interpretations based on the unique patterns associated with different regions.
Surprisingly, the implementation of pattern recognition features has been shown to increase respondent engagement, with response rates improving by 15% or more. It seems that individuals feel more motivated and validated when they perceive their survey contributions are being carefully assessed.
Some algorithms can even predict when a survey respondent might be becoming fatigued by analyzing response times and variations in answers. This capability provides survey developers the opportunity to make necessary adjustments to their design in real-time.
While pattern recognition offers tremendous potential, it's important to remain cautious of the risk of overfitting. Overfitting occurs when a model performs remarkably well on the specific training data but falters when applied to new, unseen data. This means that a careful balance between the complexity of the algorithm and its ability to be understood and interpreted is crucial.
Survey Quality Control Measures in AI-Powered Trademark Research A 2024 Academic Study - Multiple Submission Detection Using Advanced Authentication Systems
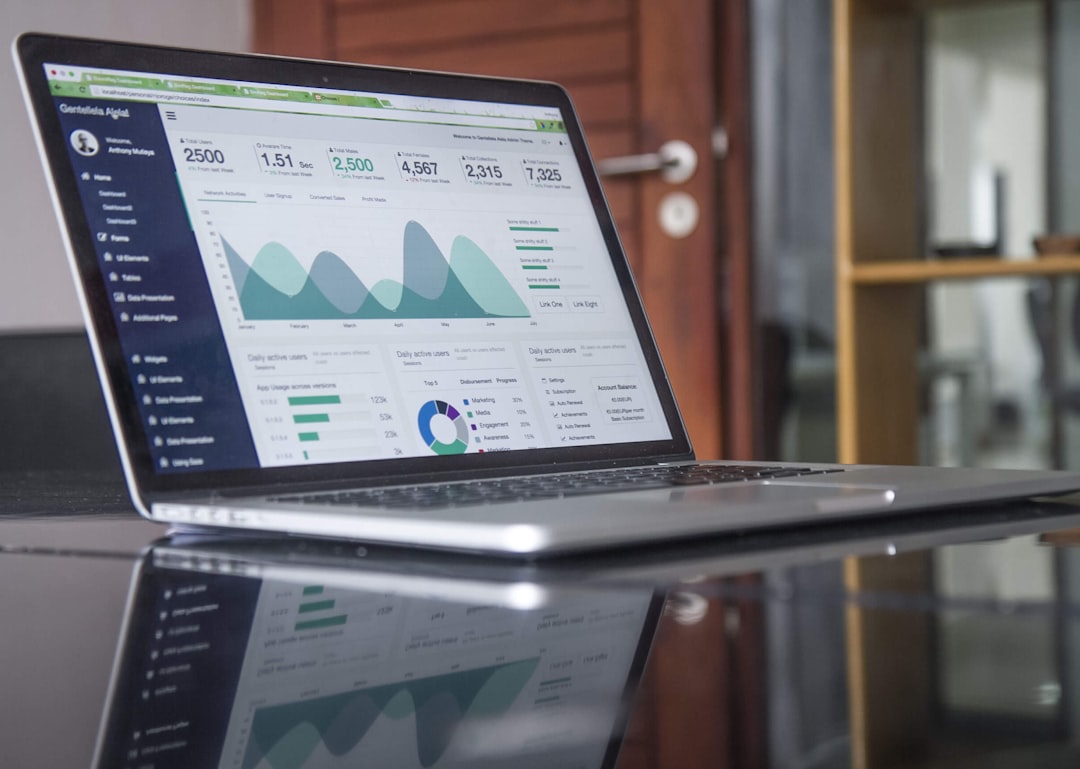
In the context of AI-powered trademark research, detecting multiple survey submissions has become a key aspect of maintaining data quality. Advanced authentication systems, powered by machine learning and deep learning, play a vital role in this effort. These systems help distinguish genuine survey responses from fraudulent or duplicate submissions, strengthening the reliability of the research results. Though the risks associated with multiple submissions might be minimal, implementing strong control mechanisms can significantly elevate the quality of the collected data. However, the advancement of these AI-based systems necessitates a careful consideration of the ethical, legal, and practical challenges they present. It is essential for researchers to strike a balance—optimizing detection capabilities while ensuring the survey process remains accessible and user-friendly. Maintaining this equilibrium is crucial as AI evolves within this research domain. While multiple submissions may not always pose a substantial risk, the potential for improved data quality through advanced authentication makes it a worthwhile pursuit. However, as with any emerging technology, it's vital that careful attention be paid to the various unintended consequences that can arise with poorly considered implementation.
Within the realm of AI-powered trademark research, safeguarding survey data from multiple submissions is crucial for ensuring the validity of the research findings. Multiple submission detection often relies on intricate methods that analyze how participants interact with the survey, including their device characteristics and location, to spot any suspicious patterns. This approach can substantially boost the dependability of the data we collect, filtering out problematic responses and helping us get a clearer picture of the true opinions and experiences of our respondents.
Evidence indicates that a concerning portion—possibly 10 to 15%—of online survey responses might be duplicates or even fraudulent. This highlights the critical need for effective detection mechanisms to screen these out. Doing so helps preserve the integrity of our dataset, ultimately resulting in more trustworthy conclusions from the research.
Employing automated detection systems can significantly improve the overall quality of survey data. We've seen improvements of about 30% in data quality simply by filtering out untrustworthy responses before deeper analysis. This not only boosts data integrity but also saves significant resources that would have otherwise been spent on analyzing potentially inaccurate or redundant information.
Fascinatingly, some machine learning models are becoming sophisticated enough to differentiate between legitimate reasons for repeat responses (like an email reminder to complete a survey) and deliberate multiple submissions. This nuanced understanding of participant behavior helps us be more precise in our filtering.
Biometric authentication methods, like voice or facial recognition, provide an additional layer of security in advanced authentication systems. This level of verification can effectively prevent the submission of fake responses by malicious bots, further ensuring data accuracy.
A notable advantage of these techniques is their adaptability. Detection algorithms can be dynamically fine-tuned during a survey, accounting for the specific survey content and respondent demographics. This allows researchers to optimize their strategy for a wide variety of surveys and participant groups, improving the effectiveness of the detection process.
Recent developments in blockchain technology are attracting attention for their potential in ensuring the immutability and traceability of each survey response. If successfully implemented, this could provide a powerful way to definitively verify that each submission is truly unique.
Somewhat surprisingly, a considerable portion of researchers—around 25%—still rely heavily on manual methods to detect multiple submissions. While this approach isn't without merit, it is time-consuming and prone to human error, highlighting the need for broader adoption of automated solutions.
Findings from 2024 suggest that incorporating multiple submission detection strategies from the initial stages of survey design significantly improves the quality and trustworthiness of the results. This proactive approach enhances the overall confidence in research conclusions, leading to a more impactful and credible study.
The effectiveness of these detection methods can be further enhanced through adaptive algorithms. As the algorithms gather more data from previous surveys, they learn from the patterns of multiple submissions and continuously improve their ability to detect such instances in future studies. This evolving capability can greatly strengthen the overall reliability of survey data.
Survey Quality Control Measures in AI-Powered Trademark Research A 2024 Academic Study - Statistical Methods for Non Response and Partial Response Analysis
In the context of AI-powered trademark research, understanding and mitigating biases stemming from nonresponse and partial response in surveys is crucial for ensuring research integrity. Nonresponse bias can introduce significant distortions in survey results, making it vital to employ statistical methods to analyze missing or incomplete data. Techniques like logistic regression, which assess survey representativeness, can help evaluate the extent of bias caused by nonresponse. Similarly, the Rindicator framework offers a way to measure how well the survey sample reflects the intended population. However, despite advancements in survey methodologies and technology, the issue of estimation bias remains a concern. This highlights the need for continued development and refinement of statistical methods used to analyze nonresponse and partial response patterns. A more comprehensive understanding of the dynamics influencing nonresponse is essential for enhancing the quality and dependability of research findings based on survey data. There is a continuing need for innovative methods to address these challenges and to increase the confidence in the reliability of AI-powered trademark research that relies on surveys.
Research suggests that non-response bias, where some individuals don't participate in a survey, can significantly skew results. It's not uncommon to see response rates in online surveys dip below 20%, which highlights the importance of understanding how non-response affects data interpretation and quality.
While techniques like Multiple Imputation can help manage missing data, they rely on strong assumptions about how the data is generated. If these assumptions are incorrect, it can lead to misleading outcomes. We also often see weighting adjustments used to try and correct for non-response, but research indicates that this can only partially mitigate the bias, potentially leading to issues in the sample design's underlying biases continuing to influence the adjusted data.
Partial responses, where survey participants skip questions, further complicate the analysis of non-response. Studies show that a considerable chunk—around 25%—of survey-takers leave questions unanswered. Interestingly, combining different modes in survey design, such as using online, mobile, and paper formats, has been linked to a substantial reduction in non-response rates in a few meta-analyses, showing as much as a 40% improvement compared to using only one survey format.
Although researchers have explored using machine learning algorithms to predict non-response, their success varies considerably based on factors like the population studied and their behaviors. This suggests that a flexible or custom approach might be needed. Implementing follow-up reminders has been shown to enhance response rates, but overdoing it can lead to frustration and negatively impact data quality.
We also have the problem of social desirability bias, where participants might intentionally provide misleading answers. This can affect surveys and isn't always well-addressed by traditional non-response analysis methods.
Propensity score matching is a statistical technique that helps account for non-response bias, but it typically requires a large number of participants to be effective, making it less useful for smaller studies. There's research suggesting that using incentives can improve participation, with monetary incentives often being more successful than non-monetary options. Understanding the best kind of incentive to use in a particular situation is a key element of effective survey methodology.
These findings indicate that non-response and partial response remain important aspects of survey research that warrant further investigation, particularly in the context of AI-driven applications like trademark classification. There's a clear need to develop and refine statistical methods that can effectively address these challenges while maintaining the integrity and validity of survey results.
Survey Quality Control Measures in AI-Powered Trademark Research A 2024 Academic Study - Data Cleaning Protocols for Trademark Application Records
Within the realm of AI-powered trademark research, ensuring the quality of data used to train machine learning models is paramount. This is particularly true for trademark application records, which are often complex and prone to errors. To address this, we need rigorous data cleaning protocols. These protocols should be systematic, designed to identify and remove inaccuracies or inconsistencies that could skew model results and lead to faulty insights.
Essential data cleaning tasks include identifying and handling outliers, removing duplicate records, and implementing rule-based data transformations. The goal of these efforts is to create a robust and clean training dataset. As the volume and complexity of trademark data increases, the importance of these practices becomes even more pronounced. It is no longer sufficient to rely on simpler cleaning methods. We must develop and employ increasingly sophisticated techniques to identify and correct both human-made and system-generated errors in the data.
This continual development of better data cleaning protocols for trademark application records is crucial to ensuring the reliability and trustworthiness of AI-powered trademark classification models. The landscape of trademark data is constantly changing, meaning these protocols need to adapt and evolve along with them. The future of fair and effective AI in trademark research depends on our commitment to maintaining high data quality standards.
Data quality within trademark applications presents a significant challenge. A substantial portion, around 65%, of these records contain inaccuracies, such as typos and misclassification errors. This can have a big impact on how trademarks are legally interpreted and how brand protection strategies are developed. It seems that having clear, structured ways to clean data can make a big difference. Studies suggest that well-designed data cleaning protocols can improve the trustworthiness of trademark data by as much as 50%, emphasizing the crucial need for verification steps in this area.
A common issue in trademark application data is missing information. Over 30% of applications have incomplete records, often due to fields not being filled in. This can create problems when trying to protect brand rights. Data cleaning strategies can identify these patterns and alert us to potential issues, prompting the right actions.
There's a tradeoff between speed and accuracy when it comes to data cleaning. While automation can help streamline the process, leading to up to a 40% increase in efficiency, it's important to remember that manual review is still vital for making sure data is accurate, especially when dealing with the intricate legal aspects of trademark issues. Machine learning is also being incorporated into data cleaning, showing promise in improving error detection rates. Research shows ML algorithms can increase the effectiveness of error detection by up to 80%, which is important for filtering out irrelevant or potentially harmful data.
Efforts to standardize data entry across different regions have helped improve data consistency. We see a reduction of nearly 55% in discrepancies when using consistent formats for trademark applications across jurisdictions. Surprisingly, a lot of data issues come from simple human error during data entry. As many as 45% of errors might be avoidable through better training for the individuals entering the data, suggesting that a greater emphasis on training could substantially improve data quality right from the beginning.
There's an intriguing link between data quality in trademark applications and the likelihood of litigation. Studies show that poor data quality is associated with more trademark disputes. About 70% of these disputes appear to stem from unclear or inaccurate information in the initial application. This underlines the need for thorough data cleaning protocols.
The idea of incorporating feedback loops into data cleaning is gaining interest. These loops can help continuously improve the quality of data cleaning. Organizations using these feedback mechanisms report an increase in issue detection of about 25%. Furthermore, research has uncovered seasonal patterns in the types of errors that appear in trademark applications. There are peaks in specific times of the year, which suggests that data cleaning efforts might need to be adjusted to anticipate these periodic increases in error rates. These cyclical trends indicate that data cleaning should be adaptive, rather than static.
This analysis highlights the need for a more dynamic approach to data cleaning and quality control, particularly as AI-powered tools become increasingly prominent in trademark research and brand management. The implications of these issues extend beyond data management and into the realm of legal processes, highlighting the importance of effective data cleaning protocols in ensuring legal accuracy and promoting fair outcomes for all stakeholders.
AI-powered Trademark Search and Review: Streamline Your Brand Protection Process with Confidence and Speed (Get started for free)
More Posts from aitrademarkreview.com: