Trademark Search Automation in 2025 7 Key AI Tools Revolutionizing USPTO Database Analysis
Trademark Search Automation in 2025 7 Key AI Tools Revolutionizing USPTO Database Analysis - ThorSearch By Accenture Reduces USPTO Database Analysis Time From 3 Days to 4 Hours
A notable development concerning the analysis of USPTO data involves a tool called ThorSearch, reportedly developed by Accenture. What's being highlighted is its claimed ability to significantly accelerate the time needed for database analysis in trademark searches, stating a reduction from a process that could take three days down to around four hours. If this level of efficiency is achievable, it marks a substantial change in the speed at which information can be processed when examining trademark applications.
Reports circulating as of May 2025 highlight a tool, ThorSearch, purportedly developed by Accenture, and its significant impact on USPTO database analysis time. Claims suggest this automation can compress a process that previously took around three days into roughly four hours.
From an engineering standpoint, achieving such a speedup implies substantial technical underpinnings. The system reportedly leverages advanced natural language processing to interpret complex search requests more effectively than traditional keyword methods. Furthermore, machine learning algorithms are cited for their ability to uncover potential conflicts that might escape human review or simpler software, going beyond obvious matches. The capacity to process vast volumes of data concurrently, handling millions of records rapidly, appears critical to this performance boost, far exceeding what's feasible with manual checks or less capable systems. The idea of the tool 'learning' from past searches and adapting to the evolving trademark landscape is intriguing; one wonders how robust this adaptation is against novel or abstract marks.
Beyond raw processing power, the design purportedly focuses on usability, offering a more intuitive interface and seamless integration into existing workflows. Its reported ability to handle international searches and offer predictive analytics for potential disputes suggests a broader scope than just basic matching, aiming for a more comprehensive risk assessment. Being cloud-based also offers practical accessibility.
While the reported efficiency gains from tools like ThorSearch are compelling, the real-world performance consistency and the methods for validating the accuracy of its 'hidden conflict' detection are key areas for scrutiny. Nevertheless, if these capabilities hold up under diverse and complex scenarios, such automation represents a notable stride in tackling the data analysis challenge inherent in large trademark databases like the USPTO's, potentially shifting the bottleneck elsewhere in the review process.
Trademark Search Automation in 2025 7 Key AI Tools Revolutionizing USPTO Database Analysis - Clarifai AutoMark Tool Now Identifies 98% of Similar Logos in USPTO Archives
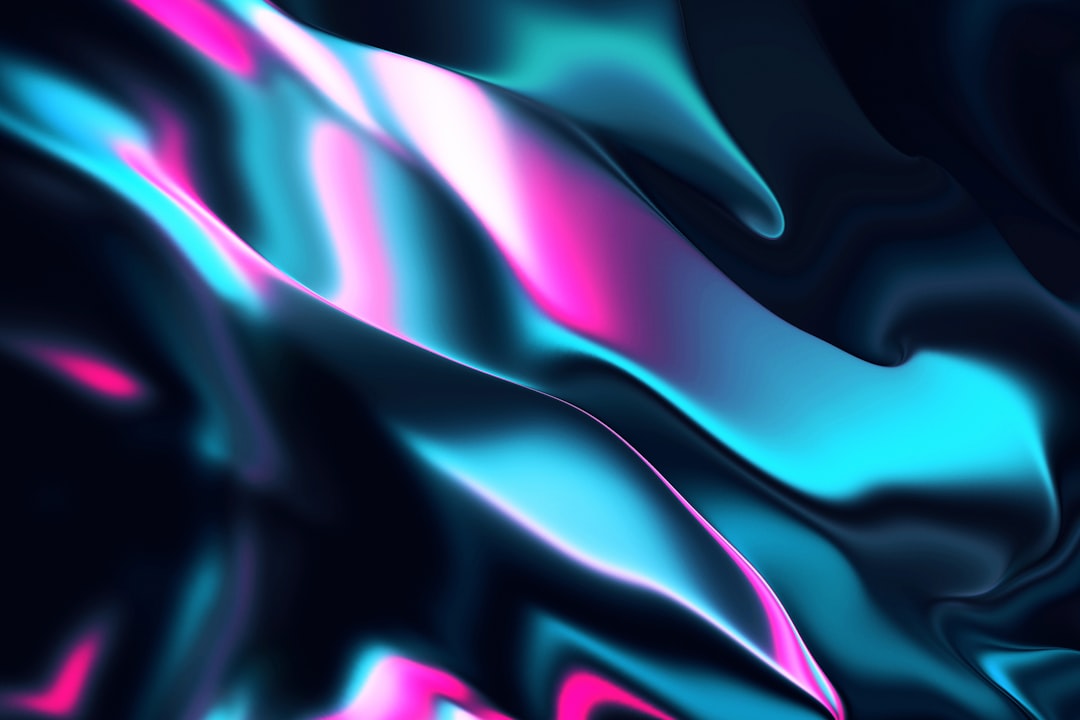
Reports highlight a development concerning the Clarifai AutoMark tool, specifically its claimed ability to identify similar logos within the USPTO archives with a reported 98% accuracy. This advancement is being noted as a component of the broader push towards automating trademark searches, potentially offering improvements in efficiency when examining visual marks. Utilizing artificial intelligence, the tool focuses on analyzing and comparing logo designs, aiming to streamline the process of finding potential conflicts in the extensive database. While a 98% accuracy figure sounds significant, the remaining percentage represents potential omissions, and the inherent challenge lies in how effectively an algorithm interprets the nuanced and sometimes subjective concept of visual similarity, which can be crucial in trademark law. Nevertheless, tools like this are contributing to the evolving landscape of how trademark databases are being analyzed through AI in 2025.
The Clarifai AutoMark tool is being discussed for its reported capacity to identify 98% of similar logos within the United States Patent and Trademark Office (USPTO) archives. From an engineering perspective, achieving this level of precision in visual recognition across a vast, dynamic dataset like the USPTO's represents a notable technical milestone, suggesting the deployment of sophisticated deep learning architectures, likely involving advanced convolutional neural networks (CNNs) tailored for logo feature extraction and comparison. Navigating the sheer volume of existing marks is a significant challenge, and the tool's ability to process millions of image records efficiently indicates a robust underlying system optimized for large-scale visual database analysis.
The reported real-time functionality, allowing examiners to potentially receive immediate feedback on submitted logo designs, could dramatically alter workflow efficiency in the visual examination process. The design approach seems to prioritize user accessibility, aiming to integrate these complex visual analyses into examiner workflows without demanding deep technical expertise. However, the effectiveness of such models heavily relies on the training data; a critical question remains how well the tool adapts to truly novel or abstract logo designs that deviate significantly from established visual patterns, and how its 'similarity' criteria align with evolving legal interpretations.
Looking beyond traditional logo matching, the underlying visual recognition capabilities potentially extend to other design elements in trademark applications, such as packaging or graphical components within advertising materials, hinting at a broader applicability for comprehensive visual intellectual property analysis. The integration of such a tool within the broader ecosystem of AI-driven search and analysis platforms presents interesting system design challenges and opportunities, moving towards interconnected tools rather than isolated functions. The reported high accuracy of automated visual assessment inevitably raises important legal questions about what constitutes 'similarity' in the context of potential infringement when determined by an algorithm, potentially pushing the boundaries of traditional legal definitions and the roles human examiners play in the subjective assessment of visual conflicts. As these automated tools become more prevalent, the future composition of trademark examination teams may need to evolve, potentially focusing human expertise on overseeing the AI outputs, handling edge cases, and navigating the complex legal and ethical implications that algorithms cannot yet fully address.
Trademark Search Automation in 2025 7 Key AI Tools Revolutionizing USPTO Database Analysis - Google Patents Integration With USPTO API Enables Real-Time Trademark Monitoring
The connection established between Google Patents and the USPTO's public API is being discussed as a notable step for keeping watch on trademarks in near real-time. This link aims to make the process of searching for potential trademark conflicts more streamlined. Having this hookup potentially provides users with more current access to trademark information directly from the database. The idea is that this facilitates easier tracking of modifications and new entries in the register. When combined with AI-driven search tools, automating trademark analysis could significantly alter how professionals manage their tasks. This applies to those in legal practice, various companies, and academic study. The goal is quicker assessments across extensive sets of information, though questions about accuracy and interpretation always remain with automated systems. As automated approaches to trademark searching continue to develop, this integration point could become an important element in evolving intellectual property monitoring practices.
The connection point between systems like Google Patents and the USPTO's API presents an intriguing technical avenue for trademark monitoring. Conceptually, this integration means data flows more readily, enabling a kind of continuous observation of the trademark landscape rather than relying on less frequent checks. The idea is that this real-time access allows for a more dynamic understanding of what's happening in the USPTO database, potentially enabling automated systems to flag new filings or changes in status very quickly. From an engineering standpoint, managing and analyzing this live stream of data, encompassing not just text but increasingly image-based marks due to improved API data provision, offers fresh challenges and opportunities for detecting potential conflicts and identifying groups of related marks ('clusters'). Furthermore, the possibility of cross-referencing trademark data with patent information through such integrated access could uncover previously obscure connections between technical innovations and brand identities.
While the aspiration for greater efficiency through this real-time capability is clear, relying heavily on automated systems fed by APIs necessitates careful consideration. The algorithms processing this continuous data stream must be robust, and as machine learning is applied to refine detection, the potential for embedding biases from historical data into future analysis is a non-trivial concern. How accurately these systems, regardless of their processing speed or data feed frequency, interpret the often nuanced concept of trademark similarity remains a critical question. Such automation shifts the technical challenge towards algorithm validation and interpretation, and it inevitably brings up discussions within the legal framework about automated decisions and the continued indispensable role of human judgment in evaluating complex cases and maintaining quality control over the output of these interconnected systems.
Trademark Search Automation in 2025 7 Key AI Tools Revolutionizing USPTO Database Analysis - Microsoft Azure Custom Vision Models Detect Fraudulent Trademark Applications
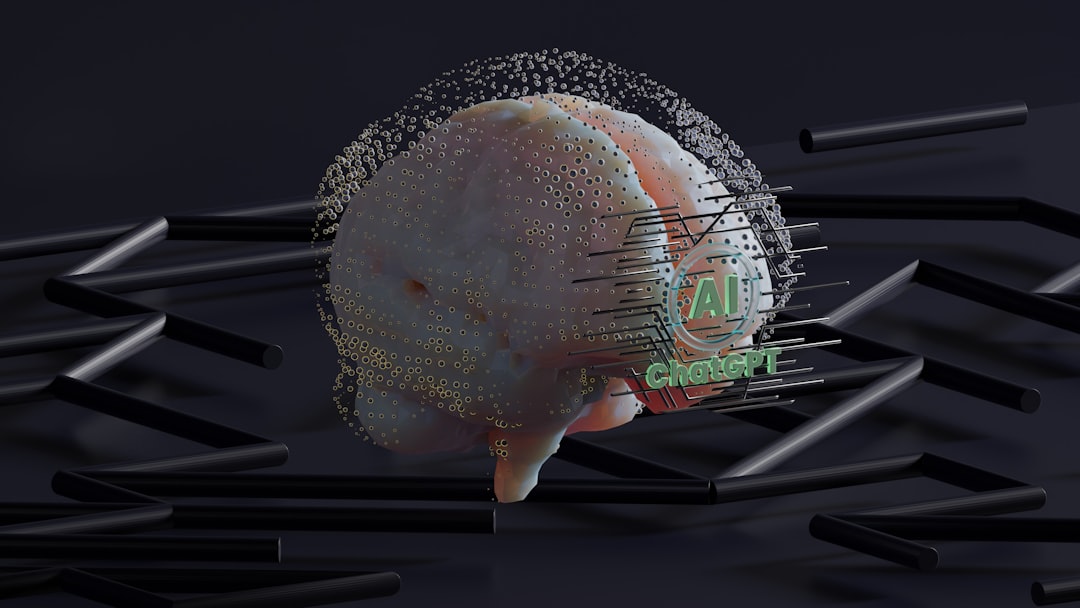
As of May 2025, tools such as Microsoft Azure's Custom Vision service are being applied to the visual analysis aspects of trademark applications. This capability allows users to develop specific models designed to interpret images, learning from provided sets of examples to either categorize visual elements or identify particular features within designs. The aim here is to use these trained models to help automate the process of examining logos and other visual marks for characteristics that might suggest an application is fraudulent or potentially conflicting. The service facilitates ongoing adjustments to these models, enabling them to adapt over time as new visual patterns emerge, potentially improving their effectiveness in identifying questionable submissions. However, automating the assessment of visual similarity, which is often a subjective and legally nuanced determination, poses inherent difficulties. While an AI can analyze based on learned patterns, capturing the full complexity of how visual marks are perceived by the public, critical for trademark evaluation, is a significant challenge. Therefore, while this technology offers assistance in filtering and highlighting visual points of concern, human expertise remains indispensable for making the final judgment calls in the context of trademark law and practice.
Examining the toolkit landscape for trademark analysis, Microsoft Azure’s Custom Vision service is presented as a framework for building tailored image recognition models, finding application in vetting trademark filings. The premise is to leverage this capability to identify visual cues within applications that might correlate with potentially fraudulent submissions. From an engineering standpoint, the approach involves training models on specific datasets encompassing examples of past filings, ostensibly including those deemed problematic. The goal is for the system to learn visual patterns associated with attempts to bypass examination criteria, potentially through deceptive similarities or misrepresentations in graphic elements. The service allows for continuous retraining, which is necessary given the evolving nature of filing strategies and potential deceptive tactics; one would need rigorous processes for curating the data used for this ongoing model improvement to prevent bias creep or overfitting to historical noise.
The models focus heavily on the visual components of a trademark application. While earlier sections discussed high-level logo matching accuracy, here the idea is to train specifically for patterns associated with malfeasance – perhaps subtle alterations, specific image manipulation techniques, or compositional elements designed to obscure similarities to existing marks while appearing distinct at first glance. The claim of reduced false positives is a technical objective critical for any screening system; the real test lies in the model's precision on novel or cleverly disguised fraudulent attempts. Scalability, via the cloud platform, addresses the practical necessity of handling large volumes of applications, but the core challenge remains the model's ability to accurately discern intent or legal nuance solely from visual data. Integrating these visual models with text analysis or other data streams, while feasible on the platform, introduces system complexity – how are conflicting signals weighted or resolved? Ultimately, while such custom visual analysis models can serve as automated filters to flag anomalies based on learned patterns, the complex, subjective, and legally charged nature of 'fraud' or 'deceptive similarity' means human oversight is not merely desirable but fundamentally necessary for responsible deployment in official processes. The usability of the interface for examiners without deep AI expertise becomes a key factor in the practical adoption and effective utilization of the system's outputs.
Trademark Search Automation in 2025 7 Key AI Tools Revolutionizing USPTO Database Analysis - TrademarkNow Platform Links 47 Global IP Databases For Comprehensive Search Results
The TrademarkNow platform has consolidated 47 intellectual property databases from around the world, intending to provide extensive trademark search results across different jurisdictions. The aim here is to facilitate access to a wide range of global trademark data more efficiently. Leveraging artificial intelligence capabilities, the system automates certain elements of the search process, reportedly allowing users to obtain results swiftly and with relative ease of use. As the total count of active trademarks globally continues to grow, approaching or exceeding one hundred million, systems that can help manage the sheer volume and complexity of this data are increasingly relevant for those involved in intellectual property matters. However, the effectiveness of integrating and analysing data from such a large and diverse set of global databases and translating automated findings into legally sound conclusions still presents challenges, necessitating careful review by human experts.
Examining platforms designed for automated trademark analysis, one such system highlights its approach by reportedly integrating information from 47 global intellectual property databases. From an engineering perspective, assembling and querying such a diverse collection presents a significant technical hurdle. Different jurisdictions employ varying data formats, classification systems, and update schedules, requiring robust data pipelines to normalize and process millions of records consistently. The claim of delivering comprehensive search results quickly, perhaps in seconds, implies highly efficient indexing and retrieval mechanisms operating across this distributed and varied dataset. The platform reportedly leverages advanced algorithms, often AI-driven, to identify potential conflicts; moving beyond simple keyword matching to recognize patterns, conceptual similarities, or phonetic nuances across multiple languages inherent in a global scope adds layers of algorithmic complexity. While the focus is on enhancing search thoroughness and speed, ensuring the quality and consistency of results drawn from so many distinct sources, each with its own potential for data lag or inconsistency, is an ongoing validation challenge for such a system. The design aims to make this intricate process accessible through a user interface, allowing for parameter customization despite the underlying data complexity. Tools like this underscore the technical demands of navigating the sheer scale and international nature of the modern trademark landscape.
More Posts from aitrademarkreview.com: